What is cancer detection? Cancer detection using Artificial Intelligence (AI) involves leveraging advanced machine learning algorithms and techniques to identify and diagnose cancer from various medical data sources. The goal is to enhance early detection, improve diagnostic accuracy, and potentially reduce the need for invasive procedures.
Papers and Code
Dec 23, 2024
Abstract:Breast cancer detection based on pre-trained convolution neural network (CNN) has gained much interest among other conventional computer-based systems. In the past few years, CNN technology has been the most promising way to find cancer in mammogram scans. In this paper, the effect of layer freezing in a pre-trained CNN is investigated for breast cancer detection by classifying mammogram images as benign or malignant. Different VGG19 scenarios have been examined based on the number of convolution layer blocks that have been frozen. There are a total of six scenarios in this study. The primary benefits of this research are twofold: it improves the model's ability to detect breast cancer cases and it reduces the training time of VGG19 by freezing certain layers.To evaluate the performance of these scenarios, 1693 microbiological images of benign and malignant breast cancers were utilized. According to the reported results, the best recognition rate was obtained from a frozen first block of VGG19 with a sensitivity of 95.64 %, while the training of the entire VGG19 yielded 94.48%.
* Its a conference paper; the full proceeding is avalible at
https://icogoia.utem.edu.my/proceedings.html
Via
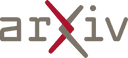
Dec 31, 2024
Abstract:Lung cancer is an extremely lethal disease primarily due to its late-stage diagnosis and significant mortality rate, making it the major cause of cancer-related demises globally. Machine Learning (ML) and Convolution Neural network (CNN) based Deep Learning (DL) techniques are primarily used for precise segmentation and classification of cancerous nodules in the CT (Computed Tomography) or MRI images. This study introduces an innovative approach to lung nodule segmentation by utilizing the Segment Anything Model (SAM) combined with transfer learning techniques. Precise segmentation of lung nodules is crucial for the early detection of lung cancer. The proposed method leverages Bounding Box prompts and a vision transformer model to enhance segmentation performance, achieving high accuracy, Dice Similarity Coefficient (DSC) and Intersection over Union (IoU) metrics. The integration of SAM and Transfer Learning significantly improves Computer-Aided Detection (CAD) systems in medical imaging, particularly for lung cancer diagnosis. The findings demonstrate the proposed model effectiveness in precisely segmenting lung nodules from CT scans, underscoring its potential to advance early detection and improve patient care outcomes in lung cancer diagnosis. The results show SAM Model with transfer learning achieving a DSC of 97.08% and an IoU of 95.6%, for segmentation and accuracy of 96.71% for classification indicates that ,its performance is noteworthy compared to existing techniques.
Via
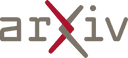
Jan 03, 2025
Abstract:Cancer remains a significant health challenge worldwide, with a new diagnosis occurring every two minutes in the UK. Surgery is one of the main treatment options for cancer. However, surgeons rely on the sense of touch and naked eye with limited use of pre-operative image data to directly guide the excision of cancerous tissues and metastases due to the lack of reliable intraoperative visualisation tools. This leads to increased costs and harm to the patient where the cancer is removed with positive margins, or where other critical structures are unintentionally impacted. There is therefore a pressing need for more reliable and accurate intraoperative visualisation tools for minimally invasive surgery to improve surgical outcomes and enhance patient care. A recent miniaturised cancer detection probe (i.e., SENSEI developed by Lightpoint Medical Ltd.) leverages the cancer-targeting ability of nuclear agents to more accurately identify cancer intra-operatively using the emitted gamma signal. However, the use of this probe presents a visualisation challenge as the probe is non-imaging and is air-gapped from the tissue, making it challenging for the surgeon to locate the probe-sensing area on the tissue surface. Geometrically, the sensing area is defined as the intersection point between the gamma probe axis and the tissue surface in 3D space but projected onto the 2D laparoscopic image. Hence, in this thesis, tool tracking, pose estimation, and segmentation tools were developed first, followed by laparoscope image depth estimation algorithms and 3D reconstruction methods.
