Topic:Hyperspectral Unmixing
What is Hyperspectral Unmixing? Hyperspectral unmixing is a procedure that decomposes the measured pixel spectrum of hyperspectral data into a collection of constituent spectral signatures (or endmembers) and a set of corresponding fractional abundances. Hyperspectral Unmixing techniques have been widely used for a variety of applications, such as mineral mapping and land cover change detection.
Papers and Code
Mar 25, 2025
Abstract:Hyperspectral unmixing (HU) is a critical yet challenging task in remote sensing. However, existing nonnegative matrix factorization (NMF) methods with graph learning mostly focus on first-order or second-order nearest neighbor relationships and usually require manual parameter tuning, which fails to characterize intrinsic data structures. To address the above issues, we propose a novel adaptive multi-order graph regularized NMF method (MOGNMF) with three key features. First, multi-order graph regularization is introduced into the NMF framework to exploit global and local information comprehensively. Second, these parameters associated with the multi-order graph are learned adaptively through a data-driven approach. Third, dual sparsity is embedded to obtain better robustness, i.e., $\ell_{1/2}$-norm on the abundance matrix and $\ell_{2,1}$-norm on the noise matrix. To solve the proposed model, we develop an alternating minimization algorithm whose subproblems have explicit solutions, thus ensuring effectiveness. Experiments on simulated and real hyperspectral data indicate that the proposed method delivers better unmixing results.
Via
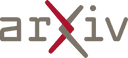
Mar 21, 2025
Abstract:Unmixing is a fundamental process in hyperspectral image processing in which the materials present in a mixed pixel are determined based on the spectra of candidate materials and the pixel spectrum. Practical and general utility requires a large spectral library with sample measurements covering the full variation in each candidate material as well as a sufficiently varied collection of potential materials. However, any spectral library with more spectra than bands will lead to an ill-posed inversion problem when using classical least-squares regression-based unmixing methods. Moreover, for numerical and dimensionality reasons, libraries with over 10 or 20 spectra behave computationally as though they are ill-posed. In current practice, unmixing is often applied to imagery using manually-selected materials or image endmembers. General unmixing of a spectrum from an unknown material with a large spectral library requires some form of sparse regression; regression where only a small number of coefficients are nonzero. This requires a trade-off between goodness-of-fit and model size. In this study we compare variations of two sparse regression techniques, focusing on the relationship between structure and chemistry of materials and the accuracy of the various models for identifying the correct mixture of materials present. Specifically, we examine LASSO regression and ElasticNet in contrast with variations of iterative feature selection, Bayesian Model Averaging (BMA), and quadratic BMA (BMA-Q) -- incorporating LASSO regression and ElasticNet as their base model. To evaluate the the effectiveness of these methods, we consider the molecular composition similarities and differences of substances selected in the models compared to the ground truth.
Via
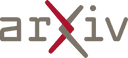
Mar 21, 2025
Abstract:Hyperspectral unmixing is the analytical process of determining the pure materials and estimating the proportions of such materials composed within an observed mixed pixel spectrum. We can unmix mixed pixel spectra using linear and nonlinear mixture models. Ordinary least squares (OLS) regression serves as the foundation for many linear mixture models employed in Hyperspectral Image analysis. Though variations of OLS are implemented, studies rarely address the underlying assumptions that affect results. This paper provides an in depth discussion on the assumptions inherently endorsed by the application of OLS regression. We also examine variations of OLS models stemming from highly effective approaches in spectral unmixing -- sparse regression, iterative feature search strategies and Mathematical programming. These variations are compared to a novel unmixing approach called HySUDeB. We evaluated each approach's performance by computing the average error and precision of each model. Additionally, we provide a taxonomy of the molecular structure of each mineral to derive further understanding into the detection of the target materials.
