What is cancer detection? Cancer detection using Artificial Intelligence (AI) involves leveraging advanced machine learning algorithms and techniques to identify and diagnose cancer from various medical data sources. The goal is to enhance early detection, improve diagnostic accuracy, and potentially reduce the need for invasive procedures.
Papers and Code
Nov 11, 2024
Abstract:Current imaging-based prostate cancer diagnosis requires both MR T2-weighted (T2w) and diffusion-weighted imaging (DWI) sequences, with additional sequences for potentially greater accuracy improvement. However, measuring diffusion patterns in DWI sequences can be time-consuming, prone to artifacts and sensitive to imaging parameters. While machine learning (ML) models have demonstrated radiologist-level accuracy in detecting prostate cancer from these two sequences, this study investigates the potential of ML-enabled methods using only the T2w sequence as input during inference time. We first discuss the technical feasibility of such a T2-only approach, and then propose a novel ML formulation, where DWI sequences - readily available for training purposes - are only used to train a meta-learning model, which subsequently only uses T2w sequences at inference. Using multiple datasets from more than 3,000 prostate cancer patients, we report superior or comparable performance in localising radiologist-identified prostate cancer using our proposed T2-only models, compared with alternative models using T2-only or both sequences as input. Real patient cases are presented and discussed to demonstrate, for the first time, the exclusively true-positive cases from models with different input sequences.
Via
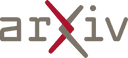
Dec 02, 2024
Abstract:Colorectal cancer is a prevalent form of cancer, and many patients develop colorectal cancer liver metastasis (CRLM) as a result. Early detection of CRLM is critical for improving survival rates. Radiologists usually rely on a series of multi-phase contrast-enhanced computed tomography (CECT) scans done during follow-up visits to perform early detection of the potential CRLM. These scans form unique five-dimensional data (time, phase, and axial, sagittal, and coronal planes in 3D CT). Most of the existing deep learning models can readily handle four-dimensional data (e.g., time-series 3D CT images) and it is not clear how well they can be extended to handle the additional dimension of phase. In this paper, we build a dataset of time-series CECT scans to aid in the early diagnosis of CRLM, and build upon state-of-the-art deep learning techniques to evaluate how to best predict CRLM. Our experimental results show that a multi-plane architecture based on 3D bi-directional LSTM, which we call MPBD-LSTM, works best, achieving an area under curve (AUC) of 0.79. On the other hand, analysis of the results shows that there is still great room for further improvement.
* MICCAI 2023; vol 14225; page 379-388
Via
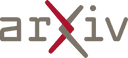
Oct 29, 2024
Abstract:Recent advances in machine learning are transforming medical image analysis, particularly in cancer detection and classification. Techniques such as deep learning, especially convolutional neural networks (CNNs) and vision transformers (ViTs), are now enabling the precise analysis of complex histopathological images, automating detection, and enhancing classification accuracy across various cancer types. This study focuses on osteosarcoma (OS), the most common bone cancer in children and adolescents, which affects the long bones of the arms and legs. Early and accurate detection of OS is essential for improving patient outcomes and reducing mortality. However, the increasing prevalence of cancer and the demand for personalized treatments create challenges in achieving precise diagnoses and customized therapies. We propose a novel hybrid model that combines convolutional neural networks (CNN) and vision transformers (ViT) to improve diagnostic accuracy for OS using hematoxylin and eosin (H&E) stained histopathological images. The CNN model extracts local features, while the ViT captures global patterns from histopathological images. These features are combined and classified using a Multi-Layer Perceptron (MLP) into four categories: non-tumor (NT), non-viable tumor (NVT), viable tumor (VT), and none-viable ratio (NVR). Using the Cancer Imaging Archive (TCIA) dataset, the model achieved an accuracy of 99.08%, precision of 99.10%, recall of 99.28%, and an F1-score of 99.23%. This is the first successful four-class classification using this dataset, setting a new benchmark in OS research and offering promising potential for future diagnostic advancements.
Via
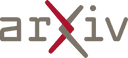
Dec 02, 2024
Abstract:Early detection of skin abnormalities plays a crucial role in diagnosing and treating skin cancer. Segmentation of affected skin regions using AI-powered devices is relatively common and supports the diagnostic process. However, achieving high performance remains a significant challenge due to the need for high-resolution images and the often unclear boundaries of individual lesions. At the same time, medical devices require segmentation models to have a small memory foot-print and low computational cost. Based on these requirements, we introduce a novel lightweight model called MambaU-Lite, which combines the strengths of Mamba and CNN architectures, featuring just over 400K parameters and a computational cost of more than 1G flops. To enhance both global context and local feature extraction, we propose the P-Mamba block, a novel component that incorporates VSS blocks along-side multiple pooling layers, enabling the model to effectively learn multiscale features and enhance segmentation performance. We evaluate the model's performance on two skin datasets, ISIC2018 and PH2, yielding promising results. Our source code will be made publicly available at: https://github.com/nqnguyen812/MambaU-Lite.
