What is autonomous cars? Autonomous cars are self-driving vehicles that use artificial intelligence (AI) and sensors to navigate and operate without human intervention, using high-resolution cameras and lidars that detect what happens in the car's immediate surroundings. They have the potential to revolutionize transportation by improving safety, efficiency, and accessibility.
Papers and Code
Dec 04, 2024
Abstract:Considerable study has already been conducted regarding autonomous driving in modern era. An autonomous driving system must be extremely good at detecting objects surrounding the car to ensure safety. In this paper, classification, and estimation of an object's (pedestrian) position (concerning an ego 3D coordinate system) are studied and the distance between the ego vehicle and the object in the context of autonomous driving is measured. To classify the object, faster Region-based Convolution Neural Network (R-CNN) with inception v2 is utilized. First, a network is trained with customized dataset to estimate the reference position of objects as well as the distance from the vehicle. From camera calibration to computing the distance, cutting-edge technologies of computer vision algorithms in a series of processes are applied to generate a 3D reference point of the region of interest. The foremost step in this process is generating a disparity map using the concept of stereo vision.
Via
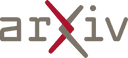
Nov 20, 2024
Abstract:Autonomous racing is gaining attention for its potential to advance autonomous vehicle technologies. Accurate race car dynamics modeling is essential for capturing and predicting future states like position, orientation, and velocity. However, accurately modeling complex subsystems such as tires and suspension poses significant challenges. In this paper, we introduce the Deep Kernel-based Multi-task Gaussian Process (DKMGP), which leverages the structure of a variational multi-task and multi-step Gaussian process model enhanced with deep kernel learning for vehicle dynamics modeling. Unlike existing single-step methods, DKMGP performs multi-step corrections with an adaptive correction horizon (ACH) algorithm that dynamically adjusts to varying driving conditions. To validate and evaluate the proposed DKMGP method, we compare the model performance with DKL-SKIP and a well-tuned single-track model, using high-speed dynamics data (exceeding 230kmph) collected from a full-scale Indy race car during the Indy Autonomous Challenge held at the Las Vegas Motor Speedway at CES 2024. The results demonstrate that DKMGP achieves upto 99% prediction accuracy compared to one-step DKL-SKIP, while improving real-time computational efficiency by 1752x. Our results show that DKMGP is a scalable and efficient solution for vehicle dynamics modeling making it suitable for high-speed autonomous racing control.
* 13 pages, 6 figures, 4 tables; submitted to 7th Annual Learning for
Dynamics & Control Conference
Via
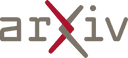
Oct 28, 2024
Abstract:The skill to drift a car--i.e., operate in a state of controlled oversteer like professional drivers--could give future autonomous cars maximum flexibility when they need to retain control in adverse conditions or avoid collisions. We investigate real-time drifting strategies that put the car where needed while bypassing expensive trajectory optimization. To this end, we design a reinforcement learning agent that builds on the concept of tire energy absorption to autonomously drift through changing and complex waypoint configurations while safely staying within track bounds. We achieve zero-shot deployment on the car by training the agent in a simulation environment built on top of a neural stochastic differential equation vehicle model learned from pre-collected driving data. Experiments on a Toyota GR Supra and Lexus LC 500 show that the agent is capable of drifting smoothly through varying waypoint configurations with tracking error as low as 10 cm while stably pushing the vehicles to sideslip angles of up to 63{\deg}.
* Initial submission to ICRA 2025
Via
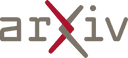
Nov 23, 2024
Abstract:Vehicle trajectory prediction is crucial for advancing autonomous driving and advanced driver assistance systems (ADAS). Although deep learning-based approaches - especially those utilizing transformer-based and generative models - have markedly improved prediction accuracy by capturing complex, non-linear patterns in vehicle dynamics and traffic interactions, they frequently overlook detailed car-following behaviors and the inter-vehicle interactions critical for real-world driving applications, particularly in fully autonomous or mixed traffic scenarios. To address the issue, this study introduces a scaled noise conditional diffusion model for car-following trajectory prediction, which integrates detailed inter-vehicular interactions and car-following dynamics into a generative framework, improving both the accuracy and plausibility of predicted trajectories. The model utilizes a novel pipeline to capture historical vehicle dynamics by scaling noise with encoded historical features within the diffusion process. Particularly, it employs a cross-attention-based transformer architecture to model intricate inter-vehicle dependencies, effectively guiding the denoising process and enhancing prediction accuracy. Experimental results on diverse real-world driving scenarios demonstrate the state-of-the-art performance and robustness of the proposed method.
* arXiv admin note: text overlap with arXiv:2406.11941
Via
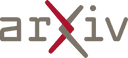
Dec 29, 2024
Abstract:Autonomous vehicles face significant challenges in navigating adverse weather, particularly rain, due to the visual impairment of camera-based systems. In this study, we leveraged contemporary deep learning techniques to mitigate these challenges, aiming to develop a vision model that processes live vehicle camera feeds to eliminate rain-induced visual hindrances, yielding visuals closely resembling clear, rain-free scenes. Using the Car Learning to Act (CARLA) simulation environment, we generated a comprehensive dataset of clear and rainy images for model training and testing. In our model, we employed a classic encoder-decoder architecture with skip connections and concatenation operations. It was trained using novel batching schemes designed to effectively distinguish high-frequency rain patterns from low-frequency scene features across successive image frames. To evaluate the model performance, we integrated it with a steering module that processes front-view images as input. The results demonstrated notable improvements in steering accuracy, underscoring the model's potential to enhance navigation safety and reliability in rainy weather conditions.
