Topic:Lung Nodule Detection
What is Lung Nodule Detection? Lung-nodule detection is the process of identifying and localizing nodules in lung CT scans for early diagnosis of lung cancer.
Papers and Code
Apr 30, 2025
Abstract:Objective: A number of machine learning models have utilized semantic features, deep features, or both to assess lung nodule malignancy. However, their reliance on manual annotation during inference, limited interpretability, and sensitivity to imaging variations hinder their application in real-world clinical settings. Thus, this research aims to integrate semantic features derived from radiologists' assessments of nodules, allowing the model to learn clinically relevant, robust, and explainable features for predicting lung cancer. Methods: We obtained 938 low-dose CT scans from the National Lung Screening Trial with 1,246 nodules and semantic features. The Lung Image Database Consortium dataset contains 1,018 CT scans, with 2,625 lesions annotated for nodule characteristics. Three external datasets were obtained from UCLA Health, the LUNGx Challenge, and the Duke Lung Cancer Screening. We finetuned a pretrained Contrastive Language-Image Pretraining model with a parameter-efficient fine-tuning approach to align imaging and semantic features and predict the one-year lung cancer diagnosis. Results: We evaluated the performance of the one-year diagnosis of lung cancer with AUROC and AUPRC and compared it to three state-of-the-art models. Our model demonstrated an AUROC of 0.90 and AUPRC of 0.78, outperforming baseline state-of-the-art models on external datasets. Using CLIP, we also obtained predictions on semantic features, such as nodule margin (AUROC: 0.81), nodule consistency (0.81), and pleural attachment (0.84), that can be used to explain model predictions. Conclusion: Our approach accurately classifies lung nodules as benign or malignant, providing explainable outputs, aiding clinicians in comprehending the underlying meaning of model predictions. This approach also prevents the model from learning shortcuts and generalizes across clinical settings.
Via
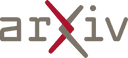
Apr 27, 2025
Abstract:Lung cancer, a leading cause of cancer-related deaths globally, emphasises the importance of early detection for better patient outcomes. Pulmonary nodules, often early indicators of lung cancer, necessitate accurate, timely diagnosis. Despite Explainable Artificial Intelligence (XAI) advances, many existing systems struggle providing clear, comprehensive explanations, especially with limited labelled data. This study introduces MERA, a Multimodal and Multiscale self-Explanatory model designed for lung nodule diagnosis with considerably Reduced Annotation requirements. MERA integrates unsupervised and weakly supervised learning strategies (self-supervised learning techniques and Vision Transformer architecture for unsupervised feature extraction) and a hierarchical prediction mechanism leveraging sparse annotations via semi-supervised active learning in the learned latent space. MERA explains its decisions on multiple levels: model-level global explanations via semantic latent space clustering, instance-level case-based explanations showing similar instances, local visual explanations via attention maps, and concept explanations using critical nodule attributes. Evaluations on the public LIDC dataset show MERA's superior diagnostic accuracy and self-explainability. With only 1% annotated samples, MERA achieves diagnostic accuracy comparable to or exceeding state-of-the-art methods requiring full annotation. The model's inherent design delivers comprehensive, robust, multilevel explanations aligned closely with clinical practice, enhancing trustworthiness and transparency. Demonstrated viability of unsupervised and weakly supervised learning lowers the barrier to deploying diagnostic AI in broader medical domains. Our complete code is open-source available: https://github.com/diku-dk/credanno.
Via
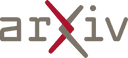
Apr 09, 2025
Abstract:In the U.S., lung cancer is the second major cause of death. Early detection of suspicious lung nodules is crucial for patient treatment planning, management, and improving outcomes. Many approaches for lung nodule segmentation and volumetric analysis have been proposed, but few have looked at longitudinal changes in total lung tumor burden. In this work, we trained two 3D models (nnUNet) with and without anatomical priors to automatically segment lung lesions and quantified total lesion burden for each patient. The 3D model without priors significantly outperformed ($p < .001$) the model trained with anatomy priors. For detecting clinically significant lesions $>$ 1cm, a precision of 71.3\%, sensitivity of 68.4\%, and F1-score of 69.8\% was achieved. For segmentation, a Dice score of 77.1 $\pm$ 20.3 and Hausdorff distance error of 11.7 $\pm$ 24.1 mm was obtained. The median lesion burden was 6.4 cc (IQR: 2.1, 18.1) and the median volume difference between manual and automated measurements was 0.02 cc (IQR: -2.8, 1.2). Agreements were also evaluated with linear regression and Bland-Altman plots. The proposed approach can produce a personalized evaluation of the total tumor burden for a patient and facilitate interval change tracking over time.
