May 14, 2025
Abstract:Since 2022, versions of generative AI chatbots such as ChatGPT and Claude have been trained using a specialized technique called Reinforcement Learning from Human Feedback (RLHF) to fine-tune language model output using feedback from human annotators. As a result, the integration of RLHF has greatly enhanced the outputs of these large language models (LLMs) and made the interactions and responses appear more "human-like" than those of previous versions using only supervised learning. The increasing convergence of human and machine-written text has potentially severe ethical, sociotechnical, and pedagogical implications relating to transparency, trust, bias, and interpersonal relations. To highlight these implications, this paper presents a rhetorical analysis of some of the central procedures and processes currently being reshaped by RLHF-enhanced generative AI chatbots: upholding language conventions, information seeking practices, and expectations for social relationships. Rhetorical investigations of generative AI and LLMs have, to this point, focused largely on the persuasiveness of the content generated. Using Ian Bogost's concept of procedural rhetoric, this paper shifts the site of rhetorical investigation from content analysis to the underlying mechanisms of persuasion built into RLHF-enhanced LLMs. In doing so, this theoretical investigation opens a new direction for further inquiry in AI ethics that considers how procedures rerouted through AI-driven technologies might reinforce hegemonic language use, perpetuate biases, decontextualize learning, and encroach upon human relationships. It will therefore be of interest to educators, researchers, scholars, and the growing number of users of generative AI chatbots.
* 10 pages, 1 figure, Accepted version
Via
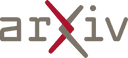