Via
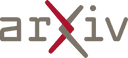
Mar 26, 2025
Abstract:With the aim of estimating the abundance map from observations only, linear unmixing approaches are not always suitable to spectral images, especially when the number of bands is too small or when the spectra of the observed data are too correlated. To address this issue in the general case, we present a novel approach which provides an adapted spatial density function based on any arbitrary linear classifier. A robust mathematical formulation for computing the Euclidean distance to polyhedral sets is presented, along with an efficient algorithm that provides the exact minimum-norm point in a polyhedron. An empirical evaluation on the widely-used Samson hyperspectral dataset demonstrates that the proposed method surpasses state-of-the-art approaches in reconstructing abundance maps. Furthermore, its application to spectral images of a Lithium-ion battery, incompatible with linear unmixing models, validates the method's generality and effectiveness.
* 14th International Conference on Pattern Recognition Applications
and Methods, Feb 2025, Porto, France. pp.192-203
Via
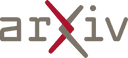
Mar 06, 2025
Abstract:Transformers have shown significant success in hyperspectral unmixing (HU). However, challenges remain. While multi-scale and long-range spatial correlations are essential in unmixing tasks, current Transformer-based unmixing networks, built on Vision Transformer (ViT) or Swin-Transformer, struggle to capture them effectively. Additionally, current Transformer-based unmixing networks rely on the linear mixing model, which lacks the flexibility to accommodate scenarios where nonlinear effects are significant. To address these limitations, we propose a multi-scale Dilated Transformer-based unmixing network for nonlinear HU (DTU-Net). The encoder employs two branches. The first one performs multi-scale spatial feature extraction using Multi-Scale Dilated Attention (MSDA) in the Dilated Transformer, which varies dilation rates across attention heads to capture long-range and multi-scale spatial correlations. The second one performs spectral feature extraction utilizing 3D-CNNs with channel attention. The outputs from both branches are then fused to integrate multi-scale spatial and spectral information, which is subsequently transformed to estimate the abundances. The decoder is designed to accommodate both linear and nonlinear mixing scenarios. Its interpretability is enhanced by explicitly modeling the relationships between endmembers, abundances, and nonlinear coefficients in accordance with the polynomial post-nonlinear mixing model (PPNMM). Experiments on synthetic and real datasets validate the effectiveness of the proposed DTU-Net compared to PPNMM-derived methods and several advanced unmixing networks.
Via
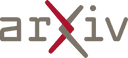
Mar 11, 2025
Abstract:Hyperspectral image (HSI) unmixing is a challenging research problem that tries to identify the constituent components, known as endmembers, and their corresponding proportions, known as abundances, in the scene by analysing images captured by hyperspectral cameras. Recently, many deep learning based unmixing approaches have been proposed with the surge of machine learning techniques, especially convolutional neural networks (CNN). However, these methods face two notable challenges: 1. They frequently yield results lacking physical significance, such as signatures corresponding to unknown or non-existent materials. 2. CNNs, as general-purpose network structures, are not explicitly tailored for unmixing tasks. In response to these concerns, our work draws inspiration from double deep image prior (DIP) techniques and algorithm unrolling, presenting a novel network structure that effectively addresses both issues. Specifically, we first propose a MatrixConv Unmixing (MCU) approach for endmember and abundance estimation, respectively, which can be solved via certain iterative solvers. We then unroll these solvers to build two sub-networks, endmember estimation DIP (UEDIP) and abundance estimation DIP (UADIP), to generate the estimation of endmember and abundance, respectively. The overall network is constructed by assembling these two sub-networks. In order to generate meaningful unmixing results, we also propose a composite loss function. To further improve the unmixing quality, we also add explicitly a regularizer for endmember and abundance estimation, respectively. The proposed methods are tested for effectiveness on both synthetic and real datasets.
