What is cancer detection? Cancer detection using Artificial Intelligence (AI) involves leveraging advanced machine learning algorithms and techniques to identify and diagnose cancer from various medical data sources. The goal is to enhance early detection, improve diagnostic accuracy, and potentially reduce the need for invasive procedures.
Papers and Code
Jun 18, 2025
Abstract:Cervical cancer remains a significant health problem, especially in developing countries. Early detection is critical for effective treatment. Convolutional neural networks (CNN) have shown promise in automated cervical cancer screening, but their performance depends on Pap smear image quality. This study investigates the impact of various image preprocessing techniques on CNN performance for cervical cancer classification using the SIPaKMeD dataset. Three preprocessing techniques were evaluated: perona-malik diffusion (PMD) filter for noise reduction, contrast-limited adaptive histogram equalization (CLAHE) for image contrast enhancement, and the proposed hybrid PMD filter-CLAHE approach. The enhanced image datasets were evaluated on pretrained models, such as ResNet-34, ResNet-50, SqueezeNet-1.0, MobileNet-V2, EfficientNet-B0, EfficientNet-B1, DenseNet-121, and DenseNet-201. The results show that hybrid preprocessing PMD filter-CLAHE can improve the Pap smear image quality and CNN architecture performance compared to the original images. The maximum metric improvements are 13.62% for accuracy, 10.04% for precision, 13.08% for recall, and 14.34% for F1-score. The proposed hybrid PMD filter-CLAHE technique offers a new perspective in improving cervical cancer classification performance using CNN architectures.
Via
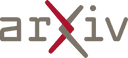
Jun 10, 2025
Abstract:Recent discoveries have suggested that the promising avenue of using circulating tumor DNA (ctDNA) levels in blood samples provides reasonable accuracy for cancer monitoring, with extremely low burden on the patient's side. It is known that the presence of ctDNA can result from various mechanisms leading to DNA release from cells, such as apoptosis, necrosis or active secretion. One key idea in recent cancer monitoring studies is that monitoring the dynamics of ctDNA levels might be sufficient for early multi-cancer detection. This interesting idea has been turned into commercial products, e.g. in the company named GRAIL. In the present work, we propose to explore the use of Signature theory for detecting aggressive cancer tumors based on the analysis of blood samples. Our approach combines tools from continuous time Markov modelling for the dynamics of ctDNA levels in the blood, with Signature theory for building efficient testing procedures. Signature theory is a topic of growing interest in the Machine Learning community (see Chevyrev2016 and Fermanian2021), which is now recognised as a powerful feature extraction tool for irregularly sampled signals. The method proposed in the present paper is shown to correctly address the challenging problem of overcoming the inherent data scarsity due to the extremely small number of blood samples per patient. The relevance of our approach is illustrated with extensive numerical experiments that confirm the efficiency of the proposed pipeline.
* Under review
Via
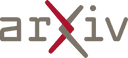
Jun 11, 2025
Abstract:Oral squamous cell carcinoma OSCC is a major global health burden, particularly in several regions across Asia, Africa, and South America, where it accounts for a significant proportion of cancer cases. Early detection dramatically improves outcomes, with stage I cancers achieving up to 90 percent survival. However, traditional diagnosis based on histopathology has limited accessibility in low-resource settings because it is invasive, resource-intensive, and reliant on expert pathologists. On the other hand, oral cytology of brush biopsy offers a minimally invasive and lower cost alternative, provided that the remaining challenges, inter observer variability and unavailability of expert pathologists can be addressed using artificial intelligence. Development and validation of robust AI solutions requires access to large, labeled, and multi-source datasets to train high capacity models that generalize across domain shifts. We introduce the first large and multicenter oral cytology dataset, comprising annotated slides stained with Papanicolaou(PAP) and May-Grunwald-Giemsa(MGG) protocols, collected from ten tertiary medical centers in India. The dataset is labeled and annotated by expert pathologists for cellular anomaly classification and detection, is designed to advance AI driven diagnostic methods. By filling the gap in publicly available oral cytology datasets, this resource aims to enhance automated detection, reduce diagnostic errors, and improve early OSCC diagnosis in resource-constrained settings, ultimately contributing to reduced mortality and better patient outcomes worldwide.
