Topic:Time Series Analysis
What is Time Series Analysis? Time series analysis comprises statistical methods for analyzing a sequence of data points collected over an interval of time to identify interesting patterns and trends.
Papers and Code
Oct 21, 2024
Abstract:Time series analysis plays a critical role in numerous applications, supporting tasks such as forecasting, classification, anomaly detection, and imputation. In this work, we present the time series pattern machine (TSPM), a model designed to excel in a broad range of time series tasks through powerful representation and pattern extraction capabilities. Traditional time series models often struggle to capture universal patterns, limiting their effectiveness across diverse tasks. To address this, we define multiple scales in the time domain and various resolutions in the frequency domain, employing various mixing strategies to extract intricate, task-adaptive time series patterns. Specifically, we introduce a general-purpose TSPM that processes multi-scale time series using (1) multi-resolution time imaging (MRTI), (2) time image decomposition (TID), (3) multi-scale mixing (MCM), and (4) multi-resolution mixing (MRM) to extract comprehensive temporal patterns. MRTI transforms multi-scale time series into multi-resolution time images, capturing patterns across both temporal and frequency domains. TID leverages dual-axis attention to extract seasonal and trend patterns, while MCM hierarchically aggregates these patterns across scales. MRM adaptively integrates all representations across resolutions. This method achieves state-of-the-art performance across 8 time series analytical tasks, consistently surpassing both general-purpose and task-specific models. Our work marks a promising step toward the next generation of TSPMs, paving the way for further advancements in time series analysis.
Via
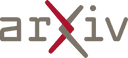
Oct 21, 2024
Abstract:We propose a new architecture for real-time anomaly detection in video data, inspired by human behavior by combining spatial and temporal analyses. This approach uses two distinct models: for temporal analysis, a recurrent convolutional network (CNN + RNN) is employed, associating VGG19 and a GRU to process video sequences. Regarding spatial analysis, it is performed using YOLOv7 to analyze individual images. These two analyses can be carried out either in parallel, with a final prediction that combines the results of both analyses, or in series, where the spatial analysis enriches the data before the temporal analysis. In this article, we will compare these two architectural configurations with each other, to evaluate the effectiveness of our hybrid approach in video anomaly detection.
Via
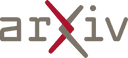
Oct 20, 2024
Abstract:Understanding events in time series is an important task in a variety of contexts. However, human analysis and labeling are expensive and time-consuming. Therefore, it is advantageous to learn embeddings for moments in time series in an unsupervised way, which allows for good performance in classification or detection tasks after later minimal human labeling. In this paper, we propose dynamic contrastive learning (DynaCL), an unsupervised contrastive representation learning framework for time series that uses temporal adjacent steps to define positive pairs. DynaCL adopts N-pair loss to dynamically treat all samples in a batch as positive or negative pairs, enabling efficient training and addressing the challenges of complicated sampling of positives. We demonstrate that DynaCL embeds instances from time series into semantically meaningful clusters, which allows superior performance on downstream tasks on a variety of public time series datasets. Our findings also reveal that high scores on unsupervised clustering metrics do not guarantee that the representations are useful in downstream tasks.
Via
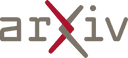
Oct 19, 2024
Abstract:In this study, we introduces a parameter-efficient model that outperforms traditional models in time series forecasting, by integrating High-order Polynomial Projection (HiPPO) theory into the Kolmogorov-Arnold network (KAN) framework. This HiPPO-KAN model achieves superior performance on long sequence data without increasing parameter count. Experimental results demonstrate that HiPPO-KAN maintains a constant parameter count while varying window sizes and prediction horizons, in contrast to KAN, whose parameter count increases linearly with window size. Surprisingly, although the HiPPO-KAN model keeps a constant parameter count as increasing window size, it significantly outperforms KAN model at larger window sizes. These results indicate that HiPPO-KAN offers significant parameter efficiency and scalability advantages for time series forecasting. Additionally, we address the lagging problem commonly encountered in time series forecasting models, where predictions fail to promptly capture sudden changes in the data. We achieve this by modifying the loss function to compute the MSE directly on the coefficient vectors in the HiPPO domain. This adjustment effectively resolves the lagging problem, resulting in predictions that closely follow the actual time series data. By incorporating HiPPO theory into KAN, this study showcases an efficient approach for handling long sequences with improved predictive accuracy, offering practical contributions for applications in large-scale time series data.
