What is facial recognition? Facial recognition is an AI-based technique for identifying or confirming an individual's identity using their face. It maps facial features from an image or video and then compares the information with a collection of known faces to find a match.
Papers and Code
Jan 16, 2025
Abstract:Vision Transformers (ViTs) are increasingly being adopted in various sensitive vision applications - like medical diagnosis, facial recognition, etc. To improve the interpretability of such models, many approaches attempt to forward-align them with carefully annotated abstract, human-understandable semantic entities - concepts. Concepts provide global rationales to the model predictions and can be quickly understood/intervened on by domain experts. Most current research focuses on designing model-agnostic, plug-and-play generic concept-based explainability modules that do not incorporate the inner workings of foundation models (e.g., inductive biases, scale invariance, etc.) during training. To alleviate this issue for ViTs, in this paper, we propose a novel Concept Representation Alignment Module (CRAM) which learns both scale and position-aware representations from multi-scale feature pyramids and patch representations respectively. CRAM further aligns these representations with concept annotations through an attention matrix. The proposed CRAM module improves the predictive performance of ViT architectures and also provides accurate and robust concept explanations as demonstrated on five datasets - including three widely used benchmarks (CUB, Pascal APY, Concept-MNIST) and 2 real-world datasets (AWA2, KITS).
Via
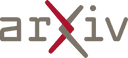
Feb 05, 2025
Abstract:Autonomous driving technology has advanced significantly, yet detecting driving anomalies remains a major challenge due to the long-tailed distribution of driving events. Existing methods primarily rely on single-modal road condition video data, which limits their ability to capture rare and unpredictable driving incidents. This paper proposes a multimodal driver assistance detection system that integrates road condition video, driver facial video, and audio data to enhance incident recognition accuracy. Our model employs an attention-based intermediate fusion strategy, enabling end-to-end learning without separate feature extraction. To support this approach, we develop a new three-modality dataset using a driving simulator. Experimental results demonstrate that our method effectively captures cross-modal correlations, reducing misjudgments and improving driving safety.
Via
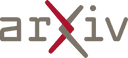
Jan 03, 2025
Abstract:Most facial expression recognition (FER) models are trained on large-scale expression data with centralized learning. Unfortunately, collecting a large amount of centralized expression data is difficult in practice due to privacy concerns of facial images. In this paper, we investigate FER under the framework of personalized federated learning, which is a valuable and practical decentralized setting for real-world applications. To this end, we develop a novel uncertainty-Aware label refineMent on hYpergraphs (AMY) method. For local training, each local model consists of a backbone, an uncertainty estimation (UE) block, and an expression classification (EC) block. In the UE block, we leverage a hypergraph to model complex high-order relationships between expression samples and incorporate these relationships into uncertainty features. A personalized uncertainty estimator is then introduced to estimate reliable uncertainty weights of samples in the local client. In the EC block, we perform label propagation on the hypergraph, obtaining high-quality refined labels for retraining an expression classifier. Based on the above, we effectively alleviate heterogeneous sample uncertainty across clients and learn a robust personalized FER model in each client. Experimental results on two challenging real-world facial expression databases show that our proposed method consistently outperforms several state-of-the-art methods. This indicates the superiority of hypergraph modeling for uncertainty estimation and label refinement on the personalized federated FER task. The source code will be released at https://github.com/mobei1006/AMY.
* IEEE Transactions on Circuits and Systems for Video Technology,
2024
Via
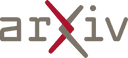
Mar 04, 2025
Abstract:This paper proposes FABG (Facial Affective Behavior Generation), an end-to-end imitation learning system for human-robot interaction, designed to generate natural and fluid facial affective behaviors. In interaction, effectively obtaining high-quality demonstrations remains a challenge. In this work, we develop an immersive virtual reality (VR) demonstration system that allows operators to perceive stereoscopic environments. This system ensures "the operator's visual perception matches the robot's sensory input" and "the operator's actions directly determine the robot's behaviors" - as if the operator replaces the robot in human interaction engagements. We propose a prediction-driven latency compensation strategy to reduce robotic reaction delays and enhance interaction fluency. FABG naturally acquires human interactive behaviors and subconscious motions driven by intuition, eliminating manual behavior scripting. We deploy FABG on a real-world 25-degree-of-freedom (DoF) humanoid robot, validating its effectiveness through four fundamental interaction tasks: expression response, dynamic gaze, foveated attention, and gesture recognition, supported by data collection and policy training. Project website: https://cybergenies.github.io
Via
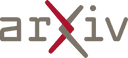
Jan 13, 2025
Abstract:The integration of dialogue interfaces in mobile devices has become ubiquitous, providing a wide array of services. As technology progresses, humanoid robots designed with human-like features to interact effectively with people are gaining prominence, and the use of advanced human-robot dialogue interfaces is continually expanding. In this context, emotion recognition plays a crucial role in enhancing human-robot interaction by enabling robots to understand human intentions. This research proposes a facial emotion detection interface integrated into a mobile humanoid robot, capable of displaying real-time emotions from multiple individuals on a user interface. To this end, various deep neural network models for facial expression recognition were developed and evaluated under consistent computer-based conditions, yielding promising results. Afterwards, a trade-off between accuracy and memory footprint was carefully considered to effectively implement this application on a mobile humanoid robot.
