What is Object Detection? Object detection is a computer vision task in which the goal is to detect and locate objects of interest in an image or video. The task involves identifying the position and boundaries of objects in an image, and classifying the objects into different categories. It forms a crucial part of vision recognition, alongside image classification and retrieval.
Papers and Code
Jun 25, 2025
Abstract:Obtaining a better knowledge of the current state and behavior of objects orbiting Earth has proven to be essential for a range of applications such as active debris removal, in-orbit maintenance, or anomaly detection. 3D models represent a valuable source of information in the field of Space Situational Awareness (SSA). In this work, we leveraged Neural Radiance Fields (NeRF) to perform 3D reconstruction of non-cooperative space objects from simulated images. This scenario is challenging for NeRF models due to unusual camera characteristics and environmental conditions : mono-chromatic images, unknown object orientation, limited viewing angles, absence of diffuse lighting etc. In this work we focus primarly on the joint optimization of camera poses alongside the NeRF. Our experimental results show that the most accurate 3D reconstruction is achieved when training with successive images one-by-one. We estimate camera poses by optimizing an uniform rotation and use regularization to prevent successive poses from being too far apart.
* accepted for CVPR 2025 NFBCC workshop
Via
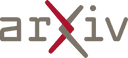
Jun 26, 2025
Abstract:Machine learning-assisted diagnosis is gaining traction in skin disease detection, but training effective models requires large amounts of high-quality data. Skin disease datasets often suffer from class imbalance, privacy concerns, and object bias, making data augmentation essential. While classical generative models are widely used, they demand extensive computational resources and lengthy training time. Quantum computing offers a promising alternative, but existing quantum-based image generation methods can only yield grayscale low-quality images. Through a novel classical-quantum latent space fusion technique, our work overcomes this limitation and introduces the first classical-quantum generative adversarial network (GAN) capable of generating color medical images. Our model outperforms classical deep convolutional GANs and existing hybrid classical-quantum GANs in both image generation quality and classification performance boost when used as data augmentation. Moreover, the performance boost is comparable with that achieved using state-of-the-art classical generative models, yet with over 25 times fewer parameters and 10 times fewer training epochs. Such results suggest a promising future for quantum image generation as quantum hardware advances. Finally, we demonstrate the robust performance of our model on real IBM quantum machine with hardware noise.
Via
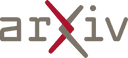
Jun 16, 2025
Abstract:Leveraging the high temporal resolution and dynamic range, object detection with event cameras can enhance the performance and safety of automotive and robotics applications in real-world scenarios. However, processing sparse event data requires compute-intensive convolutional recurrent units, complicating their integration into resource-constrained edge applications. Here, we propose the Sparse Event-based Efficient Detector (SEED) for efficient event-based object detection on neuromorphic processors. We introduce sparse convolutional recurrent learning, which achieves over 92% activation sparsity in recurrent processing, vastly reducing the cost for spatiotemporal reasoning on sparse event data. We validated our method on Prophesee's 1 Mpx and Gen1 event-based object detection datasets. Notably, SEED sets a new benchmark in computational efficiency for event-based object detection which requires long-term temporal learning. Compared to state-of-the-art methods, SEED significantly reduces synaptic operations while delivering higher or same-level mAP. Our hardware simulations showcase the critical role of SEED's hardware-aware design in achieving energy-efficient and low-latency neuromorphic processing.
* Accepted by IJCNN 2025
Via
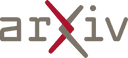
Jun 17, 2025
Abstract:In general, background subtraction-based methods are used to detect moving objects in visual tracking applications. In this paper, we employed a background subtraction-based scheme to detect the temporarily stationary objects. We proposed two schemes for stationary object detection, and we compare those in terms of detection performance and computational complexity. In the first approach, we used a single background, and in the second approach, we used dual backgrounds, generated with different learning rates, in order to detect temporarily stopped objects. Finally, we used normalized cross correlation (NCC) based image comparison to monitor and track the detected stationary object in a video scene. The proposed method is robust with partial occlusion, short-time fully occlusion, and illumination changes, and it can operate in real time.
* Smart Media Journal 1 (2012) 48-55
* 8 pages, 6 figures
Via
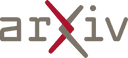
Jun 16, 2025
Abstract:Event cameras are gaining traction in traffic monitoring applications due to their low latency, high temporal resolution, and energy efficiency, which makes them well-suited for real-time object detection at traffic intersections. However, the development of robust event-based detection models is hindered by the limited availability of annotated real-world datasets. To address this, several simulation tools have been developed to generate synthetic event data. Among these, the CARLA driving simulator includes a built-in dynamic vision sensor (DVS) module that emulates event camera output. Despite its potential, the sim-to-real gap for event-based object detection remains insufficiently studied. In this work, we present a systematic evaluation of this gap by training a recurrent vision transformer model exclusively on synthetic data generated using CARLAs DVS and testing it on varying combinations of synthetic and real-world event streams. Our experiments show that models trained solely on synthetic data perform well on synthetic-heavy test sets but suffer significant performance degradation as the proportion of real-world data increases. In contrast, models trained on real-world data demonstrate stronger generalization across domains. This study offers the first quantifiable analysis of the sim-to-real gap in event-based object detection using CARLAs DVS. Our findings highlight limitations in current DVS simulation fidelity and underscore the need for improved domain adaptation techniques in neuromorphic vision for traffic monitoring.
