What is autonomous cars? Autonomous cars are self-driving vehicles that use artificial intelligence (AI) and sensors to navigate and operate without human intervention, using high-resolution cameras and lidars that detect what happens in the car's immediate surroundings. They have the potential to revolutionize transportation by improving safety, efficiency, and accessibility.
Papers and Code
Jul 02, 2025
Abstract:Most autonomous cars rely on the availability of high-definition (HD) maps. Current research aims to address this constraint by directly predicting HD map elements from onboard sensors and reasoning about the relationships between the predicted map and traffic elements. Despite recent advancements, the coherent online construction of HD maps remains a challenging endeavor, as it necessitates modeling the high complexity of road topologies in a unified and consistent manner. To address this challenge, we propose a coherent approach to predict lane segments and their corresponding topology, as well as road boundaries, all by leveraging prior map information represented by commonly available standard-definition (SD) maps. We propose a network architecture, which leverages hybrid lane segment encodings comprising prior information and denoising techniques to enhance training stability and performance. Furthermore, we facilitate past frames for temporal consistency. Our experimental evaluation demonstrates that our approach outperforms previous methods by a large margin, highlighting the benefits of our modeling scheme.
* Accepted at IROS 2025
Via
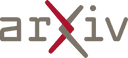
Jul 03, 2025
Abstract:Simulators are useful tools for testing automated driving controllers. Vehicle-in-the-loop (ViL) tests and digital twins (DTs) are widely used simulation technologies to facilitate the smooth deployment of controllers to physical vehicles. However, conventional ViL tests rely on full-size vehicles, requiring large space and high expenses. Also, physical-model-based DT suffers from the reality gap caused by modeling imprecision. This paper develops a comprehensive and practical simulator for testing automated driving controllers enhanced by scaled physical cars and AI-powered DT models. The scaled cars allow for saving space and expenses of simulation tests. The AI-powered DT models ensure superior simulation fidelity. Moreover, the simulator integrates well with off-the-shelf software and control algorithms, making it easy to extend. We use a filtered control benchmark with formal safety guarantees to showcase the capability of the simulator in validating automated driving controllers. Experimental studies are performed to showcase the efficacy of the simulator, implying its great potential in validating control solutions for autonomous vehicles and intelligent traffic.
Via
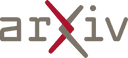
Jul 03, 2025
Abstract:We present CoInfra, a large-scale cooperative infrastructure perception system and dataset designed to advance robust multi-agent perception under real-world and adverse weather conditions. The CoInfra system includes 14 fully synchronized sensor nodes, each equipped with dual RGB cameras and a LiDAR, deployed across a shared region and operating continuously to capture all traffic participants in real-time. A robust, delay-aware synchronization protocol and a scalable system architecture that supports real-time data fusion, OTA management, and remote monitoring are provided in this paper. On the other hand, the dataset was collected in different weather scenarios, including sunny, rainy, freezing rain, and heavy snow and includes 195k LiDAR frames and 390k camera images from 8 infrastructure nodes that are globally time-aligned and spatially calibrated. Furthermore, comprehensive 3D bounding box annotations for five object classes (i.e., car, bus, truck, person, and bicycle) are provided in both global and individual node frames, along with high-definition maps for contextual understanding. Baseline experiments demonstrate the trade-offs between early and late fusion strategies, the significant benefits of HD map integration are discussed. By openly releasing our dataset, codebase, and system documentation at https://github.com/NingMingHao/CoInfra, we aim to enable reproducible research and drive progress in infrastructure-supported autonomous driving, particularly in challenging, real-world settings.
* This paper has been submitted to the IEEE Transactions on Robotics
for review
Via
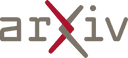
Jun 26, 2025
Abstract:Recent advances in scene reconstruction have pushed toward highly realistic modeling of autonomous driving (AD) environments using 3D Gaussian splatting. However, the resulting reconstructions remain closely tied to the original observations and struggle to support photorealistic synthesis of significantly altered or novel driving scenarios. This work introduces MADrive, a memory-augmented reconstruction framework designed to extend the capabilities of existing scene reconstruction methods by replacing observed vehicles with visually similar 3D assets retrieved from a large-scale external memory bank. Specifically, we release MAD-Cars, a curated dataset of ${\sim}70$K 360{\deg} car videos captured in the wild and present a retrieval module that finds the most similar car instances in the memory bank, reconstructs the corresponding 3D assets from video, and integrates them into the target scene through orientation alignment and relighting. The resulting replacements provide complete multi-view representations of vehicles in the scene, enabling photorealistic synthesis of substantially altered configurations, as demonstrated in our experiments. Project page: https://yandex-research.github.io/madrive/
Via
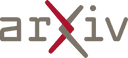
Jun 17, 2025
Abstract:Accurate and interpretable car-following models are essential for traffic simulation and autonomous vehicle development. However, classical models like the Intelligent Driver Model (IDM) are fundamentally limited by their parsimonious and single-regime structure. They fail to capture the multi-modal nature of human driving, where a single driving state (e.g., speed, relative speed, and gap) can elicit many different driver actions. This forces the model to average across distinct behaviors, reducing its fidelity and making its parameters difficult to interpret. To overcome this, we introduce a regime-switching framework that allows driving behavior to be governed by different IDM parameter sets, each corresponding to an interpretable behavioral mode. This design enables the model to dynamically switch between interpretable behavioral modes, rather than averaging across diverse driving contexts. We instantiate the framework using a Factorial Hidden Markov Model with IDM dynamics (FHMM-IDM), which explicitly separates intrinsic driving regimes (e.g., aggressive acceleration, steady-state following) from external traffic scenarios (e.g., free-flow, congestion, stop-and-go) through two independent latent Markov processes. Bayesian inference via Markov chain Monte Carlo (MCMC) is used to jointly estimate the regime-specific parameters, transition dynamics, and latent state trajectories. Experiments on the HighD dataset demonstrate that FHMM-IDM uncovers interpretable structure in human driving, effectively disentangling internal driver actions from contextual traffic conditions and revealing dynamic regime-switching patterns. This framework provides a tractable and principled solution to modeling context-dependent driving behavior under uncertainty, offering improvements in the fidelity of traffic simulations, the efficacy of safety analyses, and the development of more human-centric ADAS.
