What is Recommendation? Recommendation is the task of providing personalized suggestions to users based on their preferences and behavior.
Papers and Code
Jun 26, 2025
Abstract:In real-world applications, users always interact with items in multiple aspects, such as through implicit binary feedback (e.g., clicks, dislikes, long views) and explicit feedback (e.g., comments, reviews). Modern recommendation systems (RecSys) learn user-item collaborative signals from these implicit feedback signals as a large-scale binary data-streaming, subsequently recommending other highly similar items based on users' personalized historical interactions. However, from this collaborative-connection perspective, the RecSys does not focus on the actual content of the items themselves but instead prioritizes higher-probability signals of behavioral co-occurrence among items. Consequently, under this binary learning paradigm, the RecSys struggles to understand why a user likes or dislikes certain items. To alleviate it, some works attempt to utilize the content-based reviews to capture the semantic knowledge to enhance recommender models. However, most of these methods focus on predicting the ratings of reviews, but do not provide a human-understandable explanation.
* Work in progress
Via
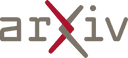
Jun 25, 2025
Abstract:This paper addresses the challenge of developing multimodal recommender systems for the movie domain, where limited metadata (e.g., title, genre) often hinders the generation of robust recommendations. We introduce a resource that combines LLM-generated plot descriptions with trailer-derived visual embeddings in a unified pipeline supporting both Retrieval-Augmented Generation (RAG) and collaborative filtering. Central to our approach is a data augmentation step that transforms sparse metadata into richer textual signals, alongside fusion strategies (e.g., PCA, CCA) that integrate visual cues. Experimental evaluations demonstrate that CCA-based fusion significantly boosts recall compared to unimodal baselines, while an LLM-driven re-ranking step further improves NDCG, particularly in scenarios with limited textual data. By releasing this framework, we invite further exploration of multi-modal recommendation techniques tailored to cold-start, novelty-focused, and domain-specific settings. All code, data, and detailed documentation are publicly available at: https://github.com/RecSys-lab/RAG-VisualRec
* 20 pages, 6 figures, 5 tables
Via
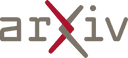
Jun 25, 2025
Abstract:LLM-powered applications are highly susceptible to the quality of user prompts, and crafting high-quality prompts can often be challenging especially for domain-specific applications. This paper presents a novel dynamic context-aware prompt recommendation system for domain-specific AI applications. Our solution combines contextual query analysis, retrieval-augmented knowledge grounding, hierarchical skill organization, and adaptive skill ranking to generate relevant and actionable prompt suggestions. The system leverages behavioral telemetry and a two-stage hierarchical reasoning process to dynamically select and rank relevant skills, and synthesizes prompts using both predefined and adaptive templates enhanced with few-shot learning. Experiments on real-world datasets demonstrate that our approach achieves high usefulness and relevance, as validated by both automated and expert evaluations.
Via
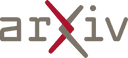
Jun 25, 2025
Abstract:Deep learning on graphs has shown remarkable success across numerous applications, including social networks, bio-physics, traffic networks, and recommendation systems. Regardless of their successes, current methods frequently depend on the assumption that training and testing data share the same distribution, a condition rarely met in real-world scenarios. While graph-transformer (GT) backbones have recently outperformed traditional message-passing neural networks (MPNNs) in multiple in-distribution (ID) benchmarks, their effectiveness under distribution shifts remains largely unexplored. In this work, we address the challenge of out-of-distribution (OOD) generalization for graph neural networks, with a special focus on the impact of backbone architecture. We systematically evaluate GT and hybrid backbones in OOD settings and compare them to MPNNs. To do so, we adapt several leading domain generalization (DG) algorithms to work with GTs and assess their performance on a benchmark designed to test a variety of distribution shifts. Our results reveal that GT and hybrid GT-MPNN backbones consistently demonstrate stronger generalization ability compared to MPNNs, even without specialized DG algorithms. Additionally, we propose a novel post-training analysis approach that compares the clustering structure of the entire ID and OOD test datasets, specifically examining domain alignment and class separation. Demonstrating its model-agnostic design, this approach not only provided meaningful insights into GT and MPNN backbones. It also shows promise for broader applicability to DG problems beyond graph learning, offering a deeper perspective on generalization abilities that goes beyond standard accuracy metrics. Together, our findings highlight the promise of graph-transformers for robust, real-world graph learning and set a new direction for future research in OOD generalization.
