What is Recommendation? Recommendation is the task of providing personalized suggestions to users based on their preferences and behavior.
Papers and Code
Jun 12, 2025
Abstract:Multimodal Large Language Models (MLLMs) have demonstrated remarkable capabilities in representing and understanding diverse modalities. However, they typically focus on modality alignment in a pairwise manner while overlooking structural relationships across data points. Integrating multimodality with structured graph information (i.e., multimodal graphs, MMGs) is essential for real-world applications such as social networks, healthcare, and recommendation systems. Existing MMG learning methods fall into three paradigms based on how they leverage MLLMs: Encoder, Aligner, and Predictor. MLLM-as-Encoder focuses on enhancing graph neural networks (GNNs) via multimodal feature fusion; MLLM-as-Aligner aligns multimodal attributes in language or hidden space to enable LLM-based graph reasoning; MLLM-as-Predictor treats MLLMs as standalone reasoners with in-context learning or fine-tuning. Despite their advances, the MMG field lacks a unified benchmark to fairly evaluate across these approaches, making it unclear what progress has been made. To bridge this gap, we present Graph-MLLM, a comprehensive benchmark for multimodal graph learning by systematically evaluating these three paradigms across six datasets with different domains. Through extensive experiments, we observe that jointly considering the visual and textual attributes of the nodes benefits graph learning, even when using pre-trained text-to-image alignment models (e.g., CLIP) as encoders. We also find that converting visual attributes into textual descriptions further improves performance compared to directly using visual inputs. Moreover, we observe that fine-tuning MLLMs on specific MMGs can achieve state-of-the-art results in most scenarios, even without explicit graph structure information. We hope that our open-sourced library will facilitate rapid, equitable evaluation and inspire further innovative research in this field.
* 16 pages, 4 figures
Via
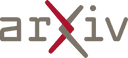
Jun 12, 2025
Abstract:AutomationML (AML) enables standardized data exchange in engineering, yet existing recommendations for proper AML modeling are typically formulated as informal and textual constraints. These constraints cannot be validated automatically within AML itself. This work-in-progress paper introduces a pipeline to formalize and verify such constraints. First, AML models are mapped to OWL ontologies via RML and SPARQL. In addition, a Large Language Model translates textual rules into SHACL constraints, which are then validated against the previously generated AML ontology. Finally, SHACL validation results are automatically interpreted in natural language. The approach is demonstrated on a sample AML recommendation. Results show that even complex modeling rules can be semi-automatically checked -- without requiring users to understand formal methods or ontology technologies.
Via
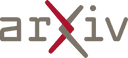
Jun 12, 2025
Abstract:Matrix completion is a widely adopted framework in recommender systems, as predicting the missing entries in the user-item rating matrix enables a comprehensive understanding of user preferences. However, current graph neural network (GNN)-based approaches are highly sensitive to noisy or irrelevant edges--due to their inherent message-passing mechanisms--and are prone to overfitting, which limits their generalizability. To overcome these challenges, we propose a novel method called Matrix Completion using Contrastive Learning (MCCL). Our approach begins by extracting local neighborhood subgraphs for each interaction and subsequently generates two distinct graph representations. The first representation emphasizes denoising by integrating GNN layers with an attention mechanism, while the second is obtained via a graph variational autoencoder that aligns the feature distribution with a standard prior. A mutual learning loss function is employed during training to gradually harmonize these representations, enabling the model to capture common patterns and significantly enhance its generalizability. Extensive experiments on several real-world datasets demonstrate that our approach not only improves the numerical accuracy of the predicted scores--achieving up to a 0.8% improvement in RMSE--but also produces superior rankings with improvements of up to 36% in ranking metrics.
* 30 pages
Via
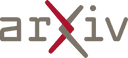
Jun 12, 2025
Abstract:This is a course project report with complete methodology, experiments, references and mathematical derivations. Matrix factorization [1] is a widely used technique in recommendation systems. Probabilistic Matrix Factorization (PMF) [2] extends traditional matrix factorization by incorporating probability distributions over latent factors, allowing for uncertainty quantification. However, computing the posterior distribution is intractable due to the high-dimensional integral. To address this, we employ two Bayesian inference methods: Markov Chain Monte Carlo (MCMC) [3, 4] and Variational Inference (VI) [5, 6] to approximate the posterior. We evaluate their performance on MovieLens dataset [7] and compare their convergence speed, predictive accuracy, and computational efficiency. Experimental results demonstrate that VI offers faster convergence, while MCMC provides more accurate posterior estimates.
* 11 pages, 2 figures. This document is a course project report. Some
derivations are presented in a simplified form. For more detailed discussions
and comprehensive proofs, please refer to the references cited in this
report. v2 replacement: we have modified the title to better match our
content. We have also updated the references to be more complete, including
the link to our code
Via
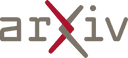
Jun 12, 2025
Abstract:Graph-based multi-task learning at billion-scale presents a significant challenge, as different tasks correspond to distinct billion-scale graphs. Traditional multi-task learning methods often neglect these graph structures, relying solely on individual user and item embeddings. However, disregarding graph structures overlooks substantial potential for improving performance. In this paper, we introduce the Macro Graph of Expert (MGOE) framework, the first approach capable of leveraging macro graph embeddings to capture task-specific macro features while modeling the correlations between task-specific experts. Specifically, we propose the concept of a Macro Graph Bottom, which, for the first time, enables multi-task learning models to incorporate graph information effectively. We design the Macro Prediction Tower to dynamically integrate macro knowledge across tasks. MGOE has been deployed at scale, powering multi-task learning for the homepage of a leading billion-scale recommender system. Extensive offline experiments conducted on three public benchmark datasets demonstrate its superiority over state-of-the-art multi-task learning methods, establishing MGOE as a breakthrough in multi-task graph-based recommendation. Furthermore, online A/B tests confirm the superiority of MGOE in billion-scale recommender systems.
