What is speech recognition? Speech recognition is the task of identifying words spoken aloud, analyzing the voice and language, and accurately transcribing the words.
Papers and Code
Jun 12, 2025
Abstract:Speech emotion recognition (SER) in naturalistic conditions presents a significant challenge for the speech processing community. Challenges include disagreement in labeling among annotators and imbalanced data distributions. This paper presents a reproducible framework that achieves superior (top 1) performance in the Emotion Recognition in Naturalistic Conditions Challenge (IS25-SER Challenge) - Task 2, evaluated on the MSP-Podcast dataset. Our system is designed to tackle the aforementioned challenges through multimodal learning, multi-task learning, and imbalanced data handling. Specifically, our best system is trained by adding text embeddings, predicting gender, and including ``Other'' (O) and ``No Agreement'' (X) samples in the training set. Our system's results secured both first and second places in the IS25-SER Challenge, and the top performance was achieved by a simple two-system ensemble.
* Accepeted to Interspeech2025
Via
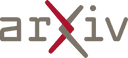
Jun 11, 2025
Abstract:Speech foundation models (SFMs), such as Open Whisper-Style Speech Models (OWSM), are trained on massive datasets to achieve accurate automatic speech recognition. However, even SFMs struggle to accurately recognize rare and unseen words. While contextual biasing (CB) is a promising approach to improve recognition of such words, most CB methods are trained from scratch, resulting in lower performance than SFMs due to the lack of pre-trained knowledge. This paper integrates an existing CB method with OWSM v3.1 while freezing its pre-trained parameters. By leveraging the knowledge embedded in SFMs, the proposed method enables effective CB while preserving the advantages of SFMs, even with a small dataset. Experimental results show that the proposed method improves the biasing word error rate (B-WER) by 11.6 points, resulting in a 0.9 point improvement in the overall WER while reducing the real-time factor by 7.5% compared to the non-biasing baseline on the LibriSpeech 100 test-clean set.
* Accepted to Interspeech 2025
Via
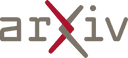
Jun 13, 2025
Abstract:Traditional audiometry often provides an incomplete characterization of the functional impact of hearing loss on speech understanding, particularly for supra-threshold deficits common in presbycusis. This motivates the development of more diagnostically specific speech perception tests. We introduce the Simulated Phoneme Speech Test (SimPhon Speech Test) methodology, a novel, multi-stage computational pipeline for the in silico design and validation of a phonetically balanced minimal-pair speech test. This methodology leverages a modern Automatic Speech Recognition (ASR) system as a proxy for a human listener to simulate the perceptual effects of sensorineural hearing loss. By processing speech stimuli under controlled acoustic degradation, we first identify the most common phoneme confusion patterns. These patterns then guide the data-driven curation of a large set of candidate word pairs derived from a comprehensive linguistic corpus. Subsequent phases involving simulated diagnostic testing, expert human curation, and a final, targeted sensitivity analysis systematically reduce the candidates to a final, optimized set of 25 pairs (the SimPhon Speech Test-25). A key finding is that the diagnostic performance of the SimPhon Speech Test-25 test items shows no significant correlation with predictions from the standard Speech Intelligibility Index (SII), suggesting the SimPhon Speech Test captures perceptual deficits beyond simple audibility. This computationally optimized test set offers a significant increase in efficiency for audiological test development, ready for initial human trials.
Via
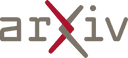
Jun 10, 2025
Abstract:The scarcity of large-scale classroom speech data has hindered the development of AI-driven speech models for education. Public classroom datasets remain limited, and the lack of a dedicated classroom noise corpus prevents the use of standard data augmentation techniques. In this paper, we introduce a scalable methodology for synthesizing classroom noise using game engines, a framework that extends to other domains. Using this methodology, we present SimClass, a dataset that includes both a synthesized classroom noise corpus and a simulated classroom speech dataset. The speech data is generated by pairing a public children's speech corpus with YouTube lecture videos to approximate real classroom interactions in clean conditions. Our experiments on clean and noisy speech demonstrate that SimClass closely approximates real classroom speech, making it a valuable resource for developing robust speech recognition and enhancement models.
Via
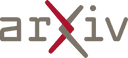
Jun 12, 2025
Abstract:Automatic Speech Recognition systems have made significant progress with large-scale pre-trained models. However, most current systems focus solely on transcribing the speech without identifying speaker roles, a function that is critical for conversational AI. In this work, we investigate the use of serialized output training (SOT) for joint ASR and speaker role tagging. By augmenting Whisper with role-specific tokens and fine-tuning it with SOT, we enable the model to generate role-aware transcriptions in a single decoding pass. We compare the SOT approach against a self-supervised previous baseline method on two real-world conversational datasets. Our findings show that this approach achieves more than 10% reduction in multi-talker WER, demonstrating its feasibility as a unified model for speaker-role aware speech transcription.
* Under review
Via
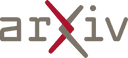
Jun 12, 2025
Abstract:With recent advances in modeling and the increasing amount of supervised training data, automatic speech recognition (ASR) systems have achieved remarkable performance on general speech. However, the word error rate (WER) of state-of-the-art ASR remains high for named entities. Since named entities are often the most critical keywords, misrecognizing them can affect all downstream applications, especially when the ASR system functions as the front end of a complex system. In this paper, we introduce a large language model (LLM) revision mechanism to revise incorrect named entities in ASR predictions by leveraging the LLM's reasoning ability as well as local context (e.g., lecture notes) containing a set of correct named entities. Finally, we introduce the NER-MIT-OpenCourseWare dataset, containing 45 hours of data from MIT courses for development and testing. On this dataset, our proposed technique achieves up to 30\% relative WER reduction for named entities.