Via
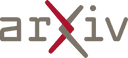
Jun 16, 2025
Abstract:Kinodynamic planning of articulated vehicles in cluttered environments faces additional challenges arising from high-dimensional state space and complex system dynamics. Built upon [1],[2], this work proposes the DE-AGT algorithm that grows a tree using pre-computed motion primitives (MPs) and A* heuristics. The first feature of DE-AGT is a delayed expansion of MPs. In particular, the MPs are divided into different modes, which are ranked online. With the MP classification and prioritization, DE-AGT expands the most promising mode of MPs first, which eliminates unnecessary computation and finds solutions faster. To obtain the cost-to-go heuristic for nonholonomic articulated vehicles, we rely on supervised learning and train neural networks for fast and accurate cost-to-go prediction. The learned heuristic is used for online mode ranking and node selection. Another feature of DE-AGT is the improved goal-reaching. Exactly reaching a goal state usually requires a constant connection checking with the goal by solving steering problems -- non-trivial and time-consuming for articulated vehicles. The proposed termination scheme overcomes this challenge by tightly integrating a light-weight trajectory tracking controller with the search process. DE-AGT is implemented for autonomous parking of a general car-like tractor with 3-trailer. Simulation results show an average of 10x acceleration compared to a previous method.
Via
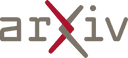
Jun 09, 2025
Abstract:This paper describes the development of an autonomous car by the UruBots team for the 2025 FIRA Autonomous Cars Challenge (Pro). The project involves constructing a compact electric vehicle, approximately the size of an RC car, capable of autonomous navigation through different tracks. The design incorporates mechanical and electronic components and machine learning algorithms that enable the vehicle to make real-time navigation decisions based on visual input from a camera. We use deep learning models to process camera images and control vehicle movements. Using a dataset of over ten thousand images, we trained a Convolutional Neural Network (CNN) to drive the vehicle effectively, through two outputs, steering and throttle. The car completed the track in under 30 seconds, achieving a pace of approximately 0.4 meters per second while avoiding obstacles.
Via
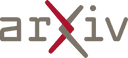
Jun 10, 2025
Abstract:Wireless communication-based multi-robot systems open the door to cyberattacks that can disrupt safety and performance of collaborative robots. The physical channel supporting inter-robot communication offers an attractive opportunity to decouple the detection of malicious robots from task-relevant data exchange between legitimate robots. Yet, trustworthiness indications coming from physical channels are uncertain and must be handled with this in mind. In this paper, we propose a resilient protocol for multi-robot operation wherein a parameter {\lambda}t accounts for how confident a robot is about the legitimacy of nearby robots that the physical channel indicates. Analytical results prove that our protocol achieves resilient coordination with arbitrarily many malicious robots under mild assumptions. Tuning {\lambda}t allows a designer to trade between near-optimal inter-robot coordination and quick task execution; see Fig. 1. This is a fundamental performance tradeoff and must be carefully evaluated based on the task at hand. The effectiveness of our approach is numerically verified with experiments involving platoons of autonomous cars where some vehicles are maliciously spoofed.
* This work has been submitted to IEEE for possible publication
Via
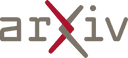
Jun 13, 2025
Abstract:Image-based 3D object detection is an inevitable part of autonomous driving because cheap onboard cameras are already available in most modern cars. Because of the accurate depth information, currently, most state-of-the-art 3D object detectors heavily rely on LiDAR data. In this paper, we propose a pipeline which lifts the results of existing vision-based 2D algorithms to 3D detections using only cameras as a cost-effective alternative to LiDAR. In contrast to existing approaches, we focus not only on cars but on all types of road users. To the best of our knowledge, we are the first using a 2D CNN to process the point cloud for each 2D detection to keep the computational effort as low as possible. Our evaluation on the challenging KITTI 3D object detection benchmark shows results comparable to state-of-the-art image-based approaches while having a runtime of only a third.
* 2020 IEEE 23rd International Conference on Information Fusion
(FUSION)
* https://ieeexplore.ieee.org/document/9190325
Via
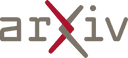
Jun 13, 2025
Abstract:In recent years, the development of interconnected devices has expanded in many fields, from infotainment to education and industrial applications. This trend has been accelerated by the increased number of sensors and accessibility to powerful hardware and software. One area that significantly benefits from these advancements is Teleoperated Driving (TD). In this scenario, a controller drives safely a vehicle from remote leveraging sensors data generated onboard the vehicle, and exchanged via Vehicle-to-Everything (V2X) communications. In this work, we tackle the problem of detecting the presence of cars and pedestrians from point cloud data to enable safe TD operations. More specifically, we exploit the SELMA dataset, a multimodal, open-source, synthetic dataset for autonomous driving, that we expanded by including the ground-truth bounding boxes of 3D objects to support object detection. We analyze the performance of state-of-the-art compression algorithms and object detectors under several metrics, including compression efficiency, (de)compression and inference time, and detection accuracy. Moreover, we measure the impact of compression and detection on the V2X network in terms of data rate and latency with respect to 3GPP requirements for TD applications.
* Submitted to IEEE Transactions on Intelligent Transportation Systems
Via
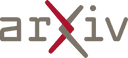