Topic:Time Series Analysis
What is Time Series Analysis? Time series analysis comprises statistical methods for analyzing a sequence of data points collected over an interval of time to identify interesting patterns and trends.
Papers and Code
May 09, 2025
Abstract:Irregular temporal data, characterized by varying recording frequencies, differing observation durations, and missing values, presents significant challenges across fields like mobility, healthcare, and environmental science. Existing research communities often overlook or address these challenges in isolation, leading to fragmented tools and methods. To bridge this gap, we introduce a unified framework, and the first standardized dataset repository for irregular time series classification, built on a common array format to enhance interoperability. This repository comprises 34 datasets on which we benchmark 12 classifier models from diverse domains and communities. This work aims to centralize research efforts and enable a more robust evaluation of irregular temporal data analysis methods.
Via
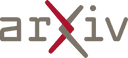
May 04, 2025
Abstract:Multivariate long-term time series forecasting is critical for applications such as weather prediction, and traffic analysis. In addition, the implementation of Transformer variants has improved prediction accuracy. Following these variants, different input data process approaches also enhanced the field, such as tokenization techniques including point-wise, channel-wise, and patch-wise tokenization. However, previous studies still have limitations in time complexity, computational resources, and cross-dimensional interactions. To address these limitations, we introduce a novel CNN Autoencoder-based Score Attention mechanism (CASA), which can be introduced in diverse Transformers model-agnosticically by reducing memory and leading to improvement in model performance. Experiments on eight real-world datasets validate that CASA decreases computational resources by up to 77.7%, accelerates inference by 44.0%, and achieves state-of-the-art performance, ranking first in 87.5% of evaluated metrics.
Via
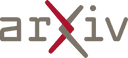
May 14, 2025
Abstract:Hamilton-Jacobi Reachability (HJR) analysis has been successfully used in many robotics and control tasks, and is especially effective in computing reach-avoid sets and control laws that enable an agent to reach a goal while satisfying state constraints. However, the original HJR formulation provides no guarantees of safety after a) the prescribed time horizon, or b) goal satisfaction. The reach-avoid-stabilize (RAS) problem has therefore gained a lot of focus: find the set of initial states (the RAS set), such that the trajectory can reach the target, and stabilize to some point of interest (POI) while avoiding obstacles. Solving RAS problems using HJR usually requires defining a new value function, whose zero sub-level set is the RAS set. The existing methods do not consider the problem when there are a series of targets to reach and/or obstacles to avoid. We propose a method that uses the idea of admissible control sets; we guarantee that the system will reach each target while avoiding obstacles as prescribed by the given time series. Moreover, we guarantee that the trajectory ultimately stabilizes to the POI. The proposed method provides an under-approximation of the RAS set, guaranteeing safety. Numerical examples are provided to validate the theory.
* 7 pages, 5 figures, submitted to 64th IEEE Conference on Decision and
Control
Via
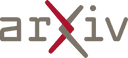
May 10, 2025
Abstract:Attention mechanisms are widely used in artificial intelligence to enhance performance and interpretability. In this paper, we investigate their utility in modeling classical dynamical systems -- specifically, a noisy predator-prey (Lotka-Volterra) system. We train a simple linear attention model on perturbed time-series data to reconstruct system trajectories. Remarkably, the learned attention weights align with the geometric structure of the Lyapunov function: high attention corresponds to flat regions (where perturbations have small effect), and low attention aligns with steep regions (where perturbations have large effect). We further demonstrate that attention-based weighting can serve as a proxy for sensitivity analysis, capturing key phase-space properties without explicit knowledge of the system equations. These results suggest a novel use of AI-derived attention for interpretable, data-driven analysis and control of nonlinear systems. For example our framework could support future work in biological modeling of circadian rhythms, and interpretable machine learning for dynamical environments.
