Topic:Information Extraction
What is Information Extraction? Information extraction is the process of automatically extracting structured information from unstructured text data.
Papers and Code
Jun 13, 2025
Abstract:Text-to-image diffusion models have achieved remarkable success, yet generating coherent image sequences for visual storytelling remains challenging. A key challenge is effectively leveraging all previous text-image pairs, referred to as history text-image pairs, which provide contextual information for maintaining consistency across frames. Existing auto-regressive methods condition on all past image-text pairs but require extensive training, while training-free subject-specific approaches ensure consistency but lack adaptability to narrative prompts. To address these limitations, we propose a multi-modal history adapter for text-to-image diffusion models, \textbf{ViSTA}. It consists of (1) a multi-modal history fusion module to extract relevant history features and (2) a history adapter to condition the generation on the extracted relevant features. We also introduce a salient history selection strategy during inference, where the most salient history text-image pair is selected, improving the quality of the conditioning. Furthermore, we propose to employ a Visual Question Answering-based metric TIFA to assess text-image alignment in visual storytelling, providing a more targeted and interpretable assessment of generated images. Evaluated on the StorySalon and FlintStonesSV dataset, our proposed ViSTA model is not only consistent across different frames, but also well-aligned with the narrative text descriptions.
Via
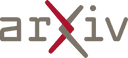
Jun 14, 2025
Abstract:Relation Extraction (RE) aims to extract semantic relationships in texts from given entity pairs, and has achieved significant improvements. However, in different domains, the RE task can be influenced by various factors. For example, in the financial domain, sentiment can affect RE results, yet this factor has been overlooked by modern RE models. To address this gap, this paper proposes a Sentiment-aware-SDP-Enhanced-Module (SSDP-SEM), a multi-task learning approach for enhancing financial RE. Specifically, SSDP-SEM integrates the RE models with a pluggable auxiliary sentiment perception (ASP) task, enabling the RE models to concurrently navigate their attention weights with the text's sentiment. We first generate detailed sentiment tokens through a sentiment model and insert these tokens into an instance. Then, the ASP task focuses on capturing nuanced sentiment information through predicting the sentiment token positions, combining both sentiment insights and the Shortest Dependency Path (SDP) of syntactic information. Moreover, this work employs a sentiment attention information bottleneck regularization method to regulate the reasoning process. Our experiment integrates this auxiliary task with several prevalent frameworks, and the results demonstrate that most previous models benefit from the auxiliary task, thereby achieving better results. These findings highlight the importance of effectively leveraging sentiment in the financial RE task.
Via
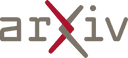
Jun 10, 2025
Abstract:Anomaly detection (AD) is a task that distinguishes normal and abnormal data, which is important for applying automation technologies of the manufacturing facilities. For MVTec dataset that is a representative AD dataset for industrial environment, many recent works have shown remarkable performances. However, the existing anomaly detection works have a limitation of showing good performance for fully-aligned datasets only, unlike real-world industrial environments. To solve this limitation, we propose HomographyAD, a novel deep anomaly detection methodology based on the ImageNet-pretrained network, which is specially designed for actual industrial dataset. Specifically, we first suggest input foreground alignment using the deep homography estimation method. In addition, we fine-tune the model by self homography learning to learn additional shape information from normal samples. Finally, we conduct anomaly detection based on the measure of how far the feature of test sample is from the distribution of the extracted normal features. By applying our proposed method to various existing AD approaches, we show performance enhancement through extensive experiments.
