Topic:Document Binarization
What is Document Binarization? Document binarization is the process of converting grayscale or color documents into binary images.
Papers and Code
Mar 11, 2025
Abstract:In recent years, partial differential equation (PDE) systems have been successfully applied to the binarization of text images, achieving promising results. Inspired by the DH model and incorporating a novel image modeling approach, this study proposes a new weakly coupled PDE system for degraded text image binarization. In this system, the first equation is designed to estimate the background component, incorporating both diffusion and fidelity terms. The second equation estimates the foreground component and includes diffusion, fidelity, and binarization source terms. The final binarization result is obtained by applying a hard projection to the estimated foreground component. Experimental results on 86 degraded text images demonstrate that the proposed model exhibits significant advantages in handling degraded text images.
* 30pages,13figures,2tables
Via
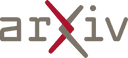
Dec 05, 2024
Abstract:Document comparison typically relies on optical character recognition (OCR) as its core technology. However, OCR requires the selection of appropriate language models for each document and the performance of multilingual or hybrid models remains limited. To overcome these challenges, we propose text change detection (TCD) using an image comparison model tailored for multilingual documents. Unlike OCR-based approaches, our method employs word-level text image-to-image comparison to detect changes. Our model generates bidirectional change segmentation maps between the source and target documents. To enhance performance without requiring explicit text alignment or scaling preprocessing, we employ correlations among multi-scale attention features. We also construct a benchmark dataset comprising actual printed and scanned word pairs in various languages to evaluate our model. We validate our approach using our benchmark dataset and public benchmarks Distorted Document Images and the LRDE Document Binarization Dataset. We compare our model against state-of-the-art semantic segmentation and change detection models, as well as to conventional OCR-based models.
* 15pages, 11figures 6tables, wacv2025 accepted
Via
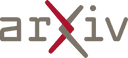
Dec 02, 2024
Abstract:The problem of converting images of text into plain text is a widely researched topic in both academia and industry. Arabic handwritten Text Recognation (AHTR) poses additional challenges due to diverse handwriting styles and limited labeled data. In this paper we present a complete OCR pipeline that starts with line segmentation using Differentiable Binarization and Adaptive Scale Fusion techniques to ensure accurate detection of text lines. Following segmentation, a CNN-BiLSTM-CTC architecture is applied to recognize characters. Our system, trained on the Arabic Multi-Fonts Dataset (AMFDS), achieves a Character Recognition Rate (CRR) of 99.20% and a Word Recognition Rate (WRR) of 93.75% on single-word samples containing 7 to 10 characters, along with a CRR of 83.76% for sentences. These results demonstrate the system's strong performance in handling Arabic scripts, establishing a new benchmark for AHTR systems.
Via
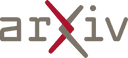
Dec 16, 2024
Abstract:Historical documents encompass a wealth of cultural treasures but suffer from severe damages including character missing, paper damage, and ink erosion over time. However, existing document processing methods primarily focus on binarization, enhancement, etc., neglecting the repair of these damages. To this end, we present a new task, termed Historical Document Repair (HDR), which aims to predict the original appearance of damaged historical documents. To fill the gap in this field, we propose a large-scale dataset HDR28K and a diffusion-based network DiffHDR for historical document repair. Specifically, HDR28K contains 28,552 damaged-repaired image pairs with character-level annotations and multi-style degradations. Moreover, DiffHDR augments the vanilla diffusion framework with semantic and spatial information and a meticulously designed character perceptual loss for contextual and visual coherence. Experimental results demonstrate that the proposed DiffHDR trained using HDR28K significantly surpasses existing approaches and exhibits remarkable performance in handling real damaged documents. Notably, DiffHDR can also be extended to document editing and text block generation, showcasing its high flexibility and generalization capacity. We believe this study could pioneer a new direction of document processing and contribute to the inheritance of invaluable cultures and civilizations. The dataset and code is available at https://github.com/yeungchenwa/HDR.
* 39th AAAI Conference on Artificial Intelligence (AAAI-25),
Philadelphia, Pennsylvania, USA, 2025
* Accepted to AAAI 2025; Github Page:
https://github.com/yeungchenwa/HDR
Via
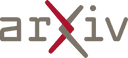
Jul 05, 2024
Abstract:For document image binarization task, generative adversarial networks (GANs) can generate images where shadows and noise are effectively removed, which allow for text information extraction. The current state-of-the-art (SOTA) method proposes a three-stage network architecture that utilizes six GANs. Despite its excellent model performance, the SOTA network architecture requires long training and inference times. To overcome this problem, this work introduces an efficient GAN method based on the three-stage network architecture that incorporates the Discrete Wavelet Transformation and normalization to reduce the input image size, which in turns, decrease both training and inference times. In addition, this work presents novel generators, discriminators, and loss functions to improve the model's performance. Experimental results show that the proposed method reduces the training time by 10% and the inference time by 26% when compared to the SOTA method while maintaining the model performance at 73.79 of Avg-Score. Our implementation code is available on GitHub at https://github.com/RuiyangJu/Efficient_Document_Image_Binarization.