* 16 pages, 6 figures, 2 tables
Via
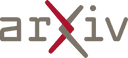
Oct 18, 2024
Abstract:In processing multiple time series, accounting for the individual features of each sequence can be challenging. To address this, modern deep learning methods for time series analysis combine a shared (global) model with local layers, specific to each time series, often implemented as learnable embeddings. Ideally, these local embeddings should encode meaningful representations of the unique dynamics of each sequence. However, when these are learned end-to-end as parameters of a forecasting model, they may end up acting as mere sequence identifiers. Shared processing blocks may then become reliant on such identifiers, limiting their transferability to new contexts. In this paper, we address this issue by investigating methods to regularize the learning of local learnable embeddings for time series processing. Specifically, we perform the first extensive empirical study on the subject and show how such regularizations consistently improve performance in widely adopted architectures. Furthermore, we show that methods preventing the co-adaptation of local and global parameters are particularly effective in this context. This hypothesis is validated by comparing several methods preventing the downstream models from relying on sequence identifiers, going as far as completely resetting the embeddings during training. The obtained results provide an important contribution to understanding the interplay between learnable local parameters and shared processing layers: a key challenge in modern time series processing models and a step toward developing effective foundation models for time series.
Via
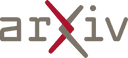
Oct 19, 2024
Abstract:This research is part of a systematic study of scientific time series. In the last three years, hundreds of papers and over fifty new deep-learning models have been described for time series models. These mainly focus on the key aspect of time dependence, whereas in some scientific time series, the situation is more complex with multiple locations, each location having multiple observed and target time-dependent streams and multiple exogenous (known) properties that are either constant or time-dependent. Here, we analyze the hydrology time series using the CAMELS and Caravan global datasets on catchment rainfall and runoff. Together, these have up to 6 observed streams and up to 209 static parameters defined at each of about 8000 locations. This analysis is fully open source with a Jupyter Notebook running on Google Colab for both an LSTM-based analysis and the data engineering preprocessing. Our goal is to investigate the importance of exogenous data, which we look at using eight different choices on representative hydrology tasks. Increasing the exogenous information significantly improves the data representation, with the mean square error decreasing to 60% of its initial value in the largest dataset examined. We present the initial results of studies of other deep-learning neural network architectures where the approaches that can use the full observed and exogenous observations outperform less flexible methods, including Foundation models. Using the natural annual periodic exogenous time series produces the largest impact, but the static and other periodic exogenous streams are also important. Our analysis is intended to be valuable as an educational resource and benchmark.
Via
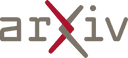
Oct 19, 2024
Abstract:Accurate time-series forecasting is essential across a multitude of scientific and industrial domains, yet deep learning models often struggle with challenges such as capturing long-term dependencies and adapting to drift in data distributions over time. We introduce Future-Guided Learning, an approach that enhances time-series event forecasting through a dynamic feedback mechanism inspired by predictive coding. Our approach involves two models: a detection model that analyzes future data to identify critical events and a forecasting model that predicts these events based on present data. When discrepancies arise between the forecasting and detection models, the forecasting model undergoes more substantial updates, effectively minimizing surprise and adapting to shifts in the data distribution by aligning its predictions with actual future outcomes. This feedback loop, drawing upon principles of predictive coding, enables the forecasting model to dynamically adjust its parameters, improving accuracy by focusing on features that remain relevant despite changes in the underlying data. We validate our method on a variety of tasks such as seizure prediction in biomedical signal analysis and forecasting in dynamical systems, achieving a 40\% increase in the area under the receiver operating characteristic curve (AUC-ROC) and a 10\% reduction in mean absolute error (MAE), respectively. By incorporating a predictive feedback mechanism that adapts to data distribution drift, Future-Guided Learning offers a promising avenue for advancing time-series forecasting with deep learning.
