Topic:Information Extraction
What is Information Extraction? Information extraction is the process of automatically extracting structured information from unstructured text data.
Papers and Code
Jun 12, 2025
Abstract:The rapid proliferation of online video content necessitates effective video summarization techniques. Traditional methods, often relying on a single modality (typically visual), struggle to capture the full semantic richness of videos. This paper introduces MF2Summ, a novel video summarization model based on multimodal content understanding, integrating both visual and auditory information. MF2Summ employs a five-stage process: feature extraction, cross-modal attention interaction, feature fusion, segment prediction, and key shot selection. Visual features are extracted using a pre-trained GoogLeNet model, while auditory features are derived using SoundNet. The core of our fusion mechanism involves a cross-modal Transformer and an alignment-guided self-attention Transformer, designed to effectively model inter-modal dependencies and temporal correspondences. Segment importance, location, and center-ness are predicted, followed by key shot selection using Non-Maximum Suppression (NMS) and the Kernel Temporal Segmentation (KTS) algorithm. Experimental results on the SumMe and TVSum datasets demonstrate that MF2Summ achieves competitive performance, notably improving F1-scores by 1.9\% and 0.6\% respectively over the DSNet model, and performing favorably against other state-of-the-art methods.
Via
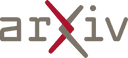
Jun 09, 2025
Abstract:This paper presents a novel framework for multi-talker automatic speech recognition without the need for auxiliary information. Serialized Output Training (SOT), a widely used approach, suffers from recognition errors due to speaker assignment failures. Although incorporating auxiliary information, such as token-level timestamps, can improve recognition accuracy, extracting such information from natural conversational speech remains challenging. To address this limitation, we propose Speaker-Distinguishable CTC (SD-CTC), an extension of CTC that jointly assigns a token and its corresponding speaker label to each frame. We further integrate SD-CTC into the SOT framework, enabling the SOT model to learn speaker distinction using only overlapping speech and transcriptions. Experimental comparisons show that multi-task learning with SD-CTC and SOT reduces the error rate of the SOT model by 26% and achieves performance comparable to state-of-the-art methods relying on auxiliary information.
* Accepted at INTERSPEECH 2025
Via
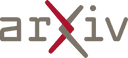
Jun 09, 2025
Abstract:Mathematical reasoning remains challenging for LLMs due to complex logic and the need for precise computation. Existing methods enhance LLM reasoning by synthesizing datasets through problem rephrasing, but face issues with generation quality and problem complexity. To address this, we propose to extract structural information with generated problem-solving code from mathematical reasoning and guide data generation with structured solutions. Applied to MATH and GSM8K, our approach produces 39K problems with labeled intermediate steps and a 6.1K-problem benchmark of higher difficulty. Results on our benchmark show that model performance declines as reasoning length increases. Additionally, we conducted fine-tuning experiments using the proposed training data on a range of LLMs, and the results validate the effectiveness of our dataset. We hope the proposed method and dataset will contribute to future research in enhancing LLM reasoning capabilities.
Via
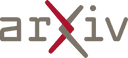
Jun 08, 2025
Abstract:Automatic Speech Recognition (ASR) has achieved remarkable success with deep learning, driving advancements in conversational artificial intelligence, media transcription, and assistive technologies. However, ASR systems still struggle in complex environments such as TV series, where overlapping speech, domain-specific terminology, and long-range contextual dependencies pose significant challenges to transcription accuracy. Existing multimodal approaches fail to correct ASR outputs with the rich temporal and contextual information available in video. To address this limitation, we propose a novel multimodal post-correction framework that refines ASR transcriptions by leveraging contextual cues extracted from video. Our framework consists of two stages: ASR Generation and Video-based Post-Correction, where the first stage produces the initial transcript and the second stage corrects errors using Video-based Contextual Information Extraction and Context-aware ASR Correction. We employ the Video-Large Multimodal Model (VLMM) to extract key contextual information using tailored prompts, which is then integrated with a Large Language Model (LLM) to refine the ASR output. We evaluate our method on a multimodal benchmark for TV series ASR and demonstrate its effectiveness in improving ASR performance by leveraging video-based context to enhance transcription accuracy in complex multimedia environments.
Via
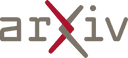
Jun 07, 2025
Abstract:Audio bandwidth extension is the task of reconstructing missing high frequency components of bandwidth-limited audio signals, where bandwidth limitation is a common issue for audio signals due to several reasons, including channel capacity and data constraints. While conventional spectral band replication is a well-established parametric approach to audio bandwidth extension, the SBR usually entails coarse feature extraction and reconstruction techniques, which leads to limitations when processing various types of audio signals. In parallel, numerous deep neural network-based audio bandwidth extension methods have been proposed. These DNN-based methods are usually referred to as blind BWE, as these methods do not rely on prior information extracted from original signals, and only utilize given low frequency band signals to estimate missing high frequency components. In order to replace conventional SBR with DNNs, simply adopting existing DNN-based methodologies results in suboptimal performance due to the blindness of these methods. My proposed research suggests a new approach to parametric non-blind bandwidth extension, as DNN-based side information extraction and DNN-based bandwidth extension are performed only at the front and end of the audio coding pipeline.