Via
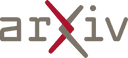
Jun 18, 2025
Abstract:This paper presents a generalization of the trajectory general optimal sub-pattern assignment (GOSPA) metric for evaluating multi-object tracking algorithms that provide trajectory estimates with track-level uncertainties. This metric builds on the recently introduced probabilistic GOSPA metric to account for both the existence and state estimation uncertainties of individual object states. Similar to trajectory GOSPA (TGOSPA), it can be formulated as a multidimensional assignment problem, and its linear programming relaxation--also a valid metric--is computable in polynomial time. Additionally, this metric retains the interpretability of TGOSPA, and we show that its decomposition yields intuitive costs terms associated to expected localization error and existence probability mismatch error for properly detected objects, expected missed and false detection error, and track switch error. The effectiveness of the proposed metric is demonstrated through a simulation study.
* 7 pages, 4 figures
Via
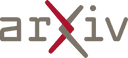
Jun 23, 2025
Abstract:Handling delicate and fragile objects remains a major challenge for robotic manipulation, especially for rigid parallel grippers. While the simplicity and versatility of parallel grippers have led to widespread adoption, these grippers are limited by their heavy reliance on visual feedback. Tactile sensing and soft robotics can add responsiveness and compliance. However, existing methods typically involve high integration complexity or suffer from slow response times. In this work, we introduce FORTE, a tactile sensing system embedded in compliant gripper fingers. FORTE uses 3D-printed fin-ray grippers with internal air channels to provide low-latency force and slip feedback. FORTE applies just enough force to grasp objects without damaging them, while remaining easy to fabricate and integrate. We find that FORTE can accurately estimate grasping forces from 0-8 N with an average error of 0.2 N, and detect slip events within 100 ms of occurring. We demonstrate FORTE's ability to grasp a wide range of slippery, fragile, and deformable objects. In particular, FORTE grasps fragile objects like raspberries and potato chips with a 98.6% success rate, and achieves 93% accuracy in detecting slip events. These results highlight FORTE's potential as a robust and practical solution for enabling delicate robotic manipulation. Project page: https://merge-lab.github.io/FORTE
Via
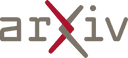
Jun 16, 2025
Abstract:We introduce the Lecture Video Visual Objects (LVVO) dataset, a new benchmark for visual object detection in educational video content. The dataset consists of 4,000 frames extracted from 245 lecture videos spanning biology, computer science, and geosciences. A subset of 1,000 frames, referred to as LVVO_1k, has been manually annotated with bounding boxes for four visual categories: Table, Chart-Graph, Photographic-image, and Visual-illustration. Each frame was labeled independently by two annotators, resulting in an inter-annotator F1 score of 83.41%, indicating strong agreement. To ensure high-quality consensus annotations, a third expert reviewed and resolved all cases of disagreement through a conflict resolution process. To expand the dataset, a semi-supervised approach was employed to automatically annotate the remaining 3,000 frames, forming LVVO_3k. The complete dataset offers a valuable resource for developing and evaluating both supervised and semi-supervised methods for visual content detection in educational videos. The LVVO dataset is publicly available to support further research in this domain.
Via
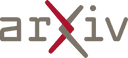
Jun 16, 2025
Abstract:This paper presents SEGO (Semantic Graph Ontology), a cognitive mapping architecture designed to integrate geometric perception, semantic reasoning, and explanation generation into a unified framework for human-centric collaborative robotics. SEGO constructs dynamic cognitive scene graphs that represent not only the spatial configuration of the environment but also the semantic relations and ontological consistency among detected objects. The architecture seamlessly combines SLAM-based localization, deep-learning-based object detection and tracking, and ontology-driven reasoning to enable real-time, semantically coherent mapping.
Via
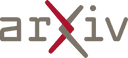
Jun 16, 2025
Abstract:The mining sector increasingly adopts digital tools to improve operational efficiency, safety, and data-driven decision-making. One of the key challenges remains the reliable acquisition of high-resolution, geo-referenced spatial information to support core activities such as extraction planning and on-site monitoring. This work presents an integrated system architecture that combines UAV-based sensing, LiDAR terrain modeling, and deep learning-based object detection to generate spatially accurate information for open-pit mining environments. The proposed pipeline includes geo-referencing, 3D reconstruction, and object localization, enabling structured spatial outputs to be integrated into an industrial digital twin platform. Unlike traditional static surveying methods, the system offers higher coverage and automation potential, with modular components suitable for deployment in real-world industrial contexts. While the current implementation operates in post-flight batch mode, it lays the foundation for real-time extensions. The system contributes to the development of AI-enhanced remote sensing in mining by demonstrating a scalable and field-validated geospatial data workflow that supports situational awareness and infrastructure safety.
Via
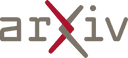