Via
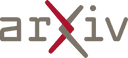
Oct 17, 2024
Abstract:Transformer models have consistently achieved remarkable results in various domains such as natural language processing and computer vision. However, despite ongoing research efforts to better understand these models, the field still lacks a comprehensive understanding. This is particularly true for deep time series forecasting methods, where analysis and understanding work is relatively limited. Time series data, unlike image and text information, can be more challenging to interpret and analyze. To address this, we approach the problem from a manifold learning perspective, assuming that the latent representations of time series forecasting models lie next to a low-dimensional manifold. In our study, we focus on analyzing the geometric features of these latent data manifolds, including intrinsic dimension and principal curvatures. Our findings reveal that deep transformer models exhibit similar geometric behavior across layers, and these geometric features are correlated with model performance. Additionally, we observe that untrained models initially have different structures, but they rapidly converge during training. By leveraging our geometric analysis and differentiable tools, we can potentially design new and improved deep forecasting neural networks. This approach complements existing analysis studies and contributes to a better understanding of transformer models in the context of time series forecasting. Code is released at https://github.com/azencot-group/GATLM.
* Accepted to TMLR 2024
Via
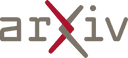
Oct 16, 2024
Abstract:Irregular Time Series Data (IRTS) has shown increasing prevalence in real-world applications. We observed that IRTS can be divided into two specialized types: Natural Irregular Time Series (NIRTS) and Accidental Irregular Time Series (AIRTS). Various existing methods either ignore the impacts of irregular patterns or statically learn the irregular dynamics of NIRTS and AIRTS data and suffer from limited data availability due to the sparsity of IRTS. We proposed a novel transformer-based framework for general irregular time series data that treats IRTS from four views: Locality, Time, Spatio and Irregularity to motivate the data usage to the highest potential. Moreover, we design a sophisticated irregularity-gate mechanism to adaptively select task-relevant information from irregularity, which improves the generalization ability to various IRTS data. We implement extensive experiments to demonstrate the resistance of our work to three highly missing ratio datasets (88.4\%, 94.9\%, 60\% missing value) and investigate the significance of the irregularity information for both NIRTS and AIRTS by additional ablation study. We release our implementation in https://github.com/IcurasLW/MTSFormer-Irregular_Time_Series.git
Via
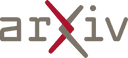
Oct 16, 2024
Abstract:Large Language Models have demonstrated impressive performance in many pivotal web applications such as sensor data analysis. However, since LLMs are not designed for time series tasks, simpler models like linear regressions can often achieve comparable performance with far less complexity. In this study, we perform extensive experiments to assess the effectiveness of applying LLMs to key time series tasks, including forecasting, classification, imputation, and anomaly detection. We compare the performance of LLMs against simpler baseline models, such as single-layer linear models and randomly initialized LLMs. Our results reveal that LLMs offer minimal advantages for these core time series tasks and may even distort the temporal structure of the data. In contrast, simpler models consistently outperform LLMs while requiring far fewer parameters. Furthermore, we analyze existing reprogramming techniques and show, through data manifold analysis, that these methods fail to effectively align time series data with language and display pseudo-alignment behaviour in embedding space. Our findings suggest that the performance of LLM-based methods in time series tasks arises from the intrinsic characteristics and structure of time series data, rather than any meaningful alignment with the language model architecture.
Via
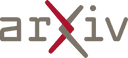