Via
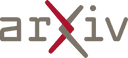
Feb 24, 2025
Abstract:Spectral unmixing is an important task in the research field of hyperspectral image processing. It can be thought of as a regression problem, where the observed variable (i.e., an image pixel) is to be found as a function of the response variables (i.e., the pure materials in a scene, called endmembers). The Linear Mixing Model (LMM) has received a great deal of attention, due to its simplicity and ease of use in, e.g., optimization problems. Its biggest flaw is that it assumes that any pure material can be characterized by one unique spectrum throughout the entire scene. In many cases this is incorrect: the endmembers face a significant amount of spectral variability caused by, e.g., illumination conditions, atmospheric effects, or intrinsic variability. Researchers have suggested several generalizations of the LMM to mitigate this effect. However, most models lead to ill-posed and highly non-convex optimization problems, which are hard to solve and have hyperparameters that are difficult to tune. In this paper, we propose a two-step LMM that bridges the gap between model complexity and computational tractability. We show that this model leads to only a mildly non-convex optimization problem, which we solve with an interior-point solver. This method requires virtually no hyperparameter tuning, and can therefore be used easily and quickly in a wide range of unmixing tasks. We show that the model is competitive and in some cases superior to existing and well-established unmixing methods and algorithms. We do this through several experiments on synthetic data, real-life satellite data, and hybrid synthetic-real data.
* 13 pages, 10 figures, 5 tables, submitted journal paper
Via
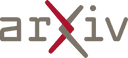
Feb 09, 2025
Abstract:Hyperspectral imaging (HSI) captures spatial and spectral data, enabling analysis of features invisible to conventional systems. The technology is vital in fields such as weather monitoring, food quality control, counterfeit detection, healthcare diagnostics, and extending into defense, agriculture, and industrial automation at the same time. HSI has advanced with improvements in spectral resolution, miniaturization, and computational methods. This study provides an overview of the HSI, its applications, challenges in data fusion and the role of deep learning models in processing HSI data. We discuss how integration of multimodal HSI with AI, particularly with deep learning, improves classification accuracy and operational efficiency. Deep learning enhances HSI analysis in areas like feature extraction, change detection, denoising unmixing, dimensionality reduction, landcover mapping, data augmentation, spectral construction and super resolution. An emerging focus is the fusion of hyperspectral cameras with large language models (LLMs), referred as highbrain LLMs, enabling the development of advanced applications such as low visibility crash detection and face antispoofing. We also highlight key players in HSI industry, its compound annual growth rate and the growing industrial significance. The purpose is to offer insight to both technical and non-technical audience, covering HSI's images, trends, and future directions, while providing valuable information on HSI datasets and software libraries.
* 39 Pages, 22 figures, 20 tables
Via
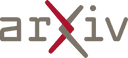
Sep 29, 2024
Abstract:The hyperspectral unmixing method is an algorithm that extracts material (usually called endmember) data from hyperspectral data cube pixels along with their abundances. Due to a lower spatial resolution of hyperspectral sensors data in each of the pixels may contain mixed information from multiple endmembers. In this paper we create a hyperspectral unmixing dataset, created from blueberry field data gathered by a hyperspectral camera mounted on a UAV. We also propose a hyperspectral unmixing algorithm based on U-Net network architecture to achieve more accurate unmixing results on existing and newly created hyperspectral unmixing datasets.
Via
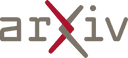
Dec 05, 2024
Abstract:Deep learning (DL) has been widely applied into hyperspectral image (HSI) classification owing to its promising feature learning and representation capabilities. However, limited by the spatial resolution of sensors, existing DL-based classification approaches mainly focus on pixel-level spectral and spatial information extraction through complex network architecture design, while ignoring the existence of mixed pixels in actual scenarios. To tackle this difficulty, we propose a novel dual-branch subpixel-guided network for HSI classification, called DSNet, which automatically integrates subpixel information and convolutional class features by introducing a deep autoencoder unmixing architecture to enhance classification performance. DSNet is capable of fully considering physically nonlinear properties within subpixels and adaptively generating diagnostic abundances in an unsupervised manner to achieve more reliable decision boundaries for class label distributions. The subpixel fusion module is designed to ensure high-quality information fusion across pixel and subpixel features, further promoting stable joint classification. Experimental results on three benchmark datasets demonstrate the effectiveness and superiority of DSNet compared with state-of-the-art DL-based HSI classification approaches. The codes will be available at https://github.com/hanzhu97702/DSNet, contributing to the remote sensing community.
Via
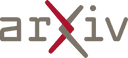