Via
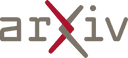
Jun 25, 2025
Abstract:For the first time, we implement the deep-neural-network-based variational Monte Carlo approach for the multiquark bound states, whose complexity surpasses that of electron or nucleon systems due to strong SU(3) color interactions. We design a novel and high-efficiency architecture, DeepQuark, to address the unique challenges in multiquark systems such as stronger correlations, extra discrete quantum numbers, and intractable confinement interaction. Our method demonstrates competitive performance with state-of-the-art approaches, including diffusion Monte Carlo and Gaussian expansion method, in the nucleon, doubly heavy tetraquark, and fully heavy tetraquark systems. Notably, it outperforms existing calculations for pentaquarks, exemplified by the triply heavy pentaquark. For the nucleon, we successfully incorporate three-body flux-tube confinement interactions without additional computational costs. In tetraquark systems, we consistently describe hadronic molecule $T_{cc}$ and compact tetraquark $T_{bb}$ with an unbiased form of wave function ansatz. In the pentaquark sector, we obtain weakly bound $\bar D^*\Xi_{cc}^*$ molecule $P_{cc\bar c}(5715)$ with $S=\frac{5}{2}$ and its bottom partner $P_{bb\bar b}(15569)$. They can be viewed as the analogs of the molecular $T_{cc}$. We recommend experimental search of $P_{cc\bar c}(5715)$ in the D-wave $J/\psi \Lambda_c$ channel. DeepQuark holds great promise for extension to larger multiquark systems, overcoming the computational barriers in conventional methods. It also serves as a powerful framework for exploring confining mechanism beyond two-body interactions in multiquark states, which may offer valuable insights into nonperturbative QCD and general many-body physics.
* 10 pages, 3 figures, 6 tables
Via
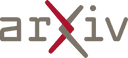
Jun 25, 2025
Abstract:Retrieval-Augmented Generation (RAG) systems are emerging as a key approach for grounding Large Language Models (LLMs) in external knowledge, addressing limitations in factual accuracy and contextual relevance. However, there is a lack of empirical studies that report on the development of RAG-based implementations grounded in real-world use cases, evaluated through general user involvement, and accompanied by systematic documentation of lessons learned. This paper presents five domain-specific RAG applications developed for real-world scenarios across governance, cybersecurity, agriculture, industrial research, and medical diagnostics. Each system incorporates multilingual OCR, semantic retrieval via vector embeddings, and domain-adapted LLMs, deployed through local servers or cloud APIs to meet distinct user needs. A web-based evaluation involving a total of 100 participants assessed the systems across six dimensions: (i) Ease of Use, (ii) Relevance, (iii) Transparency, (iv) Responsiveness, (v) Accuracy, and (vi) Likelihood of Recommendation. Based on user feedback and our development experience, we documented twelve key lessons learned, highlighting technical, operational, and ethical challenges affecting the reliability and usability of RAG systems in practice.
* Accepted as a full paper to the 51st Euromicro Conference on Software
Engineering and Advanced Applications (SEAA 2025). 9 pages, 4 figures. This
is the preprint version and not the final camera ready version
Via
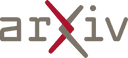
Jun 25, 2025
Abstract:[Context] Machine learning (ML)-enabled systems are present in our society, driving significant digital transformations. The dynamic nature of ML development, characterized by experimental cycles and rapid changes in data, poses challenges to traditional project management. Agile methods, with their flexibility and incremental delivery, seem well-suited to address this dynamism. However, it is unclear how to effectively apply these methods in the context of ML-enabled systems, where challenges require tailored approaches. [Goal] Our goal is to outline the state of the art in agile management for ML-enabled systems. [Method] We conducted a systematic mapping study using a hybrid search strategy that combines database searches with backward and forward snowballing iterations. [Results] Our study identified 27 papers published between 2008 and 2024. From these, we identified eight frameworks and categorized recommendations and practices into eight key themes, such as Iteration Flexibility, Innovative ML-specific Artifacts, and the Minimal Viable Model. The main challenge identified across studies was accurate effort estimation for ML-related tasks. [Conclusion] This study contributes by mapping the state of the art and identifying open gaps in the field. While relevant work exists, more robust empirical evaluation is still needed to validate these contributions.
