Jun 14, 2025
Abstract:This paper explores the growing presence of emotionally responsive artificial intelligence through a critical and interdisciplinary lens. Bringing together the voices of early-career researchers from multiple fields, it explores how AI systems that simulate or interpret human emotions are reshaping our interactions in areas such as education, healthcare, mental health, caregiving, and digital life. The analysis is structured around four central themes: the ethical implications of emotional AI, the cultural dynamics of human-machine interaction, the risks and opportunities for vulnerable populations, and the emerging regulatory, design, and technical considerations. The authors highlight the potential of affective AI to support mental well-being, enhance learning, and reduce loneliness, as well as the risks of emotional manipulation, over-reliance, misrepresentation, and cultural bias. Key challenges include simulating empathy without genuine understanding, encoding dominant sociocultural norms into AI systems, and insufficient safeguards for individuals in sensitive or high-risk contexts. Special attention is given to children, elderly users, and individuals with mental health challenges, who may interact with AI in emotionally significant ways. However, there remains a lack of cognitive or legal protections which are necessary to navigate such engagements safely. The report concludes with ten recommendations, including the need for transparency, certification frameworks, region-specific fine-tuning, human oversight, and longitudinal research. A curated supplementary section provides practical tools, models, and datasets to support further work in this domain.
* From the Spring School 2025 by AI Grid and SCAI (Sorbonne
University), 16 pages
Via
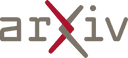