* 9 pages, 8 figures and 1 table. Accepted at the 17th International
Conference on Agents and Artificial Intelligence (ICAART 2025), Porto,
Portugal
Via
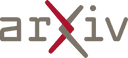
Dec 25, 2024
Abstract:This paper expands the cascaded network branch of the autoencoder-based multi-task learning (MTL) framework for dynamic facial expression recognition, namely Multi-Task Cascaded Autoencoder for Dynamic Facial Expression Recognition (MTCAE-DFER). MTCAE-DFER builds a plug-and-play cascaded decoder module, which is based on the Vision Transformer (ViT) architecture and employs the decoder concept of Transformer to reconstruct the multi-head attention module. The decoder output from the previous task serves as the query (Q), representing local dynamic features, while the Video Masked Autoencoder (VideoMAE) shared encoder output acts as both the key (K) and value (V), representing global dynamic features. This setup facilitates interaction between global and local dynamic features across related tasks. Additionally, this proposal aims to alleviate overfitting of complex large model. We utilize autoencoder-based multi-task cascaded learning approach to explore the impact of dynamic face detection and dynamic face landmark on dynamic facial expression recognition, which enhances the model's generalization ability. After we conduct extensive ablation experiments and comparison with state-of-the-art (SOTA) methods on various public datasets for dynamic facial expression recognition, the robustness of the MTCAE-DFER model and the effectiveness of global-local dynamic feature interaction among related tasks have been proven.
Via
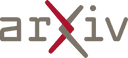
Dec 11, 2024
Abstract:Facial recognition technology has made significant advances, yet its effectiveness across diverse ethnic backgrounds, particularly in specific Indian demographics, is less explored. This paper presents a detailed evaluation of both traditional and deep learning-based facial recognition models using the established LFW dataset and our newly developed IITJ Faces of Academia Dataset (JFAD), which comprises images of students from IIT Jodhpur. This unique dataset is designed to reflect the ethnic diversity of India, providing a critical test bed for assessing model performance in a focused academic environment. We analyze models ranging from holistic approaches like Eigenfaces and SIFT to advanced hybrid models that integrate CNNs with Gabor filters, Laplacian transforms, and segmentation techniques. Our findings reveal significant insights into the models' ability to adapt to the ethnic variability within Indian demographics and suggest modifications to enhance accuracy and inclusivity in real-world applications. The JFAD not only serves as a valuable resource for further research but also highlights the need for developing facial recognition systems that perform equitably across diverse populations.
* Research Project - Computer Vision
Via
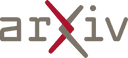
Jan 28, 2025
Abstract:Face recognition (FR) models are vulnerable to performance variations across demographic groups. The causes for these performance differences are unclear due to the highly complex deep learning-based structure of face recognition models. Several works aimed at exploring possible roots of gender and ethnicity bias, identifying semantic reasons such as hairstyle, make-up, or facial hair as possible sources. Motivated by recent discoveries of the importance of frequency patterns in convolutional neural networks, we explain bias in face recognition using state-of-the-art frequency-based explanations. Our extensive results show that different frequencies are important to FR models depending on the ethnicity of the samples.
* Accepted at xAI4Biometrics at ECCV 2024
Via
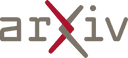
Jan 03, 2025
Abstract:Face Anti-Spoofing (FAS) is essential for ensuring the security and reliability of facial recognition systems. Most existing FAS methods are formulated as binary classification tasks, providing confidence scores without interpretation. They exhibit limited generalization in out-of-domain scenarios, such as new environments or unseen spoofing types. In this work, we introduce a multimodal large language model (MLLM) framework for FAS, termed Interpretable Face Anti-Spoofing (I-FAS), which transforms the FAS task into an interpretable visual question answering (VQA) paradigm. Specifically, we propose a Spoof-aware Captioning and Filtering (SCF) strategy to generate high-quality captions for FAS images, enriching the model's supervision with natural language interpretations. To mitigate the impact of noisy captions during training, we develop a Lopsided Language Model (L-LM) loss function that separates loss calculations for judgment and interpretation, prioritizing the optimization of the former. Furthermore, to enhance the model's perception of global visual features, we design a Globally Aware Connector (GAC) to align multi-level visual representations with the language model. Extensive experiments on standard and newly devised One to Eleven cross-domain benchmarks, comprising 12 public datasets, demonstrate that our method significantly outperforms state-of-the-art methods.
* Accepted to AAAI2025
Via
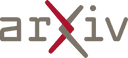
Dec 23, 2024
Abstract:Traditional psychological evaluations rely heavily on human observation and interpretation, which are prone to subjectivity, bias, fatigue, and inconsistency. To address these limitations, this work presents a multimodal emotion recognition system that provides a standardised, objective, and data-driven tool to support evaluators, such as psychologists, psychiatrists, and clinicians. The system integrates recognition of facial expressions, speech, spoken language, and body movement analysis to capture subtle emotional cues that are often overlooked in human evaluations. By combining these modalities, the system provides more robust and comprehensive emotional state assessment, reducing the risk of mis- and overdiagnosis. Preliminary testing in a simulated real-world condition demonstrates the system's potential to provide reliable emotional insights to improve the diagnostic accuracy. This work highlights the promise of automated multimodal analysis as a valuable complement to traditional psychological evaluation practices, with applications in clinical and therapeutic settings.
* 10 pages, 6 figures, 3 tables
Via
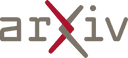