What is cancer detection? Cancer detection using Artificial Intelligence (AI) involves leveraging advanced machine learning algorithms and techniques to identify and diagnose cancer from various medical data sources. The goal is to enhance early detection, improve diagnostic accuracy, and potentially reduce the need for invasive procedures.
Papers and Code
Jul 08, 2025
Abstract:Objective: Latent diffusion models (LDMs) could mitigate data scarcity challenges affecting machine learning development for medical image interpretation. The recent CCELLA LDM improved prostate cancer detection performance using synthetic MRI for classifier training but was limited to the axial T2-weighted (AxT2) sequence, did not investigate inter-institutional domain shift, and prioritized radiology over histopathology outcomes. We propose CCELLA++ to address these limitations and improve clinical utility. Methods: CCELLA++ expands CCELLA for simultaneous biparametric prostate MRI (bpMRI) generation, including the AxT2, high b-value diffusion series (HighB) and apparent diffusion coefficient map (ADC). Domain adaptation was investigated by pretraining classifiers on real or LDM-generated synthetic data from an internal institution, followed with fine-tuning on progressively smaller fractions of an out-of-distribution, external dataset. Results: CCELLA++ improved 3D FID for HighB and ADC but not AxT2 (0.013, 0.012, 0.063 respectively) sequences compared to CCELLA (0.060). Classifier pretraining with CCELLA++ bpMRI outperformed real bpMRI in AP and AUC for all domain adaptation scenarios. CCELLA++ pretraining achieved highest classifier performance below 50% (n=665) external dataset volume. Conclusion: Synthetic bpMRI generated by our method can improve downstream classifier generalization and performance beyond real bpMRI or CCELLA-generated AxT2-only images. Future work should seek to quantify medical image sample quality, balance multi-sequence LDM training, and condition the LDM with additional information. Significance: The proposed CCELLA++ LDM can generate synthetic bpMRI that outperforms real data for domain adaptation with a limited target institution dataset. Our code is available at https://github.com/grabkeem/CCELLA-plus-plus
* BT and MAH are co-senior authors on the work. This work has been
submitted to the IEEE for possible publication
Via
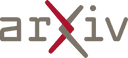
Jul 10, 2025
Abstract:Melanoma, one of the deadliest types of skin cancer, accounts for thousands of fatalities globally. The bluish, blue-whitish, or blue-white veil (BWV) is a critical feature for diagnosing melanoma, yet research into detecting BWV in dermatological images is limited. This study utilizes a non-annotated skin lesion dataset, which is converted into an annotated dataset using a proposed imaging algorithm based on color threshold techniques on lesion patches and color palettes. A Deep Convolutional Neural Network (DCNN) is designed and trained separately on three individual and combined dermoscopic datasets, using custom layers instead of standard activation function layers. The model is developed to categorize skin lesions based on the presence of BWV. The proposed DCNN demonstrates superior performance compared to conventional BWV detection models across different datasets. The model achieves a testing accuracy of 85.71% on the augmented PH2 dataset, 95.00% on the augmented ISIC archive dataset, 95.05% on the combined augmented (PH2+ISIC archive) dataset, and 90.00% on the Derm7pt dataset. An explainable artificial intelligence (XAI) algorithm is subsequently applied to interpret the DCNN's decision-making process regarding BWV detection. The proposed approach, coupled with XAI, significantly improves the detection of BWV in skin lesions, outperforming existing models and providing a robust tool for early melanoma diagnosis.
* Computers in Biology and Medicine, Vol. XXX, 2024, Article 108758
* Accepted version. Published in Computers in Biology and Medicine, 14
June 2024. DOI: 10.1016/j.compbiomed.2024.108758
Via
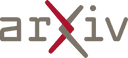
Jul 08, 2025
Abstract:Breast density assessment is a crucial component of mammographic interpretation, with high breast density (BI-RADS categories C and D) representing both a significant risk factor for developing breast cancer and a technical challenge for tumor detection. This study proposes an automated deep learning system for robust binary classification of breast density (low: A/B vs. high: C/D) using the VinDr-Mammo dataset. We implemented and compared four advanced convolutional neural networks: ResNet18, ResNet50, EfficientNet-B0, and DenseNet121, each enhanced with channel attention mechanisms. To address the inherent class imbalance, we developed a novel Combined Focal Label Smoothing Loss function that integrates focal loss, label smoothing, and class-balanced weighting. Our preprocessing pipeline incorporated advanced techniques, including contrast-limited adaptive histogram equalization (CLAHE) and comprehensive data augmentation. The individual models were combined through an optimized ensemble voting approach, achieving superior performance (AUC: 0.963, F1-score: 0.952) compared to any single model. This system demonstrates significant potential to standardize density assessments in clinical practice, potentially improving screening efficiency and early cancer detection rates while reducing inter-observer variability among radiologists.
