What is music generation? Music generation is the task of generating music or music-like sounds from a model or algorithm.
Papers and Code
May 15, 2025
Abstract:Modal synthesis methods are a long-standing approach for modelling distributed musical systems. In some cases extensions are possible in order to handle geometric nonlinearities. One such case is the high-amplitude vibration of a string, where geometric nonlinear effects lead to perceptually important effects including pitch glides and a dependence of brightness on striking amplitude. A modal decomposition leads to a coupled nonlinear system of ordinary differential equations. Recent work in applied machine learning approaches (in particular neural ordinary differential equations) has been used to model lumped dynamic systems such as electronic circuits automatically from data. In this work, we examine how modal decomposition can be combined with neural ordinary differential equations for modelling distributed musical systems. The proposed model leverages the analytical solution for linear vibration of system's modes and employs a neural network to account for nonlinear dynamic behaviour. Physical parameters of a system remain easily accessible after the training without the need for a parameter encoder in the network architecture. As an initial proof of concept, we generate synthetic data for a nonlinear transverse string and show that the model can be trained to reproduce the nonlinear dynamics of the system. Sound examples are presented.
* Accepted for publication in Proceedings of the 28th International
Conference on Digital Audio Effects (DAFx25), Ancona, Italy, September 2025
Via
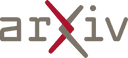
Apr 29, 2025
Abstract:Online platforms are increasingly interested in using Data-to-Text technologies to generate content and help their users. Unfortunately, traditional generative methods often fall into repetitive patterns, resulting in monotonous galleries of texts after only a few iterations. In this paper, we investigate LLM-based data-to-text approaches to automatically generate marketing texts that are of sufficient quality and diverse enough for broad adoption. We leverage Language Models such as T5, GPT-3.5, GPT-4, and LLaMa2 in conjunction with fine-tuning, few-shot, and zero-shot approaches to set a baseline for diverse marketing texts. We also introduce a metric JaccDiv to evaluate the diversity of a set of texts. This research extends its relevance beyond the music industry, proving beneficial in various fields where repetitive automated content generation is prevalent.
Via
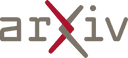
May 07, 2025
Abstract:Ornamentations, embellishments, or microtonal inflections are essential to melodic expression across many musical traditions, adding depth, nuance, and emotional impact to performances. Recognizing ornamentations in singing voices is key to MIR, with potential applications in music pedagogy, singer identification, genre classification, and controlled singing voice generation. However, the lack of annotated datasets and specialized modeling approaches remains a major obstacle for progress in this research area. In this work, we introduce R\=aga Ornamentation Detection (ROD), a novel dataset comprising Indian classical music recordings curated by expert musicians. The dataset is annotated using a custom Human-in-the-Loop tool for six vocal ornaments marked as event-based labels. Using this dataset, we develop an ornamentation detection model based on deep time-series analysis, preserving ornament boundaries during the chunking of long audio recordings. We conduct experiments using different train-test configurations within the ROD dataset and also evaluate our approach on a separate, manually annotated dataset of Indian classical concert recordings. Our experimental results support the superior performance of our proposed approach over the baseline CRNN.
Via
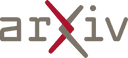
May 08, 2025
Abstract:Reactive dance generation (RDG) produces follower movements conditioned on guiding dancer and music while ensuring spatial coordination and temporal coherence. However, existing methods overemphasize global constraints and optimization, overlooking local information, such as fine-grained spatial interactions and localized temporal context. Therefore, we present ReactDance, a novel diffusion-based framework for high-fidelity RDG with long-term coherence and multi-scale controllability. Unlike existing methods that struggle with interaction fidelity, synchronization, and temporal consistency in duet synthesis, our approach introduces two key innovations: 1)Group Residual Finite Scalar Quantization (GRFSQ), a multi-scale disentangled motion representation that captures interaction semantics from coarse body rhythms to fine-grained joint dynamics, and 2)Blockwise Local Context (BLC), a sampling strategy eliminating error accumulation in long sequence generation via local block causal masking and periodic positional encoding. Built on the decoupled multi-scale GRFSQ representation, we implement a diffusion model withLayer-Decoupled Classifier-free Guidance (LDCFG), allowing granular control over motion semantics across scales. Extensive experiments on standard benchmarks demonstrate that ReactDance surpasses existing methods, achieving state-of-the-art performance.
Via
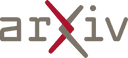
Apr 18, 2025
Abstract:Music generation aims to create music segments that align with human aesthetics based on diverse conditional information. Despite advancements in generating music from specific textual descriptions (e.g., style, genre, instruments), the practical application is still hindered by ordinary users' limited expertise or time to write accurate prompts. To bridge this application gap, this paper introduces MusFlow, a novel multimodal music generation model using Conditional Flow Matching. We employ multiple Multi-Layer Perceptrons (MLPs) to align multimodal conditional information into the audio's CLAP embedding space. Conditional flow matching is trained to reconstruct the compressed Mel-spectrogram in the pretrained VAE latent space guided by aligned feature embedding. MusFlow can generate music from images, story texts, and music captions. To collect data for model training, inspired by multi-agent collaboration, we construct an intelligent data annotation workflow centered around a fine-tuned Qwen2-VL model. Using this workflow, we build a new multimodal music dataset, MMusSet, with each sample containing a quadruple of image, story text, music caption, and music piece. We conduct four sets of experiments: image-to-music, story-to-music, caption-to-music, and multimodal music generation. Experimental results demonstrate that MusFlow can generate high-quality music pieces whether the input conditions are unimodal or multimodal. We hope this work can advance the application of music generation in multimedia field, making music creation more accessible. Our generated samples, code and dataset are available at musflow.github.io.
