What is Topic Modeling? Topic modeling is a type of statistical modeling for discovering the abstract topics that occur in a collection of documents.
Papers and Code
May 03, 2025
Abstract:Biased news reporting poses a significant threat to informed decision-making and the functioning of democracies. This study introduces a novel methodology for scalable, minimally biased analysis of media bias in political news. The proposed approach examines event selection, labeling, word choice, and commission and omission biases across news sources by leveraging natural language processing techniques, including hierarchical topic modeling, sentiment analysis, and ontology learning with large language models. Through three case studies related to current political events, we demonstrate the methodology's effectiveness in identifying biases across news sources at various levels of granularity. This work represents a significant step towards scalable, minimally biased media bias analysis, laying the groundwork for tools to help news consumers navigate an increasingly complex media landscape.
* J Comput Soc Sc 8, 41 (2025)
Via
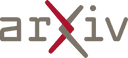
May 05, 2025
Abstract:Model averaging (MA) and ensembling play a crucial role in statistical and machine learning practice. When multiple candidate models are considered, MA techniques can be used to weight and combine them, often resulting in improved predictive accuracy and better estimation stability compared to model selection (MS) methods. In this paper, we address two challenges in combining least squares estimators from both theoretical and practical perspectives. We first establish several oracle inequalities for least squares MA via minimizing a Mallows' $C_p$ criterion under an arbitrary candidate model set. Compared to existing studies, these oracle inequalities yield faster excess risk and directly imply the asymptotic optimality of the resulting MA estimators under milder conditions. Moreover, we consider candidate model construction and investigate the problem of optimal all-subset combination for least squares estimators, which is an important yet rarely discussed topic in the existing literature. We show that there exists a fundamental limit to achieving the optimal all-subset MA risk. To attain this limit, we propose a novel Mallows-type MA procedure based on a dimension-adaptive $C_p$ criterion. The implicit ensembling effects of several MS procedures are also revealed and discussed. We conduct several numerical experiments to support our theoretical findings and demonstrate the effectiveness of the proposed Mallows-type MA estimator.
Via
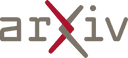
May 05, 2025
Abstract:Explainable Artificial Intelligence (XAI) has emerged as a pillar of Trustworthy AI and aims to bring transparency in complex models that are opaque by nature. Despite the benefits of incorporating explanations in models, an urgent need is found in addressing the privacy concerns of providing this additional information to end users. In this article, we conduct a scoping review of existing literature to elicit details on the conflict between privacy and explainability. Using the standard methodology for scoping review, we extracted 57 articles from 1,943 studies published from January 2019 to December 2024. The review addresses 3 research questions to present readers with more understanding of the topic: (1) what are the privacy risks of releasing explanations in AI systems? (2) what current methods have researchers employed to achieve privacy preservation in XAI systems? (3) what constitutes a privacy preserving explanation? Based on the knowledge synthesized from the selected studies, we categorize the privacy risks and preservation methods in XAI and propose the characteristics of privacy preserving explanations to aid researchers and practitioners in understanding the requirements of XAI that is privacy compliant. Lastly, we identify the challenges in balancing privacy with other system desiderata and provide recommendations for achieving privacy preserving XAI. We expect that this review will shed light on the complex relationship of privacy and explainability, both being the fundamental principles of Trustworthy AI.
* Submitted for peer review
Via
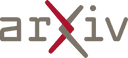
May 04, 2025
Abstract:Large language models (LLMs) have become integral to various real-world applications, leveraging massive, web-sourced datasets like Common Crawl, C4, and FineWeb for pretraining. While these datasets provide linguistic data essential for high-quality natural language generation, they often contain harmful content, such as hate speech, misinformation, and biased narratives. Training LLMs on such unfiltered data risks perpetuating toxic behaviors, spreading misinformation, and amplifying societal biases which can undermine trust in LLM-driven applications and raise ethical concerns about their use. This paper presents a large-scale analysis of inappropriate content across these datasets, offering a comprehensive taxonomy that categorizes harmful webpages into Topical and Toxic based on their intent. We also introduce a prompt evaluation dataset, a high-accuracy Topical and Toxic Prompt (TTP), and a transformer-based model (HarmFormer) for content filtering. Additionally, we create a new multi-harm open-ended toxicity benchmark (HAVOC) and provide crucial insights into how models respond to adversarial toxic inputs. Upon publishing, we will also opensource our model signal on the entire C4 dataset. Our work offers insights into ensuring safer LLM pretraining and serves as a resource for Responsible AI (RAI) compliance.
Via
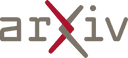
May 05, 2025
Abstract:Unsupervised contrastive learning has become a hot research topic in natural language processing. Existing works usually aim at constraining the orientation distribution of the representations of positive and negative samples in the high-dimensional semantic space in contrastive learning, but the semantic representation tensor possesses both modulus and orientation features, and the existing works ignore the modulus feature of the representations and cause insufficient contrastive learning. % Therefore, we firstly propose a training objective that aims at modulus constraints on the semantic representation tensor, to strengthen the alignment between the positive samples in contrastive learning. Therefore, we first propose a training objective that is designed to impose modulus constraints on the semantic representation tensor, to strengthen the alignment between positive samples in contrastive learning. Then, the BERT-like model suffers from the phenomenon of sinking attention, leading to a lack of attention to CLS tokens that aggregate semantic information. In response, we propose a cross-attention structure among the twin-tower ensemble models to enhance the model's attention to CLS token and optimize the quality of CLS Pooling. Combining the above two motivations, we propose a new \textbf{J}oint \textbf{T}ensor representation modulus constraint and \textbf{C}ross-attention unsupervised contrastive learning \textbf{S}entence \textbf{E}mbedding representation framework JTCSE, which we evaluate in seven semantic text similarity computation tasks, and the experimental results show that JTCSE's twin-tower ensemble model and single-tower distillation model outperform the other baselines and become the current SOTA. In addition, we have conducted an extensive zero-shot downstream task evaluation, which shows that JTCSE outperforms other baselines overall on more than 130 tasks.