* Doctoral thesis
Via
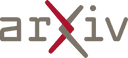
Jan 30, 2025
Abstract:Lung cancer is a major issue in worldwide public health, requiring early diagnosis using stable techniques. This work begins a thorough investigation of the use of machine learning (ML) methods for precise classification of lung cancer stages. A cautious analysis is performed to overcome overfitting issues in model performance, taking into account minimum child weight and learning rate. A set of machine learning (ML) models including XGBoost (XGB), LGBM, Adaboost, Logistic Regression (LR), Decision Tree (DT), Random Forest (RF), CatBoost, and k-Nearest Neighbor (k-NN) are run methodically and contrasted. Furthermore, the correlation between features and targets is examined using the deep neural network (DNN) model and thus their capability in detecting complex patternsis established. It is argued that several ML models can be capable of classifying lung cancer stages with great accuracy. In spite of the complexity of DNN architectures, traditional ML models like XGBoost, LGBM, and Logistic Regression excel with superior performance. The models perform better than the others in lung cancer prediction on the complete set of comparative metrics like accuracy, precision, recall, and F-1 score
Via
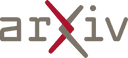
Jan 29, 2025
Abstract:Automated diagnostic systems (ADS) have shown significant potential in the early detection of polyps during endoscopic examinations, thereby reducing the incidence of colorectal cancer. However, due to high annotation costs and strict privacy concerns, acquiring high-quality endoscopic images poses a considerable challenge in the development of ADS. Despite recent advancements in generating synthetic images for dataset expansion, existing endoscopic image generation algorithms failed to accurately generate the details of polyp boundary regions and typically required medical priors to specify plausible locations and shapes of polyps, which limited the realism and diversity of the generated images. To address these limitations, we present Polyp-Gen, the first full-automatic diffusion-based endoscopic image generation framework. Specifically, we devise a spatial-aware diffusion training scheme with a lesion-guided loss to enhance the structural context of polyp boundary regions. Moreover, to capture medical priors for the localization of potential polyp areas, we introduce a hierarchical retrieval-based sampling strategy to match similar fine-grained spatial features. In this way, our Polyp-Gen can generate realistic and diverse endoscopic images for building reliable ADS. Extensive experiments demonstrate the state-of-the-art generation quality, and the synthetic images can improve the downstream polyp detection task. Additionally, our Polyp-Gen has shown remarkable zero-shot generalizability on other datasets. The source code is available at https://github.com/CUHK-AIM-Group/Polyp-Gen.
* Accepted by ICRA 2025
Via
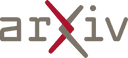
Dec 16, 2024
Abstract:Pulmonary pathologies are a significant global health concern, often leading to fatal outcomes if not diagnosed and treated promptly. Chest radiography serves as a primary diagnostic tool, but the availability of experienced radiologists remains limited. Advances in Artificial Intelligence (AI) and machine learning, particularly in computer vision, offer promising solutions to address this challenge. This research evaluates a deep learning model designed to detect lung cancer, specifically pulmonary nodules, along with eight other lung pathologies, using chest radiographs. The study leverages diverse datasets comprising over 135,120 frontal chest radiographs to train a Convolutional Neural Network (CNN). A two-stage classification system, utilizing ensemble methods and transfer learning, is employed to first triage images into Normal or Abnormal categories and then identify specific pathologies, including lung nodules. The deep learning model achieves notable results in nodule classification, with a top-performing accuracy of 77%, a sensitivity of 0.713, a specificity of 0.776 during external validation, and an AUC score of 0.888. Despite these successes, some misclassifications were observed, primarily false negatives. In conclusion, the model demonstrates robust potential for generalization across diverse patient populations, attributed to the geographic diversity of the training dataset. Future work could focus on integrating ETL data distribution strategies and expanding the dataset with additional nodule-type samples to further enhance diagnostic accuracy.
* IEEE International Workshop on Mechatronic Systems Supervision 2023
Via
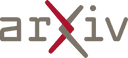
Jan 15, 2025
Abstract:Colorectal cancer (CRC) is one of the most commonly diagnosed cancers all over the world. It starts as a polyp in the inner lining of the colon. To prevent CRC, early polyp detection is required. Colonosopy is used for the inspection of the colon. Generally, the images taken by the camera placed at the tip of the endoscope are analyzed by the experts manually. Various traditional machine learning models have been used with the rise of machine learning. Recently, deep learning models have shown more effectiveness in polyp detection due to their superiority in generalizing and learning small features. These deep learning models for object detection can be segregated into two different types: single-stage and two-stage. Generally, two stage models have higher accuracy than single stage ones but the single stage models have low inference time. Hence, single stage models are easy to use for quick object detection. YOLO is one of the singlestage models used successfully for polyp detection. It has drawn the attention of researchers because of its lower inference time. The researchers have used Different versions of YOLO so far, and with each newer version, the accuracy of the model is increasing. This paper aims to see the effectiveness of the recently released YOLOv11 to detect polyp. We analyzed the performance for all five models of YOLOv11 (YOLO11n, YOLO11s, YOLO11m, YOLO11l, YOLO11x) with Kvasir dataset for the training and testing. Two different versions of the dataset were used. The first consisted of the original dataset, and the other was created using augmentation techniques. The performance of all the models with these two versions of the dataset have been analysed.
