What is facial recognition? Facial recognition is an AI-based technique for identifying or confirming an individual's identity using their face. It maps facial features from an image or video and then compares the information with a collection of known faces to find a match.
Papers and Code
Nov 18, 2024
Abstract:This study proposes a novel approach for real-time facial expression recognition utilizing short-range Frequency-Modulated Continuous-Wave (FMCW) radar equipped with one transmit (Tx), and three receive (Rx) antennas. The system leverages four distinct modalities simultaneously: Range-Doppler images (RDIs), micro range-Doppler Images (micro-RDIs), range azimuth images (RAIs), and range elevation images (REIs). Our innovative architecture integrates feature extractor blocks, intermediate feature extractor blocks, and a ResNet block to accurately classify facial expressions into smile, anger, neutral, and no-face classes. Our model achieves an average classification accuracy of 98.91% on the dataset collected using a 60 GHz short-range FMCW radar. The proposed solution operates in real-time in a person-independent manner, which shows the potential use of low-cost FMCW radars for effective facial expression recognition in various applications.
* Accepted at IEEE SENSORS 2024
Via
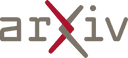
Nov 16, 2024
Abstract:Facial Emotion Recognition (FER) plays a crucial role in computer vision, with significant applications in human-computer interaction, affective computing, and areas such as mental health monitoring and personalized learning environments. However, a major challenge in FER task is the class imbalance commonly found in available datasets, which can hinder both model performance and generalization. In this paper, we tackle the issue of data imbalance by incorporating synthetic data augmentation and leveraging the ResEmoteNet model to enhance the overall performance on facial emotion recognition task. We employed Stable Diffusion 2 and Stable Diffusion 3 Medium models to generate synthetic facial emotion data, augmenting the training sets of the FER2013 and RAF-DB benchmark datasets. Training ResEmoteNet with these augmented datasets resulted in substantial performance improvements, achieving accuracies of 96.47% on FER2013 and 99.23% on RAF-DB. These findings shows an absolute improvement of 16.68% in FER2013, 4.47% in RAF-DB and highlight the efficacy of synthetic data augmentation in strengthening FER models and underscore the potential of advanced generative models in FER research and applications. The source code for ResEmoteNet is available at https://github.com/ArnabKumarRoy02/ResEmoteNet
* 5 pages, 4 tables, 4 figures, ICASSP 2025
Via
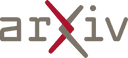
Jan 16, 2025
Abstract:Understanding emotions accurately is essential for fields like human-computer interaction. Due to the complexity of emotions and their multi-modal nature (e.g., emotions are influenced by facial expressions and audio), researchers have turned to using multi-modal models to understand human emotions rather than single-modality. However, current video multi-modal large language models (MLLMs) encounter difficulties in effectively integrating audio and identifying subtle facial micro-expressions. Furthermore, the lack of detailed emotion analysis datasets also limits the development of multimodal emotion analysis. To address these issues, we introduce a self-reviewed dataset and a human-reviewed dataset, comprising 24,137 coarse-grained samples and 3,500 manually annotated samples with detailed emotion annotations, respectively. These datasets allow models to learn from diverse scenarios and better generalize to real-world applications. Moreover, in addition to the audio modeling, we propose to explicitly integrate facial encoding models into the existing advanced Video MLLM, enabling the MLLM to effectively unify audio and the subtle facial cues for emotion understanding. By aligning these features within a unified space and employing instruction tuning in our proposed datasets, our Omni-Emotion achieves state-of-the-art performance in both emotion recognition and reasoning tasks.
