May 02, 2025
Abstract:In a world where technology is increasingly embedded in our everyday experiences, systems that sense and respond to human emotions are elevating digital interaction. At the intersection of artificial intelligence and human-computer interaction, affective computing is emerging with innovative solutions where machines are humanized by enabling them to process and respond to user emotions. This survey paper explores recent research contributions in affective computing applications in the area of emotion recognition, sentiment analysis and personality assignment developed using approaches like large language models (LLMs), multimodal techniques, and personalized AI systems. We analyze the key contributions and innovative methodologies applied by the selected research papers by categorizing them into four domains: AI chatbot applications, multimodal input systems, mental health and therapy applications, and affective computing for safety applications. We then highlight the technological strengths as well as the research gaps and challenges related to these studies. Furthermore, the paper examines the datasets used in each study, highlighting how modality, scale, and diversity impact the development and performance of affective models. Finally, the survey outlines ethical considerations and proposes future directions to develop applications that are more safe, empathetic and practical.
* 20 pages, 7 tables, 96 references. Survey paper on affective
computing applications using large language models, multimodal AI, and
therapeutic chatbots
Via
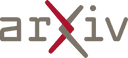