* 5 figures, 12 pages, python code included
Via
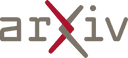
May 11, 2025
Abstract:Recent advances in artificial intelligence have highlighted the remarkable capabilities of neural network (NN)-powered systems on classical computers. However, these systems face significant computational challenges that limit scalability and efficiency. Quantum computers hold the potential to overcome these limitations and increase processing power beyond classical systems. Despite this, integrating quantum computing with NNs remains largely unrealized due to challenges posed by noise, decoherence, and high error rates in current quantum hardware. Here, we propose a novel quantum echo-state network (QESN) design and implementation algorithm that can operate within the presence of noise on current IBM hardware. We apply classical control-theoretic response analysis to characterize the QESN, emphasizing its rich nonlinear dynamics and memory, as well as its ability to be fine-tuned with sparsity and re-uploading blocks. We validate our approach through a comprehensive demonstration of QESNs functioning as quantum observers, applied in both high-fidelity simulations and hardware experiments utilizing data from a prototypical chaotic Lorenz system. Our results show that the QESN can predict long time-series with persistent memory, running over 100 times longer than the median T}1 and T2 of the IBM Marrakesh QPU, achieving state-of-the-art time-series performance on superconducting hardware.
* 14 pages, 12 figures
Via
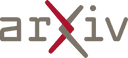
May 12, 2025
Abstract:Large Reasoning Models (LRMs) have the ability to self-correct even when they make mistakes in their reasoning paths. However, our study reveals that when the reasoning process starts with a short but poor beginning, it becomes difficult for the model to recover. We refer to this phenomenon as the "Prefix Dominance Trap". Inspired by psychological findings that peer interaction can promote self-correction without negatively impacting already accurate individuals, we propose **Learning from Peers** (LeaP) to address this phenomenon. Specifically, every tokens, each reasoning path summarizes its intermediate reasoning and shares it with others through a routing mechanism, enabling paths to incorporate peer insights during inference. However, we observe that smaller models sometimes fail to follow summarization and reflection instructions effectively. To address this, we fine-tune them into our **LeaP-T** model series. Experiments on AIME 2024, AIME 2025, AIMO 2025, and GPQA Diamond show that LeaP provides substantial improvements. For instance, QwQ-32B with LeaP achieves nearly 5 absolute points higher than the baseline on average, and surpasses DeepSeek-R1-671B on three math benchmarks with an average gain of 3.3 points. Notably, our fine-tuned LeaP-T-7B matches the performance of DeepSeek-R1-Distill-Qwen-14B on AIME 2024. In-depth analysis reveals LeaP's robust error correction by timely peer insights, showing strong error tolerance and handling varied task difficulty. LeaP marks a milestone by enabling LRMs to collaborate during reasoning. Our code, datasets, and models are available at https://learning-from-peers.github.io/ .
* 29 pages, 32 figures
Via
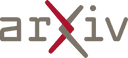
May 05, 2025
Abstract:Delirium represents a significant clinical concern characterized by high morbidity and mortality rates, particularly in patients with mild cognitive impairment (MCI). This study investigates the associated risk factors for delirium by analyzing the comorbidity patterns relevant to MCI and developing a longitudinal predictive model leveraging machine learning methodologies. A retrospective analysis utilizing the MIMIC-IV v2.2 database was performed to evaluate comorbid conditions, survival probabilities, and predictive modeling outcomes. The examination of comorbidity patterns identified distinct risk profiles for the MCI population. Kaplan-Meier survival analysis demonstrated that individuals with MCI exhibit markedly reduced survival probabilities when developing delirium compared to their non-MCI counterparts, underscoring the heightened vulnerability within this cohort. For predictive modeling, a Long Short-Term Memory (LSTM) ML network was implemented utilizing time-series data, demographic variables, Charlson Comorbidity Index (CCI) scores, and an array of comorbid conditions. The model demonstrated robust predictive capabilities with an AUROC of 0.93 and an AUPRC of 0.92. This study underscores the critical role of comorbidities in evaluating delirium risk and highlights the efficacy of time-series predictive modeling in pinpointing patients at elevated risk for delirium development.