Via
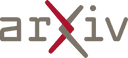
Nov 20, 2024
Abstract:Annotation ambiguity caused by the inherent subjectivity of visual judgment has always been a major challenge for Facial Expression Recognition (FER) tasks, particularly for largescale datasets from in-the-wild scenarios. A potential solution is the evaluation of relatively objective emotional distributions to help mitigate the ambiguity of subjective annotations. To this end, this paper proposes a novel Prior-based Objective Inference (POI) network. This network employs prior knowledge to derive a more objective and varied emotional distribution and tackles the issue of subjective annotation ambiguity through dynamic knowledge transfer. POI comprises two key networks: Firstly, the Prior Inference Network (PIN) utilizes the prior knowledge of AUs and emotions to capture intricate motion details. To reduce over-reliance on priors and facilitate objective emotional inference, PIN aggregates inferential knowledge from various key facial subregions, encouraging mutual learning. Secondly, the Target Recognition Network (TRN) integrates subjective emotion annotations and objective inference soft labels provided by the PIN, fostering an understanding of inherent facial expression diversity, thus resolving annotation ambiguity. Moreover, we introduce an uncertainty estimation module to quantify and balance facial expression confidence. This module enables a flexible approach to dealing with the uncertainties of subjective annotations. Extensive experiments show that POI exhibits competitive performance on both synthetic noisy datasets and multiple real-world datasets. All codes and training logs will be publicly available at https://github.com/liuhw01/POI.
Via
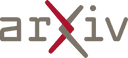
Jan 07, 2025
Abstract:The assessment of face image quality is crucial to ensure reliable face recognition. In order to provide data subjects and operators with explainable and actionable feedback regarding captured face images, relevant quality components have to be measured. Quality components that are known to negatively impact the utility of face images include JPEG and JPEG 2000 compression artefacts, among others. Compression can result in a loss of important image details which may impair the recognition performance. In this work, deep neural networks are trained to detect the compression artefacts in a face images. For this purpose, artefact-free facial images are compressed with the JPEG and JPEG 2000 compression algorithms. Subsequently, the PSNR and SSIM metrics are employed to obtain training labels based on which neural networks are trained using a single network to detect JPEG and JPEG 2000 artefacts, respectively. The evaluation of the proposed method shows promising results: in terms of detection accuracy, error rates of 2-3% are obtained for utilizing PSNR labels during training. In addition, we show that error rates of different open-source and commercial face recognition systems can be significantly reduced by discarding face images exhibiting severe compression artefacts. To minimize resource consumption, EfficientNetV2 serves as basis for the presented algorithm, which is available as part of the OFIQ software.
* 2nd Workshop on Fairness in Biometric Systems (FAIRBIO) at
International Conference on Pattern Recognition (ICPR) 2024
Via
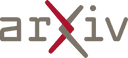
Nov 20, 2024
Abstract:Face recognition is a core task in computer vision designed to identify and authenticate individuals by analyzing facial patterns and features. This field intersects with artificial intelligence image processing and machine learning with applications in security authentication and personalization. Traditional approaches in facial recognition focus on capturing facial features like the eyes, nose and mouth and matching these against a database to verify identities. However challenges such as high false positive rates have persisted often due to the similarity among individuals facial features. Recently Contrastive Language Image Pretraining (CLIP) a model developed by OpenAI has shown promising advancements by linking natural language processing with vision tasks allowing it to generalize across modalities. Using CLIP's vision language correspondence and single-shot finetuning the model can achieve lower false positive rates upon deployment without the need of mass facial features extraction. This integration demonstrating CLIP's potential to address persistent issues in face recognition model performance without complicating our training paradigm.
Via
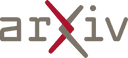
Nov 28, 2024
Abstract:Existing methods to generate aesthetic QR codes, such as image and style transfer techniques, tend to compromise either the visual appeal or the scannability of QR codes when they incorporate human face identity. Addressing these imperfections, we present Face2QR-a novel pipeline specifically designed for generating personalized QR codes that harmoniously blend aesthetics, face identity, and scannability. Our pipeline introduces three innovative components. First, the ID-refined QR integration (IDQR) seamlessly intertwines the background styling with face ID, utilizing a unified Stable Diffusion (SD)-based framework with control networks. Second, the ID-aware QR ReShuffle (IDRS) effectively rectifies the conflicts between face IDs and QR patterns, rearranging QR modules to maintain the integrity of facial features without compromising scannability. Lastly, the ID-preserved Scannability Enhancement (IDSE) markedly boosts scanning robustness through latent code optimization, striking a delicate balance between face ID, aesthetic quality and QR functionality. In comprehensive experiments, Face2QR demonstrates remarkable performance, outperforming existing approaches, particularly in preserving facial recognition features within custom QR code designs. Codes are available at $\href{https://github.com/cavosamir/Face2QR}{\text{this URL link}}$.