Via
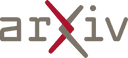
Jun 12, 2025
Abstract:Large language models (LLMs) often struggle with knowledge-intensive tasks due to a lack of background knowledge and a tendency to hallucinate. To address these limitations, integrating knowledge graphs (KGs) with LLMs has been intensively studied. Existing KG-enhanced LLMs focus on supplementary factual knowledge, but still struggle with solving complex questions. We argue that refining the relationships among facts and organizing them into a logically consistent reasoning path is equally important as factual knowledge itself. Despite their potential, extracting reliable reasoning paths from KGs poses the following challenges: the complexity of graph structures and the existence of multiple generated paths, making it difficult to distinguish between useful and redundant ones. To tackle these challenges, we propose the RRP framework to mine the knowledge graph, which combines the semantic strengths of LLMs with structural information obtained through relation embedding and bidirectional distribution learning. Additionally, we introduce a rethinking module that evaluates and refines reasoning paths according to their significance. Experimental results on two public datasets show that RRP achieves state-of-the-art performance compared to existing baseline methods. Moreover, RRP can be easily integrated into various LLMs to enhance their reasoning abilities in a plug-and-play manner. By generating high-quality reasoning paths tailored to specific questions, RRP distills effective guidance for LLM reasoning.
Via
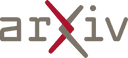
Jun 11, 2025
Abstract:Transformer-based models have achieved strong performance in remote sensing image captioning by capturing long-range dependencies and contextual information. However, their practical deployment is hindered by high computational costs, especially in multi-modal frameworks that employ separate transformer-based encoders and decoders. In addition, existing remote sensing image captioning models primarily focus on high-level semantic extraction while often overlooking fine-grained structural features such as edges, contours, and object boundaries. To address these challenges, a lightweight transformer architecture is proposed by reducing the dimensionality of the encoder layers and employing a distilled version of GPT-2 as the decoder. A knowledge distillation strategy is used to transfer knowledge from a more complex teacher model to improve the performance of the lightweight network. Furthermore, an edge-aware enhancement strategy is incorporated to enhance image representation and object boundary understanding, enabling the model to capture fine-grained spatial details in remote sensing images. Experimental results demonstrate that the proposed approach significantly improves caption quality compared to state-of-the-art methods.
Via
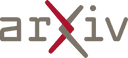
Jun 11, 2025
Abstract:Open-set domain generalization(OSDG) for hyperspectral image classification presents significant challenges due to the presence of unknown classes in target domains and the need for models to generalize across multiple unseen domains without target-specific adaptation. Existing domain adaptation methods assume access to target domain data during training and fail to address the fundamental issue of domain shift when unknown classes are present, leading to negative transfer and reduced classification performance. To address these limitations, we propose a novel open-set domain generalization framework that combines four key components: Spectrum-Invariant Frequency Disentanglement (SIFD) for domain-agnostic feature extraction, Dual-Channel Residual Network (DCRN) for robust spectral-spatial feature learning, Evidential Deep Learning (EDL) for uncertainty quantification, and Spectral-Spatial Uncertainty Disentanglement (SSUD) for reliable open-set classification. The SIFD module extracts domain-invariant spectral features in the frequency domain through attention-weighted frequency analysis and domain-agnostic regularization, while DCRN captures complementary spectral and spatial information via parallel pathways with adaptive fusion. EDL provides principled uncertainty estimation using Dirichlet distributions, enabling the SSUD module to make reliable open-set decisions through uncertainty-aware pathway weighting and adaptive rejection thresholding. Experimental results on three cross-scene hyperspectral classification tasks show that our approach achieves performance comparable to state-of-the-art domain adaptation methods while requiring no access to the target domain during training. The implementation will be made available at https://github.com/amir-khb/SSUDOSDG upon acceptance.
