May 26, 2025
Abstract:A key research area in deepfake speech detection is source tracing - determining the origin of synthesised utterances. The approaches may involve identifying the acoustic model (AM), vocoder model (VM), or other generation-specific parameters. However, progress is limited by the lack of a dedicated, systematically curated dataset. To address this, we introduce STOPA, a systematically varied and metadata-rich dataset for deepfake speech source tracing, covering 8 AMs, 6 VMs, and diverse parameter settings across 700k samples from 13 distinct synthesisers. Unlike existing datasets, which often feature limited variation or sparse metadata, STOPA provides a systematically controlled framework covering a broader range of generative factors, such as the choice of the vocoder model, acoustic model, or pretrained weights, ensuring higher attribution reliability. This control improves attribution accuracy, aiding forensic analysis, deepfake detection, and generative model transparency.
* Accepted to Interspeech 2025 conference
Via
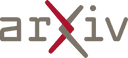