Via
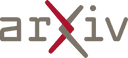
Jun 11, 2025
Abstract:SER is a challenging task due to the subjective nature of human emotions and their uneven representation under naturalistic conditions. We propose MEDUSA, a multimodal framework with a four-stage training pipeline, which effectively handles class imbalance and emotion ambiguity. The first two stages train an ensemble of classifiers that utilize DeepSER, a novel extension of a deep cross-modal transformer fusion mechanism from pretrained self-supervised acoustic and linguistic representations. Manifold MixUp is employed for further regularization. The last two stages optimize a trainable meta-classifier that combines the ensemble predictions. Our training approach incorporates human annotation scores as soft targets, coupled with balanced data sampling and multitask learning. MEDUSA ranked 1st in Task 1: Categorical Emotion Recognition in the Interspeech 2025: Speech Emotion Recognition in Naturalistic Conditions Challenge.
* Accepted at Interspeech 2025
Via
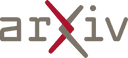
Jun 13, 2025
Abstract:Longform audio recordings obtained with microphones worn by children-also known as child-centered daylong recordings-have become a standard method for studying children's language experiences and their impact on subsequent language development. Transcripts of longform speech audio would enable rich analyses at various linguistic levels, yet the massive scale of typical longform corpora prohibits comprehensive manual annotation. At the same time, automatic speech recognition (ASR)-based transcription faces significant challenges due to the noisy, unconstrained nature of real-world audio, and no existing study has successfully applied ASR to transcribe such data. However, previous attempts have assumed that ASR must process each longform recording in its entirety. In this work, we present an approach to automatically detect those utterances in longform audio that can be reliably transcribed with modern ASR systems, allowing automatic and relatively accurate transcription of a notable proportion of all speech in typical longform data. We validate the approach on four English longform audio corpora, showing that it achieves a median word error rate (WER) of 0% and a mean WER of 18% when transcribing 13% of the total speech in the dataset. In contrast, transcribing all speech without any filtering yields a median WER of 52% and a mean WER of 51%. We also compare word log-frequencies derived from the automatic transcripts with those from manual annotations and show that the frequencies correlate at r = 0.92 (Pearson) for all transcribed words and r = 0.98 for words that appear at least five times in the automatic transcripts. Overall, the work provides a concrete step toward increasingly detailed automated linguistic analyses of child-centered longform audio.
* pre-print
Via
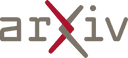
Jun 13, 2025
Abstract:Deep neural networks (DNNs) have achieved remarkable success across diverse domains, but their performance can be severely degraded by noisy or corrupted training data. Conventional noise mitigation methods often rely on explicit assumptions about noise distributions or require extensive retraining, which can be impractical for large-scale models. Inspired by the principles of machine unlearning, we propose a novel framework that integrates attribution-guided data partitioning, discriminative neuron pruning, and targeted fine-tuning to mitigate the impact of noisy samples. Our approach employs gradient-based attribution to probabilistically distinguish high-quality examples from potentially corrupted ones without imposing restrictive assumptions on the noise. It then applies regression-based sensitivity analysis to identify and prune neurons that are most vulnerable to noise. Finally, the resulting network is fine-tuned on the high-quality data subset to efficiently recover and enhance its generalization performance. This integrated unlearning-inspired framework provides several advantages over conventional noise-robust learning approaches. Notably, it combines data-level unlearning with model-level adaptation, thereby avoiding the need for full model retraining or explicit noise modeling. We evaluate our method on representative tasks (e.g., CIFAR-10 image classification and speech recognition) under various noise levels and observe substantial gains in both accuracy and efficiency. For example, our framework achieves approximately a 10% absolute accuracy improvement over standard retraining on CIFAR-10 with injected label noise, while reducing retraining time by up to 47% in some settings. These results demonstrate the effectiveness and scalability of the proposed approach for achieving robust generalization in noisy environments.
Via
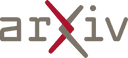
Jun 09, 2025
Abstract:The smart home systems, based on AI speech recognition and IoT technology, enable people to control devices through verbal commands and make people's lives more efficient. However, existing AI speech recognition services are primarily deployed on cloud platforms on the Internet. When users issue a command, speech recognition devices like ``Amazon Echo'' will post a recording through numerous network nodes, reach multiple servers, and then receive responses through the Internet. This mechanism presents several issues, including unnecessary energy consumption, communication latency, and the risk of a single-point failure. In this position paper, we propose a smart home concept based on offline speech recognition and IoT technology: 1) integrating offline keyword spotting (KWS) technologies into household appliances with limited resource hardware to enable them to understand user voice commands; 2) designing a local IoT network with decentralized architecture to manage and connect various devices, enhancing the robustness and scalability of the system. This proposal of a smart home based on offline speech recognition and IoT technology will allow users to use low-latency voice control anywhere in the home without depending on the Internet and provide better scalability and energy sustainability.
Via
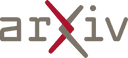