* Published at SPIE Medical Imaging 2025
Via
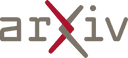
Apr 09, 2025
Abstract:Lung cancer remains one of the leading causes of cancer-related mortality worldwide, with early and accurate diagnosis playing a pivotal role in improving patient outcomes. Automated detection of pulmonary nodules in computed tomography (CT) scans is a challenging task due to variability in nodule size, shape, texture, and location. Traditional Convolutional Neural Networks (CNNs) have shown considerable promise in medical image analysis; however, their limited ability to capture fine-grained spatial-spectral variations restricts their performance in complex diagnostic scenarios. In this study, we propose a novel hybrid deep learning architecture that incorporates Chebyshev polynomial expansions into CNN layers to enhance expressive power and improve the representation of underlying anatomical structures. The proposed Chebyshev-CNN leverages the orthogonality and recursive properties of Chebyshev polynomials to extract high-frequency features and approximate complex nonlinear functions with greater fidelity. The model is trained and evaluated on benchmark lung cancer imaging datasets, including LUNA16 and LIDC-IDRI, achieving superior performance in classifying pulmonary nodules as benign or malignant. Quantitative results demonstrate significant improvements in accuracy, sensitivity, and specificity compared to traditional CNN-based approaches. This integration of polynomial-based spectral approximation within deep learning provides a robust framework for enhancing automated medical diagnostics and holds potential for broader applications in clinical decision support systems.
Via
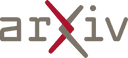
Mar 13, 2025
Abstract:The integration of Internet of Things (IoT) technology in pulmonary nodule detection significantly enhances the intelligence and real-time capabilities of the detection system. Currently, lung nodule detection primarily focuses on the identification of solid nodules, but different types of lung nodules correspond to various forms of lung cancer. Multi-type detection contributes to improving the overall lung cancer detection rate and enhancing the cure rate. To achieve high sensitivity in nodule detection, targeted improvements were made to the YOLOv8 model. Firstly, the C2f\_RepViTCAMF module was introduced to augment the C2f module in the backbone, thereby enhancing detection accuracy for small lung nodules and achieving a lightweight model design. Secondly, the MSCAF module was incorporated to reconstruct the feature fusion section of the model, improving detection accuracy for lung nodules of varying scales. Furthermore, the KAN network was integrated into the model. By leveraging the KAN network's powerful nonlinear feature learning capability, detection accuracy for small lung nodules was further improved, and the model's generalization ability was enhanced. Tests conducted on the LUNA16 dataset demonstrate that the improved model outperforms the original model as well as other mainstream models such as YOLOv9 and RT-DETR across various evaluation metrics.
Via
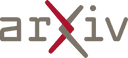
Mar 03, 2025
Abstract:Lung cancer has the highest rate of cancer-caused deaths, and early-stage diagnosis could increase the survival rate. Lung nodules are common indicators of lung cancer, making their detection crucial. Various lung nodule detection models exist, but many lack efficiency. Hence, we propose a more efficient approach by leveraging 2D CT slices, reducing computational load and complexity in training and inference. We employ the tiny version of Swin Transformer to benefit from Vision Transformers (ViT) while maintaining low computational cost. A Feature Pyramid Network is added to enhance detection, particularly for small nodules. Additionally, Transfer Learning is used to accelerate training. Our experimental results show that the proposed model outperforms state-of-the-art methods, achieving higher mAP and mAR for small nodules by 1.3% and 1.6%, respectively. Overall, our model achieves the highest mAP of 94.7% and mAR of 94.9%.