Via
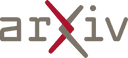
Jan 01, 2025
Abstract:Oral cancer constitutes a significant global health concern, resulting in 277,484 fatalities in 2023, with the highest prevalence observed in low- and middle-income nations. Facilitating automation in the detection of possibly malignant and malignant lesions in the oral cavity could result in cost-effective and early disease diagnosis. Establishing an extensive repository of meticulously annotated oral lesions is essential. In this research photos are being collected from global clinical experts, who have been equipped with an annotation tool to generate comprehensive labelling. This research presents a novel approach for integrating bounding box annotations from various doctors. Additionally, Deep Belief Network combined with CAPSNET is employed to develop automated systems that extracted intricate patterns to address this challenging problem. This study evaluated two deep learning-based computer vision methodologies for the automated detection and classification of oral lesions to facilitate the early detection of oral cancer: image classification utilizing CAPSNET. Image classification attained an F1 score of 94.23% for detecting photos with lesions 93.46% for identifying images necessitating referral. Object detection attained an F1 score of 89.34% for identifying lesions for referral. Subsequent performances are documented about classification based on the sort of referral decision. Our preliminary findings indicate that deep learning possesses the capability to address this complex problem.
* Accepted to IEEE International Conference on Advancement in
Communication and Computing Technology (INOACC), will be held in Sai Vidya
Institute of Technology, Bengaluru, Karnataka, India. (Preprint)
Via
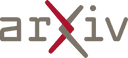
Dec 20, 2024
Abstract:Cases of laryngeal cancer are predicted to rise significantly in the coming years. Current diagnostic pathways cause many patients to be incorrectly referred to urgent suspected cancer pathways, putting undue stress on both patients and the medical system. Artificial intelligence offers a promising solution by enabling non-invasive detection of laryngeal cancer from patient speech, which could help prioritise referrals more effectively and reduce inappropriate referrals of non-cancer patients. To realise this potential, open science is crucial. A major barrier in this field is the lack of open-source datasets and reproducible benchmarks, forcing researchers to start from scratch. Our work addresses this challenge by introducing a benchmark suite comprising 36 models trained and evaluated on open-source datasets. These models are accessible in a public repository, providing a foundation for future research. They evaluate three different algorithms and three audio feature sets, offering a comprehensive benchmarking framework. We propose standardised metrics and evaluation methodologies to ensure consistent and comparable results across future studies. The presented models include both audio-only inputs and multimodal inputs that incorporate demographic and symptom data, enabling their application to datasets with diverse patient information. By providing these benchmarks, future researchers can evaluate their datasets, refine the models, and use them as a foundation for more advanced approaches. This work aims to provide a baseline for establishing reproducible benchmarks, enabling researchers to compare new methods against these standards and ultimately advancing the development of AI tools for detecting laryngeal cancer.
* 24 pages, 6 figures, 7 tables
Via
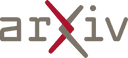
Dec 14, 2024
Abstract:Prostate cancer is a leading cause of cancer-related deaths among men. The recent development of high frequency, micro-ultrasound imaging offers improved resolution compared to conventional ultrasound and potentially a better ability to differentiate clinically significant cancer from normal tissue. However, the features of prostate cancer remain subtle, with ambiguous borders with normal tissue and large variations in appearance, making it challenging for both machine learning and humans to localize it on micro-ultrasound images. We propose a novel Mask Enhanced Deeply-supervised Micro-US network, termed MedMusNet, to automatically and more accurately segment prostate cancer to be used as potential targets for biopsy procedures. MedMusNet leverages predicted masks of prostate cancer to enforce the learned features layer-wisely within the network, reducing the influence of noise and improving overall consistency across frames. MedMusNet successfully detected 76% of clinically significant cancer with a Dice Similarity Coefficient of 0.365, significantly outperforming the baseline Swin-M2F in specificity and accuracy (Wilcoxon test, Bonferroni correction, p-value<0.05). While the lesion-level and patient-level analyses showed improved performance compared to human experts and different baseline, the improvements did not reach statistical significance, likely on account of the small cohort. We have presented a novel approach to automatically detect and segment clinically significant prostate cancer on B-mode micro-ultrasound images. Our MedMusNet model outperformed other models, surpassing even human experts. These preliminary results suggest the potential for aiding urologists in prostate cancer diagnosis via biopsy and treatment decision-making.
Via
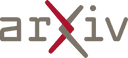