Via
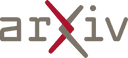
May 04, 2025
Abstract:Commercial Large Language Models (LLMs) have recently incorporated memory features to deliver personalised responses. This memory retains details such as user demographics and individual characteristics, allowing LLMs to adjust their behaviour based on personal information. However, the impact of integrating personalised information into the context has not been thoroughly assessed, leading to questions about its influence on LLM behaviour. Personalisation can be challenging, particularly with sensitive topics. In this paper, we examine various state-of-the-art LLMs to understand their behaviour in different personalisation scenarios, specifically focusing on hate speech. We prompt the models to assume country-specific personas and use different languages for hate speech detection. Our findings reveal that context personalisation significantly influences LLMs' responses in this sensitive area. To mitigate these unwanted biases, we fine-tune the LLMs by penalising inconsistent hate speech classifications made with and without country or language-specific context. The refined models demonstrate improved performance in both personalised contexts and when no context is provided.
Via
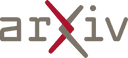
May 01, 2025
Abstract:Protecting cloud applications is crucial in an age where security constantly threatens the digital world. The inevitable cyber-attacks throughout the CI/CD pipeline make cloud security innovations necessary. This research is motivated by applying Natural Language Processing (NLP) methodologies, such as Topic Modelling, to analyse cloud security data and predict future attacks. This research aims to use topic modelling, specifically Latent Dirichlet Allocation (LDA) and Probabilistic Latent Semantic Analysis (pLSA). Utilising LDA and PLSA, security-related text data, such as reports, logs, and other relevant documents, will be analysed and sorted into relevant topics (such as phishing or encryption). These algorithms may apply through Python using the Gensim framework. The topics shall be utilised to detect vulnerabilities within relevant CI/CD pipeline records or log data. This application of Topic Modelling anticipates providing a new form of vulnerability detection, improving overall security throughout the CI/CD pipeline.
* 6 pages, 5 figures, 28th ACIS International Winter Conference on
Software Engineering, Artificial Intelligence, Networking and
Parallel/Distributed Computing (SNPD 2024-Winter)
Via
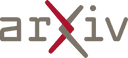
May 03, 2025
Abstract:We present a framework for large-scale sentiment and topic analysis of Twitter discourse. Our pipeline begins with targeted data collection using conflict-specific keywords, followed by automated sentiment labeling via multiple pre-trained models to improve annotation robustness. We examine the relationship between sentiment and contextual features such as timestamp, geolocation, and lexical content. To identify latent themes, we apply Latent Dirichlet Allocation (LDA) on partitioned subsets grouped by sentiment and metadata attributes. Finally, we develop an interactive visualization interface to support exploration of sentiment trends and topic distributions across time and regions. This work contributes a scalable methodology for social media analysis in dynamic geopolitical contexts.
Via
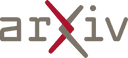
May 04, 2025
Abstract:Semantic control entails steering LM generations towards satisfying subtle non-lexical constraints, e.g., toxicity, sentiment, or politeness, attributes that can be captured by a sequence-level verifier. It can thus be viewed as sampling from the LM distribution conditioned on the target attribute, a computationally intractable problem due to the non-decomposable nature of the verifier. Existing approaches to LM control either only deal with syntactic constraints which cannot capture the aforementioned attributes, or rely on sampling to explore the conditional LM distribution, an ineffective estimator for low-probability events. In this work, we leverage a verifier's gradient information to efficiently reason over all generations that satisfy the target attribute, enabling precise steering of LM generations by reweighing the next-token distribution. Starting from an initial sample, we create a local LM distribution favoring semantically similar sentences. This approximation enables the tractable computation of an expected sentence embedding. We use this expected embedding, informed by the verifier's evaluation at the initial sample, to estimate the probability of satisfying the constraint, which directly informs the update to the next-token distribution. We evaluated the effectiveness of our approach in controlling the toxicity, sentiment, and topic-adherence of LMs yielding generations satisfying the constraint with high probability (>95%) without degrading their quality.
Via
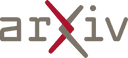
May 02, 2025
Abstract:Predicting the price that has the least error and can provide the best and highest accuracy has been one of the most challenging issues and one of the most critical concerns among capital market activists and researchers. Therefore, a model that can solve problems and provide results with high accuracy is one of the topics of interest among researchers. In this project, using time series prediction models such as ARIMA to estimate the price, variables, and indicators related to technical analysis show the behavior of traders involved in involving psychological factors for the model. By linking all of these variables to stepwise regression, we identify the best variables influencing the prediction of the variable. Finally, we enter the selected variables as inputs to the artificial neural network. In other words, we want to call this whole prediction process the "ARIMA_Stepwise Regression_Neural Network" model and try to predict the price of gold in international financial markets. This approach is expected to be able to be used to predict the types of stocks, commodities, currency pairs, financial market indicators, and other items used in local and international financial markets. Moreover, a comparison between the results of this method and time series methods is also expressed. Finally, based on the results, it can be seen that the resulting hybrid model has the highest accuracy compared to the time series method, regression, and stepwise regression.
Via
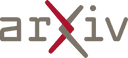