* 19th Iranian Conference on Intelligent Systems (ICIS), IEEE, 2024
Via
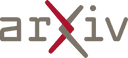
Mar 11, 2025
Abstract:Lymph node (LN) assessment is an essential task in the routine radiology workflow, providing valuable insights for cancer staging, treatment planning and beyond. Identifying scatteredly-distributed and low-contrast LNs in 3D CT scans is highly challenging, even for experienced clinicians. Previous lesion and LN detection methods demonstrate effectiveness of 2.5D approaches (i.e, using 2D network with multi-slice inputs), leveraging pretrained 2D model weights and showing improved accuracy as compared to separate 2D or 3D detectors. However, slice-based 2.5D detectors do not explicitly model inter-slice consistency for LN as a 3D object, requiring heuristic post-merging steps to generate final 3D LN instances, which can involve tuning a set of parameters for each dataset. In this work, we formulate 3D LN detection as a tracking task and propose LN-Tracker, a novel LN tracking transformer, for joint end-to-end detection and 3D instance association. Built upon DETR-based detector, LN-Tracker decouples transformer decoder's query into the track and detection groups, where the track query autoregressively follows previously tracked LN instances along the z-axis of a CT scan. We design a new transformer decoder with masked attention module to align track query's content to the context of current slice, meanwhile preserving detection query's high accuracy in current slice. An inter-slice similarity loss is introduced to encourage cohesive LN association between slices. Extensive evaluation on four lymph node datasets shows LN-Tracker's superior performance, with at least 2.7% gain in average sensitivity when compared to other top 3D/2.5D detectors. Further validation on public lung nodule and prostate tumor detection tasks confirms the generalizability of LN-Tracker as it achieves top performance on both tasks. Datasets will be released upon acceptance.
* Technical report (11 pages plus supplementary)
Via
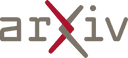
Feb 27, 2025
Abstract:Detection and classification of pulmonary nodules is a challenge in medical image analysis due to the variety of shapes and sizes of nodules and their high concealment. Despite the success of traditional deep learning methods in image classification, deep networks still struggle to perfectly capture subtle changes in lung nodule detection. Therefore, we propose a residual multi-task network (Res-MTNet) model, which combines multi-task learning and residual learning, and improves feature representation ability by sharing feature extraction layer and introducing residual connections. Multi-task learning enables the model to handle multiple tasks simultaneously, while the residual module solves the problem of disappearing gradients, ensuring stable training of deeper networks and facilitating information sharing between tasks. Res-MTNet enhances the robustness and accuracy of the model, providing a more reliable lung nodule analysis tool for clinical medicine and telemedicine.
Via
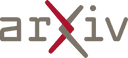
Mar 04, 2025
Abstract:Detecting and segmenting small objects, such as lung nodules and tumor lesions, remains a critical challenge in image analysis. These objects often occupy less than 0.1% of an image, making traditional transformer architectures inefficient and prone to performance degradation due to redundant attention computations on irrelevant regions. Existing sparse attention mechanisms rely on rigid hierarchical structures, which are poorly suited for detecting small, variable, and uncertain object locations. In this paper, we propose BoltzFormer, a novel transformer-based architecture designed to address these challenges through dynamic sparse attention. BoltzFormer identifies and focuses attention on relevant areas by modeling uncertainty using a Boltzmann distribution with an annealing schedule. Initially, a higher temperature allows broader area sampling in early layers, when object location uncertainty is greatest. As the temperature decreases in later layers, attention becomes more focused, enhancing efficiency and accuracy. BoltzFormer seamlessly integrates into existing transformer architectures via a modular Boltzmann attention sampling mechanism. Comprehensive evaluations on benchmark datasets demonstrate that BoltzFormer significantly improves segmentation performance for small objects while reducing attention computation by an order of magnitude compared to previous state-of-the-art methods.
Via
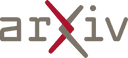
Feb 28, 2025
Abstract:AI models for lung cancer screening are limited by data scarcity, impacting generalizability and clinical applicability. Generative models address this issue but are constrained by training data variability. We introduce SYN-LUNGS, a framework for generating high-quality 3D CT images with detailed annotations. SYN-LUNGS integrates XCAT3 phantoms for digital twin generation, X-Lesions for nodule simulation (varying size, location, and appearance), and DukeSim for CT image formation with vendor and parameter variability. The dataset includes 3,072 nodule images from 1,044 simulated CT scans, with 512 lesions and 174 digital twins. Models trained on clinical + simulated data outperform clinical only models, achieving 10% improvement in detection, 2-9% in segmentation and classification, and enhanced synthesis.By incorporating anatomy-informed simulations, SYN-LUNGS provides a scalable approach for AI model development, particularly in rare disease representation and improving model reliability.
* 6 figures, 12 pages
Via
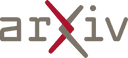