* 16 pages, 16 figures, 2 tables
Via
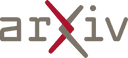
Oct 23, 2024
Abstract:Simulators are indispensable for research in autonomous systems such as self-driving cars, autonomous robots and drones. Despite significant progress in various simulation aspects, such as graphical realism, an evident gap persists between the virtual and real-world environments. Since the ultimate goal is to deploy the autonomous systems in the real world, closing the sim2real gap is of utmost importance. In this paper, we employ a state-ofthe-art approach to enhance the photorealism of simulated data, aligning them with the visual characteristics of real-world datasets. Based on this, we developed CARLA2Real, an easy-to-use, publicly available tool (plug-in) for the widely used and open-source CARLA simulator. This tool enhances the output of CARLA in near realtime, achieving a frame rate of 13 FPS, translating it to the visual style and realism of real-world datasets such as Cityscapes, KITTI, and Mapillary Vistas. By employing the proposed tool, we generated synthetic datasets from both the simulator and the enhancement model outputs, including their corresponding ground truth annotations for tasks related to autonomous driving. Then, we performed a number of experiments to evaluate the impact of the proposed approach on feature extraction and semantic segmentation methods when trained on the enhanced synthetic data. The results demonstrate that the sim2real gap is significant and can indeed be reduced by the introduced approach.
* 22 pages
Via
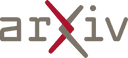
Dec 06, 2024
Abstract:As the Computer Vision community rapidly develops and advances algorithms for autonomous driving systems, the goal of safer and more efficient autonomous transportation is becoming increasingly achievable. However, it is 2024, and we still do not have fully self-driving cars. One of the remaining core challenges lies in addressing the novelty problem, where self-driving systems still struggle to handle previously unseen situations on the open road. With our Challenge of Out-Of-Label (COOOL) benchmark, we introduce a novel dataset for hazard detection, offering versatile evaluation metrics applicable across various tasks, including novelty-adjacent domains such as Anomaly Detection, Open-Set Recognition, Open Vocabulary, and Domain Adaptation. COOOL comprises over 200 collections of dashcam-oriented videos, annotated by human labelers to identify objects of interest and potential driving hazards. It includes a diverse range of hazards and nuisance objects. Due to the dataset's size and data complexity, COOOL serves exclusively as an evaluation benchmark.
Via
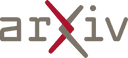
Dec 12, 2024
Abstract:The joint use of event-based vision and Spiking Neural Networks (SNNs) is expected to have a large impact in robotics in the near future, in tasks such as, visual odometry and obstacle avoidance. While researchers have used real-world event datasets for optical flow prediction (mostly captured with Unmanned Aerial Vehicles (UAVs)), these datasets are limited in diversity, scalability, and are challenging to collect. Thus, synthetic datasets offer a scalable alternative by bridging the gap between reality and simulation. In this work, we address the lack of datasets by introducing eWiz, a comprehensive library for processing event-based data. It includes tools for data loading, augmentation, visualization, encoding, and generation of training data, along with loss functions and performance metrics. We further present a synthetic event-based datasets and data generation pipelines for optical flow prediction tasks. Built on top of eWiz, eCARLA-scenes makes use of the CARLA simulator to simulate self-driving car scenarios. The ultimate goal of this dataset is the depiction of diverse environments while laying a foundation for advancing event-based camera applications in autonomous field vehicle navigation, paving the way for using SNNs on neuromorphic hardware such as the Intel Loihi.
Via
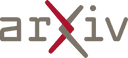
Nov 08, 2024
Abstract:As we move towards a mixed-traffic scenario of Autonomous vehicles (AVs) and Human-driven vehicles (HDVs), understanding the car-following behaviour is important to improve traffic efficiency and road safety. Using a real-world trajectory dataset, this study uses descriptive and statistical analysis to investigate the car-following behaviours of three vehicle pairs: HDV-AV, AV-HDV and HDV-HDV in mixed traffic. The ANOVA test showed that car-following behaviours across different vehicle pairs are statistically significant (p-value < 0.05). We also introduce a data-driven Knowledge Distillation Neural Network (KDNN) model for predicting car-following behaviour in terms of speed. The KDNN model demonstrates comparable predictive accuracy to its teacher network, a Long Short-Term Memory (LSTM) network, and outperforms both the standalone student network, a Multilayer Perceptron (MLP), and traditional physics-based models like the Gipps model. Notably, the KDNN model better prevents collisions, measured by minimum Time-to-Collision (TTC), and operates with lower computational power, making it ideal for AVs or driving simulators requiring efficient computing.
* 27th IEEE International Conference on Intelligent Transportation
Systems
Via
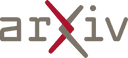
Dec 31, 2024
Abstract:The key to ensuring the safe obstacle avoidance function of autonomous driving systems lies in the use of extremely accurate vehicle recognition techniques. However, the variability of the actual road environment and the diverse characteristics of vehicles and pedestrians together constitute a huge obstacle to improving detection accuracy, posing a serious challenge to the realization of this goal. To address the above issues, this paper proposes an improved YOLOv8 vehicle detection method. Specifically, taking the YOLOv8n-seg model as the base model, firstly, the FasterNet network is used to replace the backbone network to achieve the purpose of reducing the computational complexity and memory while improving the detection accuracy and speed; secondly, the feature enhancement is achieved by adding the attention mechanism CBAM to the Neck; and lastly, the loss function CIoU is modified to WIoU, which optimizes the detection box localization while improving the segmentation accuracy. The results show that the improved model achieves 98.3%, 89.1% and 88.4% detection accuracy for car, Person and Motorcycle. Compared with the pre-improvement and YOLOv9 models in six metrics such as Precision.
Via
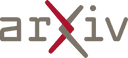