* Accepted for publication at the 51st Euromicro Conference Series on
Software Engineering and Advanced Applications (SEAA) 2025
Via
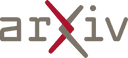
Jun 24, 2025
Abstract:A KG represents a network of entities and illustrates relationships between them. KGs are used for various applications, including semantic search and discovery, reasoning, decision-making, natural language processing, machine learning, and recommendation systems. Triple (subject-relation-object) extraction from text is the fundamental building block of KG construction and has been widely studied, for example, in early benchmarks such as ACE 2002 to more recent ones, such as WebNLG 2020, REBEL and SynthIE. While the use of LLMs is explored for KG construction, handcrafting reasonable task-specific prompts for LLMs is a labour-intensive exercise and can be brittle due to subtle changes in the LLM models employed. Recent work in NLP tasks (e.g. autonomy generation) uses automatic prompt optimization/engineering to address this challenge by generating optimal or near-optimal task-specific prompts given input-output examples. This empirical study explores the application of automatic prompt optimization for the triple extraction task using experimental benchmarking. We evaluate different settings by changing (a) the prompting strategy, (b) the LLM being used for prompt optimization and task execution, (c) the number of canonical relations in the schema (schema complexity), (d) the length and diversity of input text, (e) the metric used to drive the prompt optimization, and (f) the dataset being used for training and testing. We evaluate three different automatic prompt optimizers, namely, DSPy, APE, and TextGrad and use two different triple extraction datasets, SynthIE and REBEL. Through rigorous empirical evaluation, our main contribution highlights that automatic prompt optimization techniques can generate reasonable prompts similar to humans for triple extraction. In turn, these optimized prompts achieve improved results, particularly with increasing schema complexity and text size.
Via
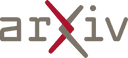
Jun 24, 2025
Abstract:We study NAFNet (Nonlinear Activation Free Network), a simple and efficient deep learning baseline for image restoration. By using CIFAR10 images corrupted with noise and blur, we conduct an ablation study of NAFNet's core components. Our baseline model implements SimpleGate activation, Simplified Channel Activation (SCA), and LayerNormalization. We compare this baseline to different variants that replace or remove components. Quantitative results (PSNR, SSIM) and examples illustrate how each modification affects restoration performance. Our findings support the NAFNet design: the SimpleGate and simplified attention mechanisms yield better results than conventional activations and attention, while LayerNorm proves to be important for stable training. We conclude with recommendations for model design, discuss potential improvements, and future work.
Via
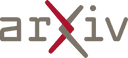
Jun 24, 2025
Abstract:We propose a new variant of online learning that we call "ambiguous online learning". In this setting, the learner is allowed to produce multiple predicted labels. Such an "ambiguous prediction" is considered correct when at least one of the labels is correct, and none of the labels are "predictably wrong". The definition of "predictably wrong" comes from a hypothesis class in which hypotheses are also multi-valued. Thus, a prediction is "predictably wrong" if it's not allowed by the (unknown) true hypothesis. In particular, this setting is natural in the context of multivalued dynamical systems, recommendation algorithms and lossless compression. It is also strongly related to so-called "apple tasting". We show that in this setting, there is a trichotomy of mistake bounds: up to logarithmic factors, any hypothesis class has an optimal mistake bound of either Theta(1), Theta(sqrt(N)) or N.
Via
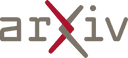