Via
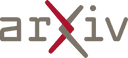
Jun 12, 2025
Abstract:Explaining why the species lives at a particular location is important for understanding ecological systems and conserving biodiversity. However, existing ecological workflows are fragmented and often inaccessible to non-specialists. We propose an end-to-end visual-to-causal framework that transforms a species image into interpretable causal insights about its habitat preference. The system integrates species recognition, global occurrence retrieval, pseudo-absence sampling, and climate data extraction. We then discover causal structures among environmental features and estimate their influence on species occurrence using modern causal inference methods. Finally, we generate statistically grounded, human-readable causal explanations from structured templates and large language models. We demonstrate the framework on a bee and a flower species and report early results as part of an ongoing project, showing the potential of the multimodal AI assistant backed up by a recommended ecological modeling practice for describing species habitat in human-understandable language.
Via
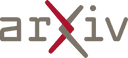
Jun 12, 2025
Abstract:Human-like AI agents such as robots and chatbots are becoming increasingly popular, but they present a variety of ethical concerns. The first concern is in how we define humanness, and how our definition impacts communities historically dehumanized by scientific research. Autistic people in particular have been dehumanized by being compared to robots, making it even more important to ensure this marginalization is not reproduced by AI that may promote neuronormative social behaviors. Second, the ubiquitous use of these agents raises concerns surrounding model biases and accessibility. In our work, we investigate the experiences of the people who build and design these technologies to gain insights into their understanding and acceptance of neurodivergence, and the challenges in making their work more accessible to users with diverse needs. Even though neurodivergent individuals are often marginalized for their unique communication styles, nearly all participants overlooked the conclusions their end-users and other AI system makers may draw about communication norms from the implementation and interpretation of humanness applied in participants' work. This highlights a major gap in their broader ethical considerations, compounded by some participants' neuronormative assumptions about the behaviors and traits that distinguish "humans" from "bots" and the replication of these assumptions in their work. We examine the impact this may have on autism inclusion in society and provide recommendations for additional systemic changes towards more ethical research directions.
* In The 2025 ACM Conference on Fairness, Accountability, and
Transparency (FAccT '25), June 23--26, 2025, Athens, Greece
* published at FAccT 2025, 15 pages, 2 tables, 4 figures
Via
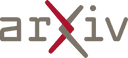
Jun 11, 2025
Abstract:This paper presents a comparison of the performance of Convolutional Neural Networks (CNNs) and Vision Transformers (ViTs) in the task of multi-classifying images containing lesions of psoriasis and diseases similar to it. Models pre-trained on ImageNet were adapted to a specific data set. Both achieved high predictive metrics, but the ViTs stood out for their superior performance with smaller models. Dual Attention Vision Transformer-Base (DaViT-B) obtained the best results, with an f1-score of 96.4%, and is recommended as the most efficient architecture for automated psoriasis detection. This article reinforces the potential of ViTs for medical image classification tasks.
* 12 pages, in Portuguese language, 2 figures, 2 tables, and 4
formulas. To be published in the Proceedings of the LII Brazilian Integrated
Software and Hardware Seminar 2025 (SEMISH 2025)
Via
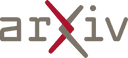
Jun 11, 2025
Abstract:Abdominal aortic aneurysms (AAAs) are progressive focal dilatations of the abdominal aorta. AAAs may rupture, with a survival rate of only 20\%. Current clinical guidelines recommend elective surgical repair when the maximum AAA diameter exceeds 55 mm in men or 50 mm in women. Patients that do not meet these criteria are periodically monitored, with surveillance intervals based on the maximum AAA diameter. However, this diameter does not take into account the complex relation between the 3D AAA shape and its growth, making standardized intervals potentially unfit. Personalized AAA growth predictions could improve monitoring strategies. We propose to use an SE(3)-symmetric transformer model to predict AAA growth directly on the vascular model surface enriched with local, multi-physical features. In contrast to other works which have parameterized the AAA shape, this representation preserves the vascular surface's anatomical structure and geometric fidelity. We train our model using a longitudinal dataset of 113 computed tomography angiography (CTA) scans of 24 AAA patients at irregularly sampled intervals. After training, our model predicts AAA growth to the next scan moment with a median diameter error of 1.18 mm. We further demonstrate our model's utility to identify whether a patient will become eligible for elective repair within two years (acc = 0.93). Finally, we evaluate our model's generalization on an external validation set consisting of 25 CTAs from 7 AAA patients from a different hospital. Our results show that local directional AAA growth prediction from the vascular surface is feasible and may contribute to personalized surveillance strategies.
Via
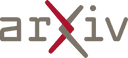
Jun 11, 2025
Abstract:Matrix factorization is a widely used technique in recommendation systems. Probabilistic Matrix Factorization (PMF) [1] extends traditional matrix factorization by incorporating probability distributions over latent factors, allowing for uncertainty quantification. However, computing the posterior distribution is intractable due to the high-dimensional integral. To address this, we employ two Bayesian inference methods: Markov Chain Monte Carlo (MCMC) [2] and Variational Inference (VI) [3] to approximate the posterior. We evaluate their performance on MovieLens dataset and compare their convergence speed, predictive accuracy, and computational efficiency. Experimental results demonstrate that VI offers faster convergence, while MCMC provides more accurate posterior estimates.
* 11 pages, 4 figures
Via
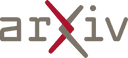