Via
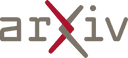
Dec 06, 2024
Abstract:The integration of conversational agents into our daily lives has become increasingly common, yet many of these agents cannot engage in deep interactions with humans. Despite this, there is a noticeable shortage of datasets that capture multimodal information from human-robot interaction dialogues. To address this gap, we have developed a Personal Emotional Robotic Conversational sYstem (PERCY) and recorded a novel multimodal dataset that encompasses rich embodied interaction data. The process involved asking participants to complete a questionnaire and gathering their profiles on ten topics, such as hobbies and favourite music. Subsequently, we initiated conversations between the robot and the participants, leveraging GPT-4 to generate contextually appropriate responses based on the participant's profile and emotional state, as determined by facial expression recognition and sentiment analysis. Automatic and user evaluations were conducted to assess the overall quality of the collected data. The results of both evaluations indicated a high level of naturalness, engagement, fluency, consistency, and relevance in the conversation, as well as the robot's ability to provide empathetic responses. It is worth noting that the dataset is derived from genuine interactions with the robot, involving participants who provided personal information and conveyed actual emotions.
* 9 pages, 5 Figures, Rejected from International Conference of Human
Robot Interaction 2025, Melbourne, Australia
Via
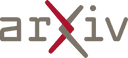
Nov 25, 2024
Abstract:Recent advancements in diffusion models have made generative image editing more accessible, enabling creative edits but raising ethical concerns, particularly regarding malicious edits to human portraits that threaten privacy and identity security. Existing protection methods primarily rely on adversarial perturbations to nullify edits but often fail against diverse editing requests. We propose FaceLock, a novel approach to portrait protection that optimizes adversarial perturbations to destroy or significantly alter biometric information, rendering edited outputs biometrically unrecognizable. FaceLock integrates facial recognition and visual perception into perturbation optimization to provide robust protection against various editing attempts. We also highlight flaws in commonly used evaluation metrics and reveal how they can be manipulated, emphasizing the need for reliable assessments of protection. Experiments show FaceLock outperforms baselines in defending against malicious edits and is robust against purification techniques. Ablation studies confirm its stability and broad applicability across diffusion-based editing algorithms. Our work advances biometric defense and sets the foundation for privacy-preserving practices in image editing. The code is available at: https://github.com/taco-group/FaceLock.
Via
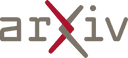
Nov 19, 2024
Abstract:Emotion Recognition (ER) is the process of identifying human emotions from given data. Currently, the field heavily relies on facial expression recognition (FER) because facial expressions contain rich emotional cues. However, it is important to note that facial expressions may not always precisely reflect genuine emotions and FER-based results may yield misleading ER. To understand and bridge this gap between FER and ER, we introduce eye behaviors as an important emotional cues for the creation of a new Eye-behavior-aided Multimodal Emotion Recognition (EMER) dataset. Different from existing multimodal ER datasets, the EMER dataset employs a stimulus material-induced spontaneous emotion generation method to integrate non-invasive eye behavior data, like eye movements and eye fixation maps, with facial videos, aiming to obtain natural and accurate human emotions. Notably, for the first time, we provide annotations for both ER and FER in the EMER, enabling a comprehensive analysis to better illustrate the gap between both tasks. Furthermore, we specifically design a new EMERT architecture to concurrently enhance performance in both ER and FER by efficiently identifying and bridging the emotion gap between the two.Specifically, our EMERT employs modality-adversarial feature decoupling and multi-task Transformer to augment the modeling of eye behaviors, thus providing an effective complement to facial expressions. In the experiment, we introduce seven multimodal benchmark protocols for a variety of comprehensive evaluations of the EMER dataset. The results show that the EMERT outperforms other state-of-the-art multimodal methods by a great margin, revealing the importance of modeling eye behaviors for robust ER. To sum up, we provide a comprehensive analysis of the importance of eye behaviors in ER, advancing the study on addressing the gap between FER and ER for more robust ER performance.
* The paper is part of ongoing work and we request to withdraw it from
arXiv to revise it further. And The paper was submitted without agreement
from all co-authors
Via
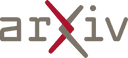