Topic:Text Classification
What is Text Classification? Text classification is the process of categorizing text documents into predefined categories or labels.
Papers and Code
Jun 09, 2025
Abstract:Natural Language Processing (NLP) models are used for text-related tasks such as classification and generation. To complete these tasks, input data is first tokenized from human-readable text into a format the model can understand, enabling it to make inferences and understand context. Text classification models can be implemented to guard against threats such as prompt injection attacks against Large Language Models (LLMs), toxic input and cybersecurity risks such as spam emails. In this paper, we introduce TokenBreak: a novel attack that can bypass these protection models by taking advantage of the tokenization strategy they use. This attack technique manipulates input text in such a way that certain models give an incorrect classification. Importantly, the end target (LLM or email recipient) can still understand and respond to the manipulated text and therefore be vulnerable to the very attack the protection model was put in place to prevent. The tokenizer is tied to model architecture, meaning it is possible to predict whether or not a model is vulnerable to attack based on family. We also present a defensive strategy as an added layer of protection that can be implemented without having to retrain the defensive model.
Via
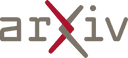
Jun 09, 2025
Abstract:We introduce MultiMatch, a novel semi-supervised learning (SSL) algorithm combining the paradigms of co-training and consistency regularization with pseudo-labeling. At its core, MultiMatch features a three-fold pseudo-label weighting module designed for three key purposes: selecting and filtering pseudo-labels based on head agreement and model confidence, and weighting them according to the perceived classification difficulty. This novel module enhances and unifies three existing techniques -- heads agreement from Multihead Co-training, self-adaptive thresholds from FreeMatch, and Average Pseudo-Margins from MarginMatch -- resulting in a holistic approach that improves robustness and performance in SSL settings. Experimental results on benchmark datasets highlight the superior performance of MultiMatch, achieving state-of-the-art results on 9 out of 10 setups from 5 natural language processing datasets and ranking first according to the Friedman test among 19 methods. Furthermore, MultiMatch demonstrates exceptional robustness in highly imbalanced settings, outperforming the second-best approach by 3.26% -- and data imbalance is a key factor for many text classification tasks.
Via
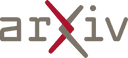
Jun 08, 2025
Abstract:Progress in Natural Language Processing (NLP) has been dictated by the rule of more: more data, more computing power and more complexity, best exemplified by the Large Language Models. However, training (or fine-tuning) large dense models for specific applications usually requires significant amounts of computing resources. This \textbf{Ph.D. dissertation} focuses on an under-investi\-gated NLP data engineering technique, whose potential is enormous in the current scenario known as Instance Selection (IS). The IS goal is to reduce the training set size by removing noisy or redundant instances while maintaining the effectiveness of the trained models and reducing the training process cost. We provide a comprehensive and scientifically sound comparison of IS methods applied to an essential NLP task -- Automatic Text Classification (ATC), considering several classification solutions and many datasets. Our findings reveal a significant untapped potential for IS solutions. We also propose two novel IS solutions that are noise-oriented and redundancy-aware, specifically designed for large datasets and transformer architectures. Our final solution achieved an average reduction of 41\% in training sets, while maintaining the same levels of effectiveness in all datasets. Importantly, our solutions demonstrated speedup improvements of 1.67x (up to 2.46x), making them scalable for datasets with hundreds of thousands of documents.
* 16 pages, 5 figures, 2 tables
Via
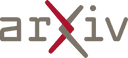
Jun 09, 2025
Abstract:Modern large language models (LLMs) are inherently auto-regressive, requiring input to be serialized into flat sequences regardless of their structural dependencies. This serialization hinders the model's ability to leverage structural inductive biases, especially in tasks such as retrieval-augmented generation (RAG) and reasoning on data with native graph structures, where inter-segment dependencies are crucial. We introduce Graph-KV with the potential to overcome this limitation. Graph-KV leverages the KV-cache of text segments as condensed representations and governs their interaction through structural inductive biases. In this framework, 'target' segments selectively attend only to the KV-caches of their designated 'source' segments, rather than all preceding segments in a serialized sequence. This approach induces a graph-structured block mask, sparsifying attention and enabling a message-passing-like step within the LLM. Furthermore, strategically allocated positional encodings for source and target segments reduce positional bias and context window consumption. We evaluate Graph-KV across three scenarios: (1) seven RAG benchmarks spanning direct inference, multi-hop reasoning, and long-document understanding; (2) Arxiv-QA, a novel academic paper QA task with full-text scientific papers structured as citation ego-graphs; and (3) paper topic classification within a citation network. By effectively reducing positional bias and harnessing structural inductive biases, Graph-KV substantially outperforms baselines, including standard costly sequential encoding, across various settings. Code and the Graph-KV data are publicly available.
Via
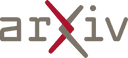
Jun 07, 2025
Abstract:The explosion of textual data has made manual document classification increasingly challenging. To address this, we introduce a robust, efficient domain-agnostic generative model framework for multi-label text classification. Instead of treating labels as mere atomic symbols, our approach utilizes predefined label descriptions and is trained to generate these descriptions based on the input text. During inference, the generated descriptions are matched to the pre-defined labels using a finetuned sentence transformer. We integrate this with a dual-objective loss function, combining cross-entropy loss and cosine similarity of the generated sentences with the predefined target descriptions, ensuring both semantic alignment and accuracy. Our proposed model LAGAMC stands out for its parameter efficiency and versatility across diverse datasets, making it well-suited for practical applications. We demonstrate the effectiveness of our proposed model by achieving new state-of-the-art performances across all evaluated datasets, surpassing several strong baselines. We achieve improvements of 13.94% in Micro-F1 and 24.85% in Macro-F1 compared to the closest baseline across all datasets.