* 10 pages, 3 figures, 2 tables
Via
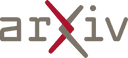
Nov 25, 2024
Abstract:Detection and differentiation of circulating tumor cells (CTCs) and non-CTCs in blood draws of cancer patients pose multiple challenges. While the gold standard relies on tedious manual evaluation of an automatically generated selection of images, machine learning (ML) techniques offer the potential to automate these processes. However, human assessment remains indispensable when the ML system arrives at uncertain or wrong decisions due to an insufficient set of labeled training data. This study introduces a human-in-the-loop (HiL) strategy for improving ML-based CTC detection. We combine self-supervised deep learning and a conventional ML-based classifier and propose iterative targeted sampling and labeling of new unlabeled training samples by human experts. The sampling strategy is based on the classification performance of local latent space clusters. The advantages of the proposed approach compared to naive random sampling are demonstrated for liquid biopsy data from patients with metastatic breast cancer.
Via
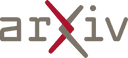
Nov 08, 2024
Abstract:In the United States, skin cancer ranks as the most commonly diagnosed cancer, presenting a significant public health issue due to its high rates of occurrence and the risk of serious complications if not caught early. Recent advancements in dataset curation and deep learning have shown promise in quick and accurate detection of skin cancer. However, current open-source datasets have significant class imbalances which impedes the effectiveness of these deep learning models. In healthcare, generative artificial intelligence (AI) models have been employed to create synthetic data, addressing data imbalance in datasets by augmenting underrepresented classes and enhancing the overall quality and performance of machine learning models. In this paper, we build on top of previous work by leveraging new advancements in generative AI, notably Stable Diffusion and DreamBooth. We introduce Cancer-Net SCa-Synth, an open access synthetically generated 2D skin lesion dataset for skin cancer classification. Further analysis on the data effectiveness by comparing the ISIC 2020 test set performance for training with and without these synthetic images for a simple model highlights the benefits of leveraging synthetic data to improve performance. Cancer-Net SCa-Synth is publicly available at https://github.com/catai9/Cancer-Net-SCa-Synth as part of a global open-source initiative for accelerating machine learning for cancer care.
Via
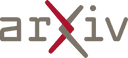
Nov 24, 2024
Abstract:MRI and CT are essential clinical cross-sectional imaging techniques for diagnosing complex conditions. However, large 3D datasets with annotations for deep learning are scarce. While methods like DINOv2 are encouraging for 2D image analysis, these methods have not been applied to 3D medical images. Furthermore, deep learning models often lack explainability due to their "black-box" nature. This study aims to extend 2D self-supervised models, specifically DINOv2, to 3D medical imaging while evaluating their potential for explainable outcomes. We introduce the Medical Slice Transformer (MST) framework to adapt 2D self-supervised models for 3D medical image analysis. MST combines a Transformer architecture with a 2D feature extractor, i.e., DINOv2. We evaluate its diagnostic performance against a 3D convolutional neural network (3D ResNet) across three clinical datasets: breast MRI (651 patients), chest CT (722 patients), and knee MRI (1199 patients). Both methods were tested for diagnosing breast cancer, predicting lung nodule dignity, and detecting meniscus tears. Diagnostic performance was assessed by calculating the Area Under the Receiver Operating Characteristic Curve (AUC). Explainability was evaluated through a radiologist's qualitative comparison of saliency maps based on slice and lesion correctness. P-values were calculated using Delong's test. MST achieved higher AUC values compared to ResNet across all three datasets: breast (0.94$\pm$0.01 vs. 0.91$\pm$0.02, P=0.02), chest (0.95$\pm$0.01 vs. 0.92$\pm$0.02, P=0.13), and knee (0.85$\pm$0.04 vs. 0.69$\pm$0.05, P=0.001). Saliency maps were consistently more precise and anatomically correct for MST than for ResNet. Self-supervised 2D models like DINOv2 can be effectively adapted for 3D medical imaging using MST, offering enhanced diagnostic accuracy and explainability compared to convolutional neural networks.
