What is Sentiment Analysis? Sentiment analysis is the process of determining the sentiment of a piece of text, such as a tweet or a review.
Papers and Code
May 22, 2025
Abstract:As of 2025, Generative Artificial Intelligence (GenAI) has become a central tool for productivity across industries. Beyond text generation, GenAI now plays a critical role in coding, data analysis, and research workflows. As large language models (LLMs) continue to evolve, it is essential to assess the reliability and accuracy of their outputs, especially in specialized, high-stakes domains like finance. Most modern LLMs transform text into numerical vectors, which are used in operations such as cosine similarity searches to generate responses. However, this abstraction process can lead to misinterpretation of emotional tone, particularly in nuanced financial contexts. While LLMs generally excel at identifying sentiment in everyday language, these models often struggle with the nuanced, strategically ambiguous language found in earnings call transcripts. Financial disclosures frequently embed sentiment in hedged statements, forward-looking language, and industry-specific jargon, making it difficult even for human analysts to interpret consistently, let alone AI models. This paper presents findings from the Santa Clara Microsoft Practicum Project, led by Professor Charlie Goldenberg, which benchmarks the performance of Microsoft's Copilot, OpenAI's ChatGPT, Google's Gemini, and traditional machine learning models for sentiment analysis of financial text. Using Microsoft earnings call transcripts, the analysis assesses how well LLM-derived sentiment correlates with market sentiment and stock movements and evaluates the accuracy of model outputs. Prompt engineering techniques are also examined to improve sentiment analysis results. Visualizations of sentiment consistency are developed to evaluate alignment between tone and stock performance, with sentiment trends analyzed across Microsoft's lines of business to determine which segments exert the greatest influence.
* 6 pages, 4 figures. Research conducted as part of a
Microsoft-sponsored Capstone Project at Santa Clara University
Via
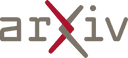
May 20, 2025
Abstract:There has been growing interest in Multimodal Aspect-Based Sentiment Analysis (MABSA) in recent years. Existing methods predominantly rely on pre-trained small language models (SLMs) to collect information related to aspects and sentiments from both image and text, with an aim to align these two modalities. However, small SLMs possess limited capacity and knowledge, often resulting in inaccurate identification of meaning, aspects, sentiments, and their interconnections in textual and visual data. On the other hand, Large language models (LLMs) have shown exceptional capabilities in various tasks by effectively exploring fine-grained information in multimodal data. However, some studies indicate that LLMs still fall short compared to fine-tuned small models in the field of ABSA. Based on these findings, we propose a novel framework, termed LRSA, which combines the decision-making capabilities of SLMs with additional information provided by LLMs for MABSA. Specifically, we inject explanations generated by LLMs as rationales into SLMs and employ a dual cross-attention mechanism for enhancing feature interaction and fusion, thereby augmenting the SLMs' ability to identify aspects and sentiments. We evaluated our method using two baseline models, numerous experiments highlight the superiority of our approach on three widely-used benchmarks, indicating its generalizability and applicability to most pre-trained models for MABSA.
Via
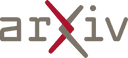
May 23, 2025
Abstract:As Large Language Models (LLMs) are increasingly being adopted for narrow tasks - such as medical question answering or sentiment analysis - and deployed in resource-constrained settings, a key question arises: how many parameters does a task actually need? In this work, we present LLM-Sieve, the first comprehensive framework for task-specific pruning of LLMs that achieves 20-75% parameter reduction with only 1-5% accuracy degradation across diverse domains. Unlike prior methods that apply uniform pruning or rely on low-rank approximations of weight matrices or inputs in isolation, LLM-Sieve (i) learns task-aware joint projections to better approximate output behavior, and (ii) employs a Genetic Algorithm to discover differentiated pruning levels for each matrix. LLM-Sieve is fully compatible with LoRA fine-tuning and quantization, and uniquely demonstrates strong generalization across datasets within the same task domain. Together, these results establish a practical and robust mechanism to generate smaller performant task-specific models.
Via
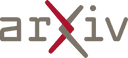
May 19, 2025
Abstract:Classical Chinese poetry is a vital and enduring part of Chinese literature, conveying profound emotional resonance. Existing studies analyze sentiment based on textual meanings, overlooking the unique rhythmic and visual features inherent in poetry,especially since it is often recited and accompanied by Chinese paintings. In this work, we propose a dialect-enhanced multimodal framework for classical Chinese poetry sentiment analysis. We extract sentence-level audio features from the poetry and incorporate audio from multiple dialects,which may retain regional ancient Chinese phonetic features, enriching the phonetic representation. Additionally, we generate sentence-level visual features, and the multimodal features are fused with textual features enhanced by LLM translation through multimodal contrastive representation learning. Our framework outperforms state-of-the-art methods on two public datasets, achieving at least 2.51% improvement in accuracy and 1.63% in macro F1. We open-source the code to facilitate research in this area and provide insights for general multimodal Chinese representation.
Via
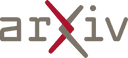
May 22, 2025
Abstract:In this paper, we combine two-step knowledge distillation, structured pruning, truncation, and vocabulary trimming for extremely compressing multilingual encoder-only language models for low-resource languages. Our novel approach systematically combines existing techniques and takes them to the extreme, reducing layer depth, feed-forward hidden size, and intermediate layer embedding size to create significantly smaller monolingual models while retaining essential language-specific knowledge. We achieve compression rates of up to 92% with only a marginal performance drop of 2-10% in four downstream tasks, including sentiment analysis, topic classification, named entity recognition, and part-of-speech tagging, across three low-resource languages. Notably, the performance degradation correlates with the amount of language-specific data in the teacher model, with larger datasets resulting in smaller performance losses. Additionally, we conduct extensive ablation studies to identify best practices for multilingual model compression using these techniques.
