What is facial recognition? Facial recognition is an AI-based technique for identifying or confirming an individual's identity using their face. It maps facial features from an image or video and then compares the information with a collection of known faces to find a match.
Papers and Code
Dec 16, 2024
Abstract:Face alignment is a crucial step in preparing face images for feature extraction in facial analysis tasks. For applications such as face recognition, facial expression recognition, and facial attribute classification, alignment is widely utilized during both training and inference to standardize the positions of key landmarks in the face. It is well known that the application and method of face alignment significantly affect the performance of facial analysis models. However, the impact of alignment on face image quality has not been thoroughly investigated. Current FIQA studies often assume alignment as a prerequisite but do not explicitly evaluate how alignment affects quality metrics, especially with the advent of modern deep learning-based detectors that integrate detection and landmark localization. To address this need, our study examines the impact of face alignment on face image quality scores. We conducted experiments on the LFW, IJB-B, and SCFace datasets, employing MTCNN and RetinaFace models for face detection and alignment. To evaluate face image quality, we utilized several assessment methods, including SER-FIQ, FaceQAN, DifFIQA, and SDD-FIQA. Our analysis included examining quality score distributions for the LFW and IJB-B datasets and analyzing average quality scores at varying distances in the SCFace dataset. Our findings reveal that face image quality assessment methods are sensitive to alignment. Moreover, this sensitivity increases under challenging real-life conditions, highlighting the importance of evaluating alignment's role in quality assessment.
* Accepted at EAI ROSENET 2024 - 8th EAI International Conference on
Robotic Sensor Networks
Via
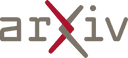
Dec 24, 2024
Abstract:In the domain of facial recognition security, multimodal Face Anti-Spoofing (FAS) is essential for countering presentation attacks. However, existing technologies encounter challenges due to modality biases and imbalances, as well as domain shifts. Our research introduces a Mixture of Experts (MoE) model to address these issues effectively. We identified three limitations in traditional MoE approaches to multimodal FAS: (1) Coarse-grained experts' inability to capture nuanced spoofing indicators; (2) Gated networks' susceptibility to input noise affecting decision-making; (3) MoE's sensitivity to prompt tokens leading to overfitting with conventional learning methods. To mitigate these, we propose the Bypass Isolated Gating MoE (BIG-MoE) framework, featuring: (1) Fine-grained experts for enhanced detection of subtle spoofing cues; (2) An isolation gating mechanism to counteract input noise; (3) A novel differential convolutional prompt bypass enriching the gating network with critical local features, thereby improving perceptual capabilities. Extensive experiments on four benchmark datasets demonstrate significant generalization performance improvement in multimodal FAS task. The code is released at https://github.com/murInJ/BIG-MoE.
* Accepted by ICASSP 2025
Via
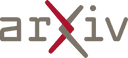
Dec 19, 2024
Abstract:Previous Deepfake detection methods perform well within their training domains, but their effectiveness diminishes significantly with new synthesis techniques. Recent studies have revealed that detection models often create decision boundaries based on facial identity rather than synthetic artifacts, resulting in poor performance on cross-domain datasets. To address this limitation, we propose Facial Recognition Identity Attenuation (FRIDAY), a novel training method that mitigates facial identity influence using a face recognizer. Specifically, we first train a face recognizer using the same backbone as the Deepfake detector. The recognizer is then frozen and employed during the detector's training to reduce facial identity information. This is achieved by feeding input images into both the recognizer and the detector, and minimizing the similarity of their feature embeddings through our Facial Identity Attenuating loss. This process encourages the detector to generate embeddings distinct from the recognizer, effectively reducing the impact of facial identity. Extensive experiments demonstrate that our approach significantly enhances detection performance on both in-domain and cross-domain datasets.
* 5 pages, 4 figures. In 2024 IEEE International Conference on Visual
Communications and Image Processing (VCIP) Oral
Via
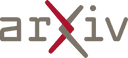
Nov 26, 2024
Abstract:Facial Expression Recognition has a wide application prospect in social robotics, health care, driver fatigue monitoring, and many other practical scenarios. Automatic recognition of facial expressions has been extensively studied by the Computer Vision research society. But Facial Expression Recognition in real-world is still a challenging task, partially due to the long-tailed distribution of the dataset. Many recent studies use data augmentation for Long-Tailed Recognition tasks. In this paper, we propose a novel semantic augmentation method. By introducing randomness into the encoding of the source data in the latent space of VAE-GAN, new samples are generated. Then, for facial expression recognition in RAF-DB dataset, we use our augmentation method to balance the long-tailed distribution. Our method can be used in not only FER tasks, but also more diverse data-hungry scenarios.
Via
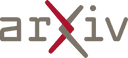
Feb 10, 2025
Abstract:The ethical, social and legal issues surrounding facial analysis technologies have been widely debated in recent years. Key critics have argued that these technologies can perpetuate bias and discrimination, particularly against marginalized groups. We contribute to this field of research by reporting on the limitations of facial analysis systems with the faces of people with Down syndrome: this particularly vulnerable group has received very little attention in the literature so far. This study involved the creation of a specific dataset of face images. An experimental group with faces of people with Down syndrome, and a control group with faces of people who are not affected by the syndrome. Two commercial tools were tested on the dataset, along three tasks: gender recognition, age prediction and face labelling. The results show an overall lower accuracy of prediction in the experimental group, and other specific patterns of performance differences: i) high error rates in gender recognition in the category of males with Down syndrome; ii) adults with Down syndrome were more often incorrectly labelled as children; iii) social stereotypes are propagated in both the control and experimental groups, with labels related to aesthetics more often associated with women, and labels related to education level and skills more often associated with men. These results, although limited in scope, shed new light on the biases that alter face classification when applied to faces of people with Down syndrome. They confirm the structural limitation of the technology, which is inherently dependent on the datasets used to train the models.