* 7 pages, 2 figurs
Via
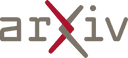
Jun 13, 2025
Abstract:Breast cancer remains a leading cause of cancer-related mortality worldwide, making early detection and accurate treatment response monitoring critical priorities. We present BreastDCEDL, a curated, deep learning-ready dataset comprising pre-treatment 3D Dynamic Contrast-Enhanced MRI (DCE-MRI) scans from 2,070 breast cancer patients drawn from the I-SPY1, I-SPY2, and Duke cohorts, all sourced from The Cancer Imaging Archive. The raw DICOM imaging data were rigorously converted into standardized 3D NIfTI volumes with preserved signal integrity, accompanied by unified tumor annotations and harmonized clinical metadata including pathologic complete response (pCR), hormone receptor (HR), and HER2 status. Although DCE-MRI provides essential diagnostic information and deep learning offers tremendous potential for analyzing such complex data, progress has been limited by lack of accessible, public, multicenter datasets. BreastDCEDL addresses this gap by enabling development of advanced models, including state-of-the-art transformer architectures that require substantial training data. To demonstrate its capacity for robust modeling, we developed the first transformer-based model for breast DCE-MRI, leveraging Vision Transformer (ViT) architecture trained on RGB-fused images from three contrast phases (pre-contrast, early post-contrast, and late post-contrast). Our ViT model achieved state-of-the-art pCR prediction performance in HR+/HER2- patients (AUC 0.94, accuracy 0.93). BreastDCEDL includes predefined benchmark splits, offering a framework for reproducible research and enabling clinically meaningful modeling in breast cancer imaging.
Via
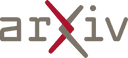
Jun 06, 2025
Abstract:Spiking Neural Networks (SNNs) event-driven nature enables efficient encoding of spatial and temporal features, making them suitable for dynamic time-dependent data processing. Despite their biological relevance, SNNs have seen limited application in medical image recognition due to difficulties in matching the performance of conventional deep learning models. To address this, we propose a novel breast cancer classification approach that combines SNNs with Lempel-Ziv Complexity (LZC) a computationally efficient measure of sequence complexity. LZC enhances the interpretability and accuracy of spike-based models by capturing structural patterns in neural activity. Our study explores both biophysical Leaky Integrate-and-Fire (LIF) and probabilistic Levy-Baxter (LB) neuron models under supervised, unsupervised, and hybrid learning regimes. Experiments were conducted on the Breast Cancer Wisconsin dataset using numerical features derived from medical imaging. LB-based models consistently exceeded 90.00% accuracy, while LIF-based models reached over 85.00%. The highest accuracy of 98.25% was achieved using an ANN-to-SNN conversion method applied to both neuron models comparable to traditional deep learning with back-propagation, but at up to 100 times lower computational cost. This hybrid approach merges deep learning performance with the efficiency and plausibility of SNNs, yielding top results at lower computational cost. We hypothesize that the synergy between temporal-coding, spike-sparsity, and LZC-driven complexity analysis enables more-efficient feature extraction. Our findings demonstrate that SNNs combined with LZC offer promising, biologically plausible alternative to conventional neural networks in medical diagnostics, particularly for resource-constrained or real-time systems.
Via
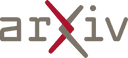
Jun 10, 2025
Abstract:Mammography is the gold standard for the detection and diagnosis of breast cancer. This procedure can be significantly enhanced with Artificial Intelligence (AI)-based software, which assists radiologists in identifying abnormalities. However, training AI systems requires large and diverse datasets, which are often difficult to obtain due to privacy and ethical constraints. To address this issue, the paper introduces MAMmography ensemBle mOdel (MAMBO), a novel patch-based diffusion approach designed to generate full-resolution mammograms. Diffusion models have shown breakthrough results in realistic image generation, yet few studies have focused on mammograms, and none have successfully generated high-resolution outputs required to capture fine-grained features of small lesions. To achieve this, MAMBO integrates separate diffusion models to capture both local and global (image-level) contexts. The contextual information is then fed into the final patch-based model, significantly aiding the noise removal process. This thoughtful design enables MAMBO to generate highly realistic mammograms of up to 3840x3840 pixels. Importantly, this approach can be used to enhance the training of classification models and extended to anomaly detection. Experiments, both numerical and radiologist validation, assess MAMBO's capabilities in image generation, super-resolution, and anomaly detection, highlighting its potential to enhance mammography analysis for more accurate diagnoses and earlier lesion detection.