* Accepted to Interspeech 2025
Via
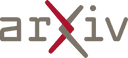
Jun 14, 2025
Abstract:Test-time adaptation (TTA) aims to boost the generalization capability of a trained model by conducting self-/unsupervised learning during the testing phase. While most existing TTA methods for video primarily utilize visual supervisory signals, they often overlook the potential contribution of inherent audio data. To address this gap, we propose a novel approach that incorporates audio information into video TTA. Our method capitalizes on the rich semantic content of audio to generate audio-assisted pseudo-labels, a new concept in the context of video TTA. Specifically, we propose an audio-to-video label mapping method by first employing pre-trained audio models to classify audio signals extracted from videos and then mapping the audio-based predictions to video label spaces through large language models, thereby establishing a connection between the audio categories and video labels. To effectively leverage the generated pseudo-labels, we present a flexible adaptation cycle that determines the optimal number of adaptation iterations for each sample, based on changes in loss and consistency across different views. This enables a customized adaptation process for each sample. Experimental results on two widely used datasets (UCF101-C and Kinetics-Sounds-C), as well as on two newly constructed audio-video TTA datasets (AVE-C and AVMIT-C) with various corruption types, demonstrate the superiority of our approach. Our method consistently improves adaptation performance across different video classification models and represents a significant step forward in integrating audio information into video TTA. Code: https://github.com/keikeiqi/Audio-Assisted-TTA.
* 14 pages, 7 figures
Via
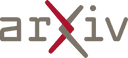
Jun 11, 2025
Abstract:As function approximators, deep neural networks have served as an effective tool to represent various signal types. Recent approaches utilize multi-layer perceptrons (MLPs) to learn a nonlinear mapping from a coordinate to its corresponding signal, facilitating the learning of continuous neural representations from discrete data points. Despite notable successes in learning diverse signal types, coordinate-based MLPs often face issues of overfitting and limited generalizability beyond the training region, resulting in subpar extrapolation performance. This study addresses scenarios where the underlying true signals exhibit periodic properties, either spatially or temporally. We propose a novel network architecture, which extracts periodic patterns from measurements and leverages this information to represent the signal, thereby enhancing generalization and improving extrapolation performance. We demonstrate the efficacy of the proposed method through comprehensive experiments, including the learning of the periodic solutions for differential equations, and time series imputation (interpolation) and forecasting (extrapolation) on real-world datasets.
Via
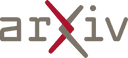
Jun 11, 2025
Abstract:Deep neural networks face several challenges in hyperspectral image classification, including high-dimensional data, sparse distribution of ground objects, and spectral redundancy, which often lead to classification overfitting and limited generalization capability. To more effectively extract and fuse spatial context with fine spectral information in hyperspectral image (HSI) classification, this paper proposes a novel network architecture called STNet. The core advantage of STNet stems from the dual innovative design of its Spatial-Spectral Transformer module: first, the fundamental explicit decoupling of spatial and spectral attention ensures targeted capture of key information in HSI; second, two functionally distinct gating mechanisms perform intelligent regulation at both the fusion level of attention flows (adaptive attention fusion gating) and the internal level of feature transformation (GFFN). This characteristic demonstrates superior feature extraction and fusion capabilities compared to traditional convolutional neural networks, while reducing overfitting risks in small-sample and high-noise scenarios. STNet enhances model representation capability without increasing network depth or width. The proposed method demonstrates superior performance on IN, UP, and KSC datasets, outperforming mainstream hyperspectral image classification approaches.
* arXiv admin note: substantial text overlap with arXiv:2504.15155,
arXiv:2504.13045, arXiv:2503.23472
Via
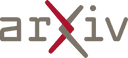
Jun 13, 2025
Abstract:The primary objective of the AIIB 2023 competition is to evaluate the predictive significance of airway-related imaging biomarkers in determining the survival outcomes of patients with lung fibrosis.This study introduces a comprehensive three-stage approach. Initially, a segmentation network, namely nn-Unet, is employed to delineate the airway's structural boundaries. Subsequently, key features are extracted from the radiomic images centered around the trachea and an enclosing bounding box around the airway. This step is motivated by the potential presence of critical survival-related insights within the tracheal region as well as pertinent information encoded in the structure and dimensions of the airway. Lastly, radiomic features obtained from the segmented areas are integrated into an SVM classifier. We could obtain an overall-score of 0.8601 for the segmentation in Task 1 while 0.7346 for the classification in Task 2.
* 8 pages
Via
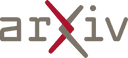
Jun 09, 2025
Abstract:Text-to-SQL enables non-experts to retrieve data from databases by converting natural language queries into SQL. However, state-of-the-art text-to-SQL studies rely on the BIRD dataset, which assumes that evidence is provided along with questions. Although BIRD facilitates research advancements, it assumes that users have expertise and domain knowledge, contradicting the fundamental goal of text-to-SQL. In addition, human-generated evidence in BIRD contains defects, including missing or erroneous evidence, which affects model performance. To address this issue, we propose SEED (System for Evidence Extraction and Domain knowledge generation), an approach that automatically generates evidence to improve performance and practical usability in real-world scenarios. SEED systematically analyzes database schema, description files, and values to extract relevant information. We evaluated SEED on BIRD and Spider, demonstrating that it significantly improves SQL generation accuracy in the no-evidence scenario, and in some cases, even outperforms the setting where BIRD evidence is provided. Our results highlight that SEED-generated evidence not only bridges the gap between research and real-world deployment but also improves the adaptability and robustness of text-to-SQL models. Our code is available at https://github.com/felix01189/SEED
* Proc. of IEEE ICDE Workshops (ICDEW), 2025
Via
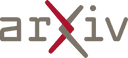