Via
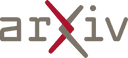
Apr 26, 2024
Abstract:Document Image Binarization is a well-known problem in Document Analysis and Computer Vision, although it is far from being solved. One of the main challenges of this task is that documents generally exhibit degradations and acquisition artifacts that can greatly vary throughout the page. Nonetheless, even when dealing with a local patch of the document, taking into account the overall appearance of a wide portion of the page can ease the prediction by enriching it with semantic information on the ink and background conditions. In this respect, approaches able to model both local and global information have been proven suitable for this task. In particular, recent applications of Vision Transformer (ViT)-based models, able to model short and long-range dependencies via the attention mechanism, have demonstrated their superiority over standard Convolution-based models, which instead struggle to model global dependencies. In this work, we propose an alternative solution based on the recently introduced Fast Fourier Convolutions, which overcomes the limitation of standard convolutions in modeling global information while requiring fewer parameters than ViTs. We validate the effectiveness of our approach via extensive experimental analysis considering different types of degradations.
* Accepted at ICDAR2024
Via
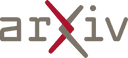
May 07, 2024
Abstract:Document image restoration is a crucial aspect of Document AI systems, as the quality of document images significantly influences the overall performance. Prevailing methods address distinct restoration tasks independently, leading to intricate systems and the incapability to harness the potential synergies of multi-task learning. To overcome this challenge, we propose DocRes, a generalist model that unifies five document image restoration tasks including dewarping, deshadowing, appearance enhancement, deblurring, and binarization. To instruct DocRes to perform various restoration tasks, we propose a novel visual prompt approach called Dynamic Task-Specific Prompt (DTSPrompt). The DTSPrompt for different tasks comprises distinct prior features, which are additional characteristics extracted from the input image. Beyond its role as a cue for task-specific execution, DTSPrompt can also serve as supplementary information to enhance the model's performance. Moreover, DTSPrompt is more flexible than prior visual prompt approaches as it can be seamlessly applied and adapted to inputs with high and variable resolutions. Experimental results demonstrate that DocRes achieves competitive or superior performance compared to existing state-of-the-art task-specific models. This underscores the potential of DocRes across a broader spectrum of document image restoration tasks. The source code is publicly available at https://github.com/ZZZHANG-jx/DocRes
* Accepted by CVPR 2024
Via
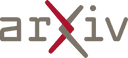
Jan 22, 2024
Abstract:Binarization of document images is an important pre-processing step in the field of document analysis. Traditional image binarization techniques usually rely on histograms or local statistics to identify a valid threshold to differentiate between different aspects of the image. Deep learning techniques are able to generate binarized versions of the images by learning context-dependent features that are less error-prone to degradation typically occurring in document images. In recent years, many deep learning-based methods have been developed for document binarization. But which one to choose? There have been no studies that compare these methods rigorously. Therefore, this work focuses on the evaluation of different deep learning-based methods under the same evaluation protocol. We evaluate them on different Document Image Binarization Contest (DIBCO) datasets and obtain very heterogeneous results. We show that the DE-GAN model was able to perform better compared to other models when evaluated on the DIBCO2013 dataset while DP-LinkNet performed best on the DIBCO2017 dataset. The 2-StageGAN performed best on the DIBCO2018 dataset while SauvolaNet outperformed the others on the DIBCO2019 challenge. Finally, we make the code, all models and evaluation publicly available (https://github.com/RichSu95/Document_Binarization_Collection) to ensure reproducibility and simplify future binarization evaluations.
* Document Analysis Systems. DAS 2022. Lecture Notes in Computer
Science, vol 13237. Springer, Cham
* DAS 2022
Via
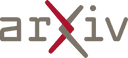
Apr 26, 2024
Abstract:This paper introduces SAGHOG, a self-supervised pretraining strategy for writer retrieval using HOG features of the binarized input image. Our preprocessing involves the application of the Segment Anything technique to extract handwriting from various datasets, ending up with about 24k documents, followed by training a vision transformer on reconstructing masked patches of the handwriting. SAGHOG is then finetuned by appending NetRVLAD as an encoding layer to the pretrained encoder. Evaluation of our approach on three historical datasets, Historical-WI, HisFrag20, and GRK-Papyri, demonstrates the effectiveness of SAGHOG for writer retrieval. Additionally, we provide ablation studies on our architecture and evaluate un- and supervised finetuning. Notably, on HisFrag20, SAGHOG outperforms related work with a mAP of 57.2 % - a margin of 11.6 % to the current state of the art, showcasing its robustness on challenging data, and is competitive on even small datasets, e.g. GRK-Papyri, where we achieve a Top-1 accuracy of 58.0%.
* accepted for ICDAR2024
Via
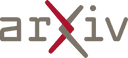
Dec 06, 2023
Abstract:In real life, various degradation scenarios exist that might damage document images, making it harder to recognize and analyze them, thus binarization is a fundamental and crucial step for achieving the most optimal performance in any document analysis task. We propose DocBinFormer (Document Binarization Transformer), a novel two-level vision transformer (TL-ViT) architecture based on vision transformers for effective document image binarization. The presented architecture employs a two-level transformer encoder to effectively capture both global and local feature representation from the input images. These complimentary bi-level features are exploited for efficient document image binarization, resulting in improved results for system-generated as well as handwritten document images in a comprehensive approach. With the absence of convolutional layers, the transformer encoder uses the pixel patches and sub-patches along with their positional information to operate directly on them, while the decoder generates a clean (binarized) output image from the latent representation of the patches. Instead of using a simple vision transformer block to extract information from the image patches, the proposed architecture uses two transformer blocks for greater coverage of the extracted feature space on a global and local scale. The encoded feature representation is used by the decoder block to generate the corresponding binarized output. Extensive experiments on a variety of DIBCO and H-DIBCO benchmarks show that the proposed model outperforms state-of-the-art techniques on four metrics. The source code will be made available at https://github.com/RisabBiswas/DocBinFormer.
Via
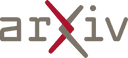