* 21 pages, 14 figures, 7 tables
Via
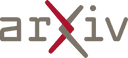
Jun 11, 2025
Abstract:Oral cancer presents a formidable challenge in oncology, necessitating early diagnosis and accurate prognosis to enhance patient survival rates. Recent advancements in machine learning and data mining have revolutionized traditional diagnostic methodologies, providing sophisticated and automated tools for differentiating between benign and malignant oral lesions. This study presents a comprehensive review of cutting-edge data mining methodologies, including Neural Networks, K-Nearest Neighbors (KNN), Support Vector Machines (SVM), and ensemble learning techniques, specifically applied to the diagnosis and prognosis of oral cancer. Through a rigorous comparative analysis, our findings reveal that Neural Networks surpass other models, achieving an impressive classification accuracy of 93,6 % in predicting oral cancer. Furthermore, we underscore the potential benefits of integrating feature selection and dimensionality reduction techniques to enhance model performance. These insights underscore the significant promise of advanced data mining techniques in bolstering early detection, optimizing treatment strategies, and ultimately improving patient outcomes in the realm of oral oncology.
* Data and Metadata [Internet]. 2025 Jan. 2 [cited 2025 Jun.
11];3:.570
Via
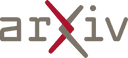
Jun 12, 2025
Abstract:Quality assurance is a critical but underexplored area in digital pathology, where even minor artifacts can have significant effects. Artifacts have been shown to negatively impact the performance of AI diagnostic models. In current practice, trained staff manually review digitized images prior to release of these slides to pathologists which are then used to render a diagnosis. Conventional image processing approaches, provide a foundation for detecting artifacts on digital pathology slides. However, current tools do not leverage deep learning, which has the potential to improve detection accuracy and scalability. Despite these advancements, methods for quality assurance in digital pathology remain limited, presenting a gap for innovation. We propose an AI algorithm designed to screen digital pathology slides by analyzing tiles and categorizing them into one of 10 predefined artifact types or as background. This algorithm identifies and localizes artifacts, creating a map that highlights regions of interest. By directing human operators to specific tiles affected by artifacts, the algorithm minimizes the time and effort required to manually review entire slides for quality issues. From internal archives and The Cancer Genome Atlas, 133 whole slide images were selected and 10 artifacts were annotated using an internally developed software ZAPP (Mayo Clinic, Jacksonville, FL). Ablation study of multiple models at different tile sizes and magnification was performed. InceptionResNet was selected. Single artifact models were trained and tested, followed by a limited multiple instance model with artifacts that performed well together (chatter, fold, and pen). From the results of this study we suggest a hybrid design for artifact screening composed of both single artifact binary models as well as multiple instance models to optimize detection of each artifact.
Via
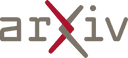
Jun 04, 2025
Abstract:Over the past decade, Medical Image Segmentation (MIS) using Deep Neural Networks (DNNs) has achieved significant performance improvements and holds great promise for future developments. This paper presents a comprehensive study on MIS based on DNNs. Intelligent Vision Systems are often evaluated based on their output levels, such as Data, Information, Knowledge, Intelligence, and Wisdom (DIKIW),and the state-of-the-art solutions in MIS at these levels are the focus of research. Additionally, Explainable Artificial Intelligence (XAI) has become an important research direction, as it aims to uncover the "black box" nature of previous DNN architectures to meet the requirements of transparency and ethics. The study emphasizes the importance of MIS in disease diagnosis and early detection, particularly for increasing the survival rate of cancer patients through timely diagnosis. XAI and early prediction are considered two important steps in the journey from "intelligence" to "wisdom." Additionally, the paper addresses existing challenges and proposes potential solutions to enhance the efficiency of implementing DNN-based MIS.
* International Journal of Advanced Computer Science and
Applications(IJACSA), 14(3), 2023
Via
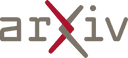
Jun 08, 2025
Abstract:Lung cancer remains one of the most prevalent and fatal diseases worldwide, demanding accurate and timely diagnosis and treatment. Recent advancements in large AI models have significantly enhanced medical image understanding and clinical decision-making. This review systematically surveys the state-of-the-art in applying large AI models to lung cancer screening, diagnosis, prognosis, and treatment. We categorize existing models into modality-specific encoders, encoder-decoder frameworks, and joint encoder architectures, highlighting key examples such as CLIP, BLIP, Flamingo, BioViL-T, and GLoRIA. We further examine their performance in multimodal learning tasks using benchmark datasets like LIDC-IDRI, NLST, and MIMIC-CXR. Applications span pulmonary nodule detection, gene mutation prediction, multi-omics integration, and personalized treatment planning, with emerging evidence of clinical deployment and validation. Finally, we discuss current limitations in generalizability, interpretability, and regulatory compliance, proposing future directions for building scalable, explainable, and clinically integrated AI systems. Our review underscores the transformative potential of large AI models to personalize and optimize lung cancer care.
* Under Review
Via
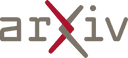