Via
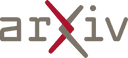
Jun 10, 2025
Abstract:Multivariate long-term and efficient time series forecasting is a key requirement for a variety of practical applications, and there are complex interleaving time dynamics in time series data that require decomposition modeling. Traditional time series decomposition methods are single and rely on fixed rules, which are insufficient for mining the potential information of the series and adapting to the dynamic characteristics of complex series. On the other hand, the Transformer-based models for time series forecasting struggle to effectively model long sequences and intricate dynamic relationships due to their high computational complexity. To overcome these limitations, we introduce KARMA, with an Adaptive Time Channel Decomposition module (ATCD) to dynamically extract trend and seasonal components. It further integrates a Hybrid Frequency-Time Decomposition module (HFTD) to further decompose Series into frequency-domain and time-domain. These components are coupled with multi-scale Mamba-based KarmaBlock to efficiently process global and local information in a coordinated manner. Experiments on eight real-world datasets from diverse domains well demonstrated that KARMA significantly outperforms mainstream baseline methods in both predictive accuracy and computational efficiency. Code and full results are available at this repository: https://github.com/yedadasd/KARMA
* 10 pages,3 figures, published to WASA2025
Via
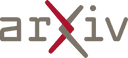
Jun 06, 2025
Abstract:Federated fine-tuning of large language models (FedLLMs) presents a promising approach for achieving strong model performance while preserving data privacy in sensitive domains. However, the inherent memorization ability of LLMs makes them vulnerable to training data extraction attacks. To investigate this risk, we introduce simple yet effective extraction attack algorithms specifically designed for FedLLMs. In contrast to prior "verbatim" extraction attacks, which assume access to fragments from all training data, our approach operates under a more realistic threat model, where the attacker only has access to a single client's data and aims to extract previously unseen personally identifiable information (PII) from other clients. This requires leveraging contextual prefixes held by the attacker to generalize across clients. To evaluate the effectiveness of our approaches, we propose two rigorous metrics-coverage rate and efficiency-and extend a real-world legal dataset with PII annotations aligned with CPIS, GDPR, and CCPA standards, achieving 89.9% human-verified precision. Experimental results show that our method can extract up to 56.57% of victim-exclusive PII, with "Address," "Birthday," and "Name" being the most vulnerable categories. Our findings underscore the pressing need for robust defense strategies and contribute a new benchmark and evaluation framework for future research in privacy-preserving federated learning.
* 10 pages, 4 figures
Via
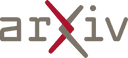
Jun 09, 2025
Abstract:How can we benefit from large models without sacrificing inference speed, a common dilemma in self-driving systems? A prevalent solution is a dual-system architecture, employing a small model for rapid, reactive decisions and a larger model for slower but more informative analyses. Existing dual-system designs often implement parallel architectures where inference is either directly conducted using the large model at each current frame or retrieved from previously stored inference results. However, these works still struggle to enable large models for a timely response to every online frame. Our key insight is to shift intensive computations of the current frame to previous time steps and perform a batch inference of multiple time steps to make large models respond promptly to each time step. To achieve the shifting, we introduce Efficiency through Thinking Ahead (ETA), an asynchronous system designed to: (1) propagate informative features from the past to the current frame using future predictions from the large model, (2) extract current frame features using a small model for real-time responsiveness, and (3) integrate these dual features via an action mask mechanism that emphasizes action-critical image regions. Evaluated on the Bench2Drive CARLA Leaderboard-v2 benchmark, ETA advances state-of-the-art performance by 8% with a driving score of 69.53 while maintaining a near-real-time inference speed at 50 ms.
* ICCV 2025 submission. For code, see
https://github.com/opendrivelab/ETA
Via
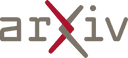
Jun 09, 2025
Abstract:Large language models (LLMs) exhibit pronounced conservative bias in relation extraction tasks, frequently defaulting to No_Relation label when an appropriate option is unavailable. While this behavior helps prevent incorrect relation assignments, our analysis reveals that it also leads to significant information loss when reasoning is not explicitly included in the output. We systematically evaluate this trade-off across multiple prompts, datasets, and relation types, introducing the concept of Hobson's choice to capture scenarios where models opt for safe but uninformative labels over hallucinated ones. Our findings suggest that conservative bias occurs twice as often as hallucination. To quantify this effect, we use SBERT and LLM prompts to capture the semantic similarity between conservative bias behaviors in constrained prompts and labels generated from semi-constrained and open-ended prompts.
* 10 pages
Via
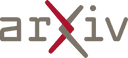