* 2025 IEEE Nuclear Science Symposium, Medical Imaging Conference and
Room Temperature Semiconductor Detector Conference
Via
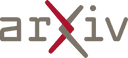
Jul 02, 2025
Abstract:Low-dose computed tomography (LDCT) imaging employed in lung cancer screening (LCS) programs is increasing in uptake worldwide. LCS programs herald a generational opportunity to simultaneously detect cancer and non-cancer-related early-stage lung disease. Yet these efforts are hampered by a shortage of radiologists to interpret scans at scale. Here, we present TANGERINE, a computationally frugal, open-source vision foundation model for volumetric LDCT analysis. Designed for broad accessibility and rapid adaptation, TANGERINE can be fine-tuned off the shelf for a wide range of disease-specific tasks with limited computational resources and training data. Relative to models trained from scratch, TANGERINE demonstrates fast convergence during fine-tuning, thereby requiring significantly fewer GPU hours, and displays strong label efficiency, achieving comparable or superior performance with a fraction of fine-tuning data. Pretrained using self-supervised learning on over 98,000 thoracic LDCTs, including the UK's largest LCS initiative to date and 27 public datasets, TANGERINE achieves state-of-the-art performance across 14 disease classification tasks, including lung cancer and multiple respiratory diseases, while generalising robustly across diverse clinical centres. By extending a masked autoencoder framework to 3D imaging, TANGERINE offers a scalable solution for LDCT analysis, departing from recent closed, resource-intensive models by combining architectural simplicity, public availability, and modest computational requirements. Its accessible, open-source lightweight design lays the foundation for rapid integration into next-generation medical imaging tools that could transform LCS initiatives, allowing them to pivot from a singular focus on lung cancer detection to comprehensive respiratory disease management in high-risk populations.
Via
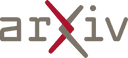
Jul 03, 2025
Abstract:Colorectal polyp segmentation is critical for early detection of colorectal cancer, yet weak and low contrast boundaries significantly limit automated accuracy. Existing deep models either blur fine edge details or rely on handcrafted filters that perform poorly under variable imaging conditions. We propose MEGANet-W, a Wavelet Driven Edge Guided Attention Network that injects directional, parameter free Haar wavelet edge maps into each decoder stage to recalibrate semantic features. Our two main contributions are: (1) a two-level Haar wavelet head for multi orientation edge extraction; and (2) Wavelet Edge Guided Attention (WEGA) modules that fuse wavelet cues with reverse and input branches. On five public polyp datasets, MEGANetW consistently outperforms existing methods, improving mIoU by up to 2.3% and mDice by 1.2%, while introducing no additional learnable parameters.
* 7 pages, 3 figures
Via
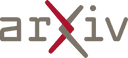
Jul 02, 2025
Abstract:Polyp segmentation in colonoscopy images is crucial for early detection and diagnosis of colorectal cancer. However, this task remains a significant challenge due to the substantial variations in polyp shape, size, and color, as well as the high similarity between polyps and surrounding tissues, often compounded by indistinct boundaries. While existing encoder-decoder CNN and transformer-based approaches have shown promising results, they struggle with stable segmentation performance on polyps with weak or blurry boundaries. These methods exhibit limited abilities to distinguish between polyps and non-polyps and capture essential boundary cues. Moreover, their generalizability still falls short of meeting the demands of real-time clinical applications. To address these limitations, we propose SAM-MaGuP, a groundbreaking approach for robust polyp segmentation. By incorporating a boundary distillation module and a 1D-2D Mamba adapter within the Segment Anything Model (SAM), SAM-MaGuP excels at resolving weak boundary challenges and amplifies feature learning through enriched global contextual interactions. Extensive evaluations across five diverse datasets reveal that SAM-MaGuP outperforms state-of-the-art methods, achieving unmatched segmentation accuracy and robustness. Our key innovations, a Mamba-guided boundary prior and a 1D-2D Mamba block, set a new benchmark in the field, pushing the boundaries of polyp segmentation to new heights.