Via
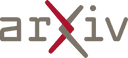
May 01, 2025
Abstract:Understanding how auditory stimuli influence emotional and physiological states is fundamental to advancing affective computing and mental health technologies. In this paper, we present a multimodal evaluation of the affective and physiological impacts of three auditory conditions, that is, spiritual meditation (SM), music (M), and natural silence (NS), using a comprehensive suite of biometric signal measures. To facilitate this analysis, we introduce the Spiritual, Music, Silence Acoustic Time Series (SMSAT) dataset, a novel benchmark comprising acoustic time series (ATS) signals recorded under controlled exposure protocols, with careful attention to demographic diversity and experimental consistency. To model the auditory induced states, we develop a contrastive learning based SMSAT audio encoder that extracts highly discriminative embeddings from ATS data, achieving 99.99% classification accuracy in interclass and intraclass evaluations. Furthermore, we propose the Calmness Analysis Model (CAM), a deep learning framework integrating 25 handcrafted and learned features for affective state classification across auditory conditions, attaining robust 99.99% classification accuracy. In contrast, pairwise t tests reveal significant deviations in cardiac response characteristics (CRC) between SM analysis via ANOVA inducing more significant physiological fluctuations. Compared to existing state of the art methods reporting accuracies up to 90%, the proposed model demonstrates substantial performance gains (up to 99%). This work contributes a validated multimodal dataset and a scalable deep learning framework for affective computing applications in stress monitoring, mental well-being, and therapeutic audio-based interventions.
Via
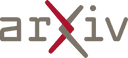
Apr 26, 2025
Abstract:The present study explores the interpretability of latent spaces produced by time series foundation models, focusing on their potential for visual analysis tasks. Specifically, we evaluate the MOMENT family of models, a set of transformer-based, pre-trained architectures for multivariate time series tasks such as: imputation, prediction, classification, and anomaly detection. We evaluate the capacity of these models on five datasets to capture the underlying structures in time series data within their latent space projection and validate whether fine tuning improves the clarity of the resulting embedding spaces. Notable performance improvements in terms of loss reduction were observed after fine tuning. Visual analysis shows limited improvement in the interpretability of the embeddings, requiring further work. Results suggest that, although Time Series Foundation Models such as MOMENT are robust, their latent spaces may require additional methodological refinements to be adequately interpreted, such as alternative projection techniques, loss functions, or data preprocessing strategies. Despite the limitations of MOMENT, foundation models supose a big reduction in execution time and so a great advance for interactive visual analytics.
* Currently under review at the International Journal of Interactive
Multimedia and Artificial Intelligence (IJIMAI)
Via
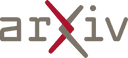
May 02, 2025
Abstract:Predicting the price that has the least error and can provide the best and highest accuracy has been one of the most challenging issues and one of the most critical concerns among capital market activists and researchers. Therefore, a model that can solve problems and provide results with high accuracy is one of the topics of interest among researchers. In this project, using time series prediction models such as ARIMA to estimate the price, variables, and indicators related to technical analysis show the behavior of traders involved in involving psychological factors for the model. By linking all of these variables to stepwise regression, we identify the best variables influencing the prediction of the variable. Finally, we enter the selected variables as inputs to the artificial neural network. In other words, we want to call this whole prediction process the "ARIMA_Stepwise Regression_Neural Network" model and try to predict the price of gold in international financial markets. This approach is expected to be able to be used to predict the types of stocks, commodities, currency pairs, financial market indicators, and other items used in local and international financial markets. Moreover, a comparison between the results of this method and time series methods is also expressed. Finally, based on the results, it can be seen that the resulting hybrid model has the highest accuracy compared to the time series method, regression, and stepwise regression.
Via
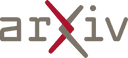