Via
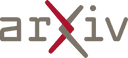
Apr 18, 2025
Abstract:With the recent developments in machine intelligence and web technologies, new generative music systems are being explored for assisted composition using machine learning techniques on the web. Such systems are built for various tasks such as melodic, harmonic or rhythm generation, music interpolation, continuation and style imitation. In this paper, we introduce Apollo, an interactive music application for generating symbolic phrases of conventional western music using corpus-based style imitation techniques. In addition to enabling the construction and management of symbolic musical corpora, the system makes it possible for music artists and researchers to generate new musical phrases in the style of the proposed corpus. The system is available as a desktop application. The generated symbolic music materials, encoded in the MIDI format, can be exported or streamed for various purposes including using them as seed material for musical projects. We present the system design, implementation details, discuss and conclude with future work for the system.
* 7 pages, 5 figures, Published as a paper at the 7th International
Workshop on Musical Metacreation (MUME 2019), UNC Charlotte, North Carolina
Via
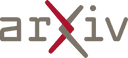
Apr 18, 2025
Abstract:With the rise of artificial intelligence in recent years, there has been a rapid increase in its application towards creative domains, including music. There exist many systems built that apply machine learning approaches to the problem of computer-assisted music composition (CAC). Calliope is a web application that assists users in performing a variety of multi-track composition tasks in the symbolic domain. The user can upload (Musical Instrument Digital Interface) MIDI files, visualize and edit MIDI tracks, and generate partial (via bar in-filling) or complete multi-track content using the Multi-Track Music Machine (MMM). Generation of new MIDI excerpts can be done in batch and can be combined with active playback listening for an enhanced assisted-composition workflow. The user can export generated MIDI materials or directly stream MIDI playback from the system to their favorite Digital Audio Workstation (DAW). We present a demonstration of the system, its features, generative parameters and describe the co-creative workflows that it affords.
* 5 pages, 5 figures, first published at the 13th International
Conference on Computational Creativity (ICCC 2022), Bozen-Bolzano, Italy
Via
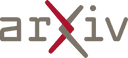
Apr 10, 2025
Abstract:Music profoundly enhances video production by improving quality, engagement, and emotional resonance, sparking growing interest in video-to-music generation. Despite recent advances, existing approaches remain limited in specific scenarios or undervalue the visual dynamics. To address these limitations, we focus on tackling the complexity of dynamics and resolving temporal misalignment between video and music representations. To this end, we propose DyViM, a novel framework to enhance dynamics modeling for video-to-music generation. Specifically, we extract frame-wise dynamics features via a simplified motion encoder inherited from optical flow methods, followed by a self-attention module for aggregation within frames. These dynamic features are then incorporated to extend existing music tokens for temporal alignment. Additionally, high-level semantics are conveyed through a cross-attention mechanism, and an annealing tuning strategy benefits to fine-tune well-trained music decoders efficiently, therefore facilitating seamless adaptation. Extensive experiments demonstrate DyViM's superiority over state-of-the-art (SOTA) methods.
* Under review
Via
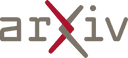
Apr 18, 2025
Abstract:With the rise of artificial intelligence (AI), there has been increasing interest in human-AI co-creation in a variety of artistic domains including music as AI-driven systems are frequently able to generate human-competitive artifacts. Now, the implications of such systems for musical practice are being investigated. We report on a thorough evaluation of the user adoption of the Multi-Track Music Machine (MMM) as a co-creative AI tool for music composers. To do this, we integrate MMM into Cubase, a popular Digital Audio Workstation (DAW) by Steinberg, by producing a "1-parameter" plugin interface named MMM-Cubase (MMM-C), which enables human-AI co-composition. We contribute a methodological assemblage as a 3-part mixed method study measuring usability, user experience and technology acceptance of the system across two groups of expert-level composers: hobbyists and professionals. Results show positive usability and acceptance scores. Users report experiences of novelty, surprise and ease of use from using the system, and limitations on controllability and predictability of the interface when generating music. Findings indicate no significant difference between the two user groups.
* 10 pages, 6 figures, 1 table, first published at the 32nd
International Joint Conference on Artificial Intelligence (IJCAI 2023),
Macao, China
Via
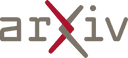
Apr 12, 2025
Abstract:In recent years, text-to-audio systems have achieved remarkable success, enabling the generation of complete audio segments directly from text descriptions. While these systems also facilitate music creation, the element of human creativity and deliberate expression is often limited. In contrast, the present work allows composers, arrangers, and performers to create the basic building blocks for music creation: audio of individual musical notes for use in electronic instruments and DAWs. Through text prompts, the user can specify the timbre characteristics of the audio. We introduce a system that combines a latent diffusion model and multi-modal contrastive learning to generate musical timbres conditioned on text descriptions. By jointly generating the magnitude and phase of the spectrogram, our method eliminates the need for subsequently running a phase retrieval algorithm, as related methods do. Audio examples, source code, and a web app are available at https://wxuanyuan.github.io/Musical-Note-Generation/
* 10 pages, 5 figures
Via
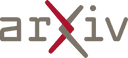