* 11 pages, 2 figures, MICCAI-2025
Via
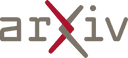
Jun 26, 2025
Abstract:Complete removal of cancer tumors with a negative specimen margin during lumpectomy is essential in reducing breast cancer recurrence. However, 2D specimen radiography (SR), the current method used to assess intraoperative specimen margin status, has limited accuracy, resulting in nearly a quarter of patients requiring additional surgery. To address this, we propose a novel deep learning framework combining the Segment Anything Model (SAM) with Forward-Forward Contrastive Learning (FFCL), a pre-training strategy leveraging both local and global contrastive learning for patch-level classification of SR images. After annotating SR images with regions of known maligancy, non-malignant tissue, and pathology-confirmed margins, we pre-train a ResNet-18 backbone with FFCL to classify margin status, then reconstruct coarse binary masks to prompt SAM for refined tumor margin segmentation. Our approach achieved an AUC of 0.8455 for margin classification and segmented margins with a 27.4% improvement in Dice similarity over baseline models, while reducing inference time to 47 milliseconds per image. These results demonstrate that FFCL-SAM significantly enhances both the speed and accuracy of intraoperative margin assessment, with strong potential to reduce re-excision rates and improve surgical outcomes in breast cancer treatment. Our code is available at https://github.com/tbwa233/FFCL-SAM/.
* 19 pages, 7 figures, 3 tables
Via
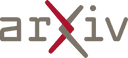
Jun 24, 2025
Abstract:Regular mammography screening is essential for early breast cancer detection. Deep learning-based risk prediction methods have sparked interest to adjust screening intervals for high-risk groups. While early methods focused only on current mammograms, recent approaches leverage the temporal aspect of screenings to track breast tissue changes over time, requiring spatial alignment across different time points. Two main strategies for this have emerged: explicit feature alignment through deformable registration and implicit learned alignment using techniques like transformers, with the former providing more control. However, the optimal approach for explicit alignment in mammography remains underexplored. In this study, we provide insights into where explicit alignment should occur (input space vs. representation space) and if alignment and risk prediction should be jointly optimized. We demonstrate that jointly learning explicit alignment in representation space while optimizing risk estimation performance, as done in the current state-of-the-art approach, results in a trade-off between alignment quality and predictive performance and show that image-level alignment is superior to representation-level alignment, leading to better deformation field quality and enhanced risk prediction accuracy. The code is available at https://github.com/sot176/Longitudinal_Mammogram_Alignment.git.
* MICCAI 2025, early accepted
Via
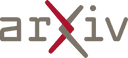
Jun 26, 2025
Abstract:According to the data, the percent of women who underwent screening for cervical cancer, breast and oral cancer in Telangana in the year 2020 was 3.3 percent, 0.3 percent and 2.3 percent respectively. Although early detection is the only way to reduce morbidity and mortality, people have very low awareness about cervical and breast cancer signs and symptoms and screening practices. We developed an ML classification model to predict if a person is susceptible to breast or cervical cancer based on demographic factors. We devised a system to provide suggestions for the nearest hospital or Cancer treatment centres based on the users location or address. In addition to this, we can integrate the health card to maintain medical records of all individuals and conduct awareness drives and campaigns. For ML classification models, we used decision tree classification and support vector classification algorithms for cervical cancer susceptibility and breast cancer susceptibility respectively. Thus, by devising this solution we come one step closer to our goal which is spreading cancer awareness, thereby, decreasing the cancer mortality and increasing cancer literacy among the people of Telangana.
Via
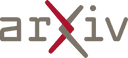
Jun 24, 2025
Abstract:The segmentation of metastatic bone disease (MBD) in whole-body MRI (WB-MRI) is a challenging problem. Due to varying appearances and anatomical locations of lesions, ambiguous boundaries, and severe class imbalance, obtaining reliable segmentations requires large, well-annotated datasets capturing lesion variability. Generating such datasets requires substantial time and expertise, and is prone to error. While self-supervised learning (SSL) can leverage large unlabeled datasets, learned generic representations often fail to capture the nuanced features needed for accurate lesion detection. In this work, we propose a Supervised Anatomical Pretraining (SAP) method that learns from a limited dataset of anatomical labels. First, an MRI-based skeletal segmentation model is developed and trained on WB-MRI scans from healthy individuals for high-quality skeletal delineation. Then, we compare its downstream efficacy in segmenting MBD on a cohort of 44 patients with metastatic prostate cancer, against both a baseline random initialization and a state-of-the-art SSL method. SAP significantly outperforms both the baseline and SSL-pretrained models, achieving a normalized surface Dice of 0.76 and a Dice coefficient of 0.64. The method achieved a lesion detection F2 score of 0.44, improving on 0.24 (baseline) and 0.31 (SSL). When considering only clinically relevant lesions larger than 1~ml, SAP achieves a detection sensitivity of 100% in 28 out of 32 patients. Learning bone morphology from anatomy yields an effective and domain-relevant inductive bias that can be leveraged for the downstream segmentation task of bone lesions. All code and models are made publicly available.
* This preprint is currently under review at *Computers in Biology and
Medicine* (Elsevier). This version has not been peer-reviewed
Via
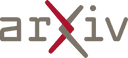