* This work has been accepted to appear at the Association for
Computational Linguistics (ACL), 2025
Via
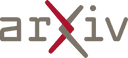
Jun 09, 2025
Abstract:Large Vision-Language Models (VLMs) now generate highly detailed, paragraphlength image captions, yet evaluating their factual accuracy remains challenging. Current methods often miss fine-grained errors, being designed for shorter texts or lacking datasets with verified inaccuracies. We introduce DOCCI-Critique, a benchmark with 1,400 VLM-generated paragraph captions (100 images, 14 VLMs) featuring over 10,216 sentence-level human annotations of factual correctness and explanatory rationales for errors, all within paragraph context. Building on this, we develop VNLI-Critique, a model for automated sentence-level factuality classification and critique generation. We highlight three key applications: (1) VNLI-Critique demonstrates robust generalization, validated by state-of-the-art performance on the M-HalDetect benchmark and strong results in CHOCOLATE claim verification. (2) The VNLI-Critique driven AutoRater for DOCCI-Critique provides reliable VLM rankings, showing excellent alignment with human factuality judgments (e.g., 0.98 Spearman). (3) An innovative Critic-and-Revise pipeline, where critiques from VNLI-Critique guide LLM-based corrections, achieves substantial improvements in caption factuality (e.g., a 46% gain on DetailCaps-4870). Our work offers a crucial benchmark alongside practical tools, designed to significantly elevate the standards for fine-grained evaluation and foster the improvement of VLM image understanding. Project page: https://google.github.io/unblocking-detail-caption
Via
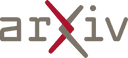
Jun 09, 2025
Abstract:This report presents a unified instruction-based framework for learning generalized text embeddings optimized for both information retrieval (IR) and non-IR tasks. Built upon a decoder-only large language model (Mistral-7B), our approach combines in-context learning, soft supervision, and adaptive hard-negative mining to generate context-aware embeddings without task-specific fine-tuning. Structured instructions and few-shot examples are used to guide the model across diverse tasks, enabling strong performance on classification, semantic similarity, clustering, and reranking benchmarks. To improve semantic discrimination, we employ a soft labeling framework where continuous relevance scores, distilled from a high-performance dense retriever and reranker, serve as fine-grained supervision signals. In addition, we introduce adaptive margin-based hard-negative mining, which filters out semantically ambiguous negatives based on their similarity to positive examples, thereby enhancing training stability and retrieval robustness. Our model is evaluated on the newly introduced MTEB (English, v2) benchmark, covering 41 tasks across seven categories. Results show that our method achieves strong generalization and ranks among the top-performing models by Borda score, outperforming several larger or fully fine-tuned baselines. These findings highlight the effectiveness of combining in-context prompting, soft supervision, and adaptive sampling for scalable, high-quality embedding generation.
* 10 pages
Via
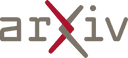
Jun 06, 2025
Abstract:Our contribution to the SemEval 2025 shared task 10, subtask 1 on entity framing, tackles the challenge of providing the necessary segments from longer documents as context for classification with a masked language model. We show that a simple entity-oriented heuristics for context selection can enable text classification using models with limited context window. Our context selection approach and the XLM-RoBERTa language model is on par with, or outperforms, Supervised Fine-Tuning with larger generative language models.
* Accepted for SemEval 2025; The 19th International Workshop on
Semantic Evaluation
Via
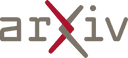
Jun 07, 2025
Abstract:Accurate and interpretable detection of depressive language in social media is useful for early interventions of mental health conditions, and has important implications for both clinical practice and broader public health efforts. In this paper, we investigate the performance of large language models (LLMs) and traditional machine learning classifiers across three classification tasks involving social media data: binary depression classification, depression severity classification, and differential diagnosis classification among depression, PTSD, and anxiety. Our study compares zero-shot LLMs with supervised classifiers trained on both conventional text embeddings and LLM-generated summary embeddings. Our experiments reveal that while zero-shot LLMs demonstrate strong generalization capabilities in binary classification, they struggle with fine-grained ordinal classifications. In contrast, classifiers trained on summary embeddings generated by LLMs demonstrate competitive, and in some cases superior, performance on the classification tasks, particularly when compared to models using traditional text embeddings. Our findings demonstrate the strengths of LLMs in mental health prediction, and suggest promising directions for better utilization of their zero-shot capabilities and context-aware summarization techniques.
* Submitted to the IEEE EMBS BHI 2025 Conference
Via
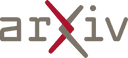
Jun 08, 2025
Abstract:In the realm of Text-attributed Graphs (TAGs), traditional graph neural networks (GNNs) often fall short due to the complex textual information associated with each node. Recent methods have improved node representations by leveraging large language models (LLMs) to enhance node text features, but these approaches typically require extensive annotations or fine-tuning across all nodes, which is both time-consuming and costly. To overcome these challenges, we introduce GAGA, an efficient framework for TAG representation learning. GAGA reduces annotation time and cost by focusing on annotating only representative nodes and edges. It constructs an annotation graph that captures the topological relationships among these annotations. Furthermore, GAGA employs a two-level alignment module to effectively integrate the annotation graph with the TAG, aligning their underlying structures. Experiments show that GAGA achieves classification accuracies on par with or surpassing state-of-the-art methods while requiring only 1% of the data to be annotated, demonstrating its high efficiency.
* 23 pages
Via
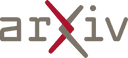