Feb 17, 2025
Abstract:How we should design and interact with social artificial intelligence depends on the socio-relational role the AI is meant to emulate or occupy. In human society, relationships such as teacher-student, parent-child, neighbors, siblings, or employer-employee are governed by specific norms that prescribe or proscribe cooperative functions including hierarchy, care, transaction, and mating. These norms shape our judgments of what is appropriate for each partner. For example, workplace norms may allow a boss to give orders to an employee, but not vice versa, reflecting hierarchical and transactional expectations. As AI agents and chatbots powered by large language models are increasingly designed to serve roles analogous to human positions - such as assistant, mental health provider, tutor, or romantic partner - it is imperative to examine whether and how human relational norms should extend to human-AI interactions. Our analysis explores how differences between AI systems and humans, such as the absence of conscious experience and immunity to fatigue, may affect an AI's capacity to fulfill relationship-specific functions and adhere to corresponding norms. This analysis, which is a collaborative effort by philosophers, psychologists, relationship scientists, ethicists, legal experts, and AI researchers, carries important implications for AI systems design, user behavior, and regulation. While we accept that AI systems can offer significant benefits such as increased availability and consistency in certain socio-relational roles, they also risk fostering unhealthy dependencies or unrealistic expectations that could spill over into human-human relationships. We propose that understanding and thoughtfully shaping (or implementing) suitable human-AI relational norms will be crucial for ensuring that human-AI interactions are ethical, trustworthy, and favorable to human well-being.
* 76 pages, 2 figures
Via
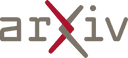