* Pre-print
Via
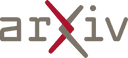
May 22, 2025
Abstract:The advancements in Multimodal Large Language Models (MLLMs) have enabled various multimodal tasks to be addressed under a zero-shot paradigm. This paradigm sidesteps the cost of model fine-tuning, emerging as a dominant trend in practical application. Nevertheless, Multimodal Sentiment Analysis (MSA), a pivotal challenge in the quest for general artificial intelligence, fails to accommodate this convenience. The zero-shot paradigm exhibits undesirable performance on MSA, casting doubt on whether MLLMs can perceive sentiments as competent as supervised models. By extending the zero-shot paradigm to In-Context Learning (ICL) and conducting an in-depth study on configuring demonstrations, we validate that MLLMs indeed possess such capability. Specifically, three key factors that cover demonstrations' retrieval, presentation, and distribution are comprehensively investigated and optimized. A sentimental predictive bias inherent in MLLMs is also discovered and later effectively counteracted. By complementing each other, the devised strategies for three factors result in average accuracy improvements of 15.9% on six MSA datasets against the zero-shot paradigm and 11.2% against the random ICL baseline.
Via
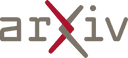
May 26, 2025
Abstract:Political biases encoded by LLMs might have detrimental effects on downstream applications. Existing bias analysis methods rely on small-size intermediate tasks (questionnaire answering or political content generation) and rely on the LLMs themselves for analysis, thus propagating bias. We propose a new approach leveraging the observation that LLM sentiment predictions vary with the target entity in the same sentence. We define an entropy-based inconsistency metric to encode this prediction variability. We insert 1319 demographically and politically diverse politician names in 450 political sentences and predict target-oriented sentiment using seven models in six widely spoken languages. We observe inconsistencies in all tested combinations and aggregate them in a statistically robust analysis at different granularity levels. We observe positive and negative bias toward left and far-right politicians and positive correlations between politicians with similar alignment. Bias intensity is higher for Western languages than for others. Larger models exhibit stronger and more consistent biases and reduce discrepancies between similar languages. We partially mitigate LLM unreliability in target-oriented sentiment classification (TSC) by replacing politician names with fictional but plausible counterparts.
* To be published in the Proceedings of the 63rd Annual Meeting of the
Association for Computational Linguistics (ACL 2025)
Via
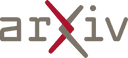
May 21, 2025
Abstract:Sarcasm is a challenge to sentiment analysis because of the incongruity between stated and implied sentiment. The challenge is exacerbated when the implication may be relevant to a specific country or geographical region. Pragmatic metacognitive prompting (PMP) is a cognition-inspired technique that has been used for pragmatic reasoning. In this paper, we harness PMP for explainable sarcasm detection for Australian and Indian English, alongside a benchmark dataset for standard English. We manually add sarcasm explanations to an existing sarcasm-labeled dataset for Australian and Indian English called BESSTIE, and compare the performance for explainable sarcasm detection for them with FLUTE, a standard English dataset containing sarcasm explanations. Our approach utilising PMP when evaluated on two open-weight LLMs (GEMMA and LLAMA) achieves statistically significant performance improvement across all tasks and datasets when compared with four alternative prompting strategies. We also find that alternative techniques such as agentic prompting mitigate context-related failures by enabling external knowledge retrieval. The focused contribution of our work is utilising PMP in generating sarcasm explanations for varieties of English.
* Under review. 4 pages + references
Via
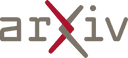
May 17, 2025
Abstract:In today's digitally-driven world, the demand for personalized and context-aware recommendations has never been greater. Traditional recommender systems have made significant strides in this direction, but they often lack the ability to tap into the richness of conversational data. This paper represents a novel approach to recommendation systems by integrating conversational insights into the recommendation process. The Conversational Recommender System integrates cutting-edge technologies such as deep learning, leveraging machine learning algorithms like Apriori for Association Rule Mining, Convolutional Neural Networks (CNN), Recurrent Neural Networks (RNN), and Long Short-Term Memory (LTSM). Furthermore, sophisticated voice recognition technologies, including Hidden Markov Models (HMMs) and Dynamic Time Warping (DTW) algorithms, play a crucial role in accurate speech-to-text conversion, ensuring robust performance in diverse environments. The methodology incorporates a fusion of content-based and collaborative recommendation approaches, enhancing them with NLP techniques. This innovative integration ensures a more personalized and context-aware recommendation experience, particularly in marketing applications.
* Presented in ISETE conference (International Conference on Artificial
Intelligence, Machine Learning and Big Data Engineering 2024)
Via
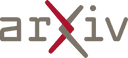
May 15, 2025
Abstract:This paper explores the design of an aspect-based sentiment analysis system using large language models (LLMs) for real-world use. We focus on quadruple opinion extraction -- identifying aspect categories, sentiment polarity, targets, and opinion expressions from text data across different domains and languages. Using internal datasets, we investigate whether a single fine-tuned model can effectively handle multiple domain-specific taxonomies simultaneously. We demonstrate that a combined multi-domain model achieves performance comparable to specialized single-domain models while reducing operational complexity. We also share lessons learned for handling non-extractive predictions and evaluating various failure modes when developing LLM-based systems for structured prediction tasks.
Via
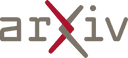