* Machine Learning and Principles and Practice of Knowledge
Discovery in Databases. ECML PKDD 2023. Communications in Computer and
Information Science, vol 2133. Springer, Cham
Via
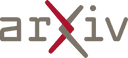
Dec 18, 2024
Abstract:This study demonstrates a novel approach to facial camouflage that combines targeted cosmetic perturbations and alpha transparency layer manipulation to evade modern facial recognition systems. Unlike previous methods -- such as CV dazzle, adversarial patches, and theatrical disguises -- this work achieves effective obfuscation through subtle modifications to key-point regions, particularly the brow, nose bridge, and jawline. Empirical testing with Haar cascade classifiers and commercial systems like BetaFaceAPI and Microsoft Bing Visual Search reveals that vertical perturbations near dense facial key points significantly disrupt detection without relying on overt disguises. Additionally, leveraging alpha transparency attacks in PNG images creates a dual-layer effect: faces remain visible to human observers but disappear in machine-readable RGB layers, rendering them unidentifiable during reverse image searches. The results highlight the potential for creating scalable, low-visibility facial obfuscation strategies that balance effectiveness and subtlety, opening pathways for defeating surveillance while maintaining plausible anonymity.
Via
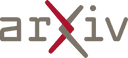
Dec 11, 2024
Abstract:Sentiment analysis and emotion recognition are crucial for applications such as human-computer interaction and depression detection. Traditional unimodal methods often fail to capture the complexity of emotional expressions due to conflicting signals from different modalities. Current Multimodal Large Language Models (MLLMs) also face challenges in detecting subtle facial expressions and addressing a wide range of emotion-related tasks. To tackle these issues, we propose M2SE, a Multistage Multitask Sentiment and Emotion Instruction Tuning Strategy for general-purpose MLLMs. It employs a combined approach to train models on tasks such as multimodal sentiment analysis, emotion recognition, facial expression recognition, emotion reason inference, and emotion cause-pair extraction. We also introduce the Emotion Multitask dataset (EMT), a custom dataset that supports these five tasks. Our model, Emotion Universe (EmoVerse), is built on a basic MLLM framework without modifications, yet it achieves substantial improvements across these tasks when trained with the M2SE strategy. Extensive experiments demonstrate that EmoVerse outperforms existing methods, achieving state-of-the-art results in sentiment and emotion tasks. These results highlight the effectiveness of M2SE in enhancing multimodal emotion perception. The dataset and code are available at https://github.com/xiaoyaoxinyi/M2SE.
Via
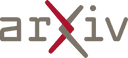
Dec 03, 2024
Abstract:Facial expression recognition (FER) systems raise significant privacy concerns due to the potential exposure of sensitive identity information. This paper presents a study on removing identity information while preserving FER capabilities. Drawing on the observation that low-frequency components predominantly contain identity information and high-frequency components capture expression, we propose a novel two-stream framework that applies privacy enhancement to each component separately. We introduce a controlled privacy enhancement mechanism to optimize performance and a feature compensator to enhance task-relevant features without compromising privacy. Furthermore, we propose a novel privacy-utility trade-off, providing a quantifiable measure of privacy preservation efficacy in closed-set FER tasks. Extensive experiments on the benchmark CREMA-D dataset demonstrate that our framework achieves 78.84% recognition accuracy with a privacy (facial identity) leakage ratio of only 2.01%, highlighting its potential for secure and reliable video-based FER applications.
* WACV2025 accepted
Via
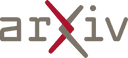
Jan 03, 2025
Abstract:Generalized age feature extraction is crucial for age-related facial analysis tasks, such as age estimation and age-invariant face recognition (AIFR). Despite the recent successes of models in homogeneous-dataset experiments, their performance drops significantly in cross-dataset evaluations. Most of these models fail to extract generalized age features as they only attempt to map extracted features with training age labels directly without explicitly modeling the natural progression of aging. In this paper, we propose Order-Enhanced Contrastive Learning (OrdCon), which aims to extract generalized age features to minimize the domain gap across different datasets and scenarios. OrdCon aligns the direction vector of two features with either the natural aging direction or its reverse to effectively model the aging process. The method also leverages metric learning which is incorporated with a novel soft proxy matching loss to ensure that features are positioned around the center of each age cluster with minimum intra-class variance. We demonstrate that our proposed method achieves comparable results to state-of-the-art methods on various benchmark datasets in homogeneous-dataset evaluations for both age estimation and AIFR. In cross-dataset experiments, our method reduces the mean absolute error by about 1.38 in average for age estimation task and boosts the average accuracy for AIFR by 1.87%.
Via
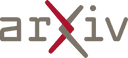
Nov 13, 2024
Abstract:This comprehensive review delves deeply into the various methodologies applied to facial expression recognition (FER) through the lens of graph representation learning (GRL). Initially, we introduce the task of FER and the concepts of graph representation and GRL. Afterward, we discuss some of the most prevalent and valuable databases for this task. We explore promising approaches for graph representation in FER, including graph diffusion, spatio-temporal graphs, and multi-stream architectures. Finally, we identify future research opportunities and provide concluding remarks.
Via
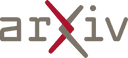