Via
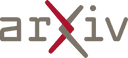
Nov 19, 2024
Abstract:Colonoscopy is crucial for identifying adenomatous polyps and preventing colorectal cancer. However, developing robust models for polyp detection is challenging by the limited size and accessibility of existing colonoscopy datasets. While previous efforts have attempted to synthesize colonoscopy images, current methods suffer from instability and insufficient data diversity. Moreover, these approaches lack precise control over the generation process, resulting in images that fail to meet clinical quality standards. To address these challenges, we propose CCIS-DIFF, a Controlled generative model for high-quality Colonoscopy Image Synthesis based on a Diffusion architecture. Our method offers precise control over both the spatial attributes (polyp location and shape) and clinical characteristics of polyps that align with clinical descriptions. Specifically, we introduce a blur mask weighting strategy to seamlessly blend synthesized polyps with the colonic mucosa, and a text-aware attention mechanism to guide the generated images to reflect clinical characteristics. Notably, to achieve this, we construct a new multi-modal colonoscopy dataset that integrates images, mask annotations, and corresponding clinical text descriptions. Experimental results demonstrate that our method generates high-quality, diverse colonoscopy images with fine control over both spatial constraints and clinical consistency, offering valuable support for downstream segmentation and diagnostic tasks.
* 5 pages, 4 figures
Via
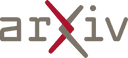
Nov 14, 2024
Abstract:Cervical cancer remains a major worldwide health issue, with early identification and risk assessment playing critical roles in effective preventive interventions. This paper presents the Cervix-AID-Net model for cervical precancer risk classification. The study designs and evaluates the proposed Cervix-AID-Net model based on patients colposcopy images. The model comprises a Convolutional Block Attention Module (CBAM) and convolutional layers that extract interpretable and representative features of colposcopic images to distinguish high-risk and low-risk cervical precancer. In addition, the proposed Cervix-AID-Net model integrates four explainable techniques, namely gradient class activation maps, Local Interpretable Model-agnostic Explanations, CartoonX, and pixel rate distortion explanation based on output feature maps and input features. The evaluation using holdout and ten-fold cross-validation techniques yielded a classification accuracy of 99.33\% and 99.81\%. The analysis revealed that CartoonX provides meticulous explanations for the decision of the Cervix-AID-Net model due to its ability to provide the relevant piece-wise smooth part of the image. The effect of Gaussian noise and blur on the input shows that the performance remains unchanged up to Gaussian noise of 3\% and blur of 10\%, while the performance reduces thereafter. A comparison study of the proposed model's performance compared to other deep learning approaches highlights the Cervix-AID-Net model's potential as a supplemental tool for increasing the effectiveness of cervical precancer risk assessment. The proposed method, which incorporates the CBAM and explainable artificial integration, has the potential to influence cervical cancer prevention and early detection, improving patient outcomes and lowering the worldwide burden of this preventable disease.
* 19 pages, 9 figure, and 7 tables
Via
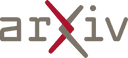
Dec 11, 2024
Abstract:Introduction: Global burden of sexually transmitted infections (STIs) is rising out of proportion to specialists. Current chatbots like ChatGPT are not tailored for handling STI-related concerns out of the box. We developed Otiz, an Artificial Intelligence-based (AI-based) chatbot platform designed specifically for STI detection and counseling, and assessed its performance. Methods: Otiz employs a multi-agent system architecture based on GPT4-0613, leveraging large language model (LLM) and Deterministic Finite Automaton principles to provide contextually relevant, medically accurate, and empathetic responses. Its components include modules for general STI information, emotional recognition, Acute Stress Disorder detection, and psychotherapy. A question suggestion agent operates in parallel. Four STIs (anogenital warts, herpes, syphilis, urethritis/cervicitis) and 2 non-STIs (candidiasis, penile cancer) were evaluated using prompts mimicking patient language. Each prompt was independently graded by two venereologists conversing with Otiz as patient actors on 6 criteria using Numerical Rating Scale ranging from 0 (poor) to 5 (excellent). Results: Twenty-three venereologists did 60 evaluations of 30 prompts. Across STIs, Otiz scored highly on diagnostic accuracy (4.1-4.7), overall accuracy (4.3-4.6), correctness of information (5.0), comprehensibility (4.2-4.4), and empathy (4.5-4.8). However, relevance scores were lower (2.9-3.6), suggesting some redundancy. Diagnostic scores for non-STIs were lower (p=0.038). Inter-observer agreement was strong, with differences greater than 1 point occurring in only 12.7% of paired evaluations. Conclusions: AI conversational agents like Otiz can provide accurate, correct, discrete, non-judgmental, readily accessible and easily understandable STI-related information in an empathetic manner, and can alleviate the burden on healthcare systems.
* 18 pages, 1 table
Via
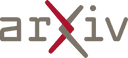