What is Sentiment Analysis? Sentiment analysis is the process of determining the sentiment of a piece of text, such as a tweet or a review.
Papers and Code
Apr 24, 2025
Abstract:Multimodal language analysis is a rapidly evolving field that leverages multiple modalities to enhance the understanding of high-level semantics underlying human conversational utterances. Despite its significance, little research has investigated the capability of multimodal large language models (MLLMs) to comprehend cognitive-level semantics. In this paper, we introduce MMLA, a comprehensive benchmark specifically designed to address this gap. MMLA comprises over 61K multimodal utterances drawn from both staged and real-world scenarios, covering six core dimensions of multimodal semantics: intent, emotion, dialogue act, sentiment, speaking style, and communication behavior. We evaluate eight mainstream branches of LLMs and MLLMs using three methods: zero-shot inference, supervised fine-tuning, and instruction tuning. Extensive experiments reveal that even fine-tuned models achieve only about 60%~70% accuracy, underscoring the limitations of current MLLMs in understanding complex human language. We believe that MMLA will serve as a solid foundation for exploring the potential of large language models in multimodal language analysis and provide valuable resources to advance this field. The datasets and code are open-sourced at https://github.com/thuiar/MMLA.
* 23 pages, 5 figures
Via
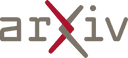
Apr 26, 2025
Abstract:This paper investigates the structural dynamics of stock market volatility through the Financial Chaos Index, a tensor- and eigenvalue-based measure designed to capture realized volatility via mutual fluctuations among asset prices. Motivated by empirical evidence of regime-dependent volatility behavior and perceptual time dilation during financial crises, we develop a regime-switching framework based on the Modified Lognormal Power-Law distribution. Analysis of the FCIX from January 1990 to December 2023 identifies three distinct market regimes, low-chaos, intermediate-chaos, and high-chaos, each characterized by differing levels of systemic stress, statistical dispersion and persistence characteristics. Building upon the segmented regime structure, we further examine the informational forces that shape forward-looking market expectations. Using sentiment-based predictors derived from the Equity Market Volatility tracker, we employ an elastic net regression model to forecast implied volatility, as proxied by the VIX index. Our findings indicate that shifts in macroeconomic, financial, policy, and geopolitical uncertainty exhibit strong predictive power for volatility dynamics across regimes. Together, these results offer a unified empirical perspective on how systemic uncertainty governs both the realized evolution of financial markets and the anticipatory behavior embedded in implied volatility measures.
Via
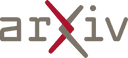
Mar 27, 2025
Abstract:Large Language models (LLMs) have been prominent for language translation, including low-resource languages. There has been limited study about the assessment of the quality of translations generated by LLMs, including Gemini, GPT and Google Translate. In this study, we address this limitation by using semantic and sentiment analysis of selected LLMs for Indian languages, including Sanskrit, Telugu and Hindi. We select prominent texts that have been well translated by experts and use LLMs to generate their translations to English, and then we provide a comparison with selected expert (human) translations. Our findings suggest that while LLMs have made significant progress in translation accuracy, challenges remain in preserving sentiment and semantic integrity, especially in figurative and philosophical contexts. The sentiment analysis revealed that GPT-4o and GPT-3.5 are better at preserving the sentiments for the Bhagavad Gita (Sanskrit-English) translations when compared to Google Translate. We observed a similar trend for the case of Tamas (Hindi-English) and Maha P (Telugu-English) translations. GPT-4o performs similarly to GPT-3.5 in the translation in terms of sentiments for the three languages. We found that LLMs are generally better at translation for capturing sentiments when compared to Google Translate.
Via
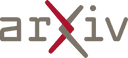
Apr 01, 2025
Abstract:There are not one but two dimensions of bias that can be revealed through the study of large AI models: not only bias in training data or the products of an AI, but also bias in society, such as disparity in employment or health outcomes between different demographic groups. Often training data and AI output is biased for or against certain demographics (i.e. older white people are overrepresented in image datasets), but sometimes large AI models accurately illustrate biases in the real world (i.e. young black men being disproportionately viewed as threatening). These social disparities often appear in image generation AI outputs in the form of 'marked' features, where some feature of an individual or setting is a social marker of disparity, and prompts both humans and AI systems to treat subjects that are marked in this way as exceptional and requiring special treatment. Generative AI has proven to be very sensitive to such marked features, to the extent of over-emphasising them and thus often exacerbating social biases. I briefly discuss how we can use complex prompts to image generation AI to investigate either dimension of bias, emphasising how we can probe the large language models underlying image generation AI through, for example, automated sentiment analysis of the text prompts used to generate images.
* Presented at the 74th Annual ICA 2024 Conference, in the stream
"Image-as-Data Methods in the Age of Generative Artificial Intelligence", 22
June 2024
Via
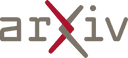
Apr 02, 2025
Abstract:Automatic text classification (ATC) has experienced remarkable advancements in the past decade, best exemplified by recent small and large language models (SLMs and LLMs), leveraged by Transformer architectures. Despite recent effectiveness improvements, a comprehensive cost-benefit analysis investigating whether the effectiveness gains of these recent approaches compensate their much higher costs when compared to more traditional text classification approaches such as SVMs and Logistic Regression is still missing in the literature. In this context, this work's main contributions are twofold: (i) we provide a scientifically sound comparative analysis of the cost-benefit of twelve traditional and recent ATC solutions including five open LLMs, and (ii) a large benchmark comprising {22 datasets}, including sentiment analysis and topic classification, with their (train-validation-test) partitions based on folded cross-validation procedures, along with documentation, and code. The release of code, data, and documentation enables the community to replicate experiments and advance the field in a more scientifically sound manner. Our comparative experimental results indicate that LLMs outperform traditional approaches (up to 26%-7.1% on average) and SLMs (up to 4.9%-1.9% on average) in terms of effectiveness. However, LLMs incur significantly higher computational costs due to fine-tuning, being, on average 590x and 8.5x slower than traditional methods and SLMs, respectively. Results suggests the following recommendations: (1) LLMs for applications that require the best possible effectiveness and can afford the costs; (2) traditional methods such as Logistic Regression and SVM for resource-limited applications or those that cannot afford the cost of tuning large LLMs; and (3) SLMs like Roberta for near-optimal effectiveness-efficiency trade-off.
* 7 pages, 2 figures, 3 tables
Via
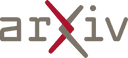
Mar 28, 2025
Abstract:Central bank communication plays a critical role in shaping economic expectations and monetary policy effectiveness. This study applies supervised machine learning techniques to classify the sentiment of press releases from the Bank of Thailand, addressing gaps in research that primarily focus on lexicon-based approaches. My findings show that supervised learning can be an effective method, even with smaller datasets, and serves as a starting point for further automation. However, achieving higher accuracy and better generalization requires a substantial amount of labeled data, which is time-consuming and demands expertise. Using models such as Na\"ive Bayes, Random Forest and SVM, this study demonstrates the applicability of machine learning for central bank sentiment analysis, with English-language communications from the Thai Central Bank as a case study.
Via
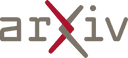
Apr 01, 2025
Abstract:Negation plays an important role in various natural language processing tasks such as Natural Language Inference and Sentiment Analysis tasks. Numerous prior studies have found that contextual text embedding models such as BERT, ELMO, RoBERTa or XLNet face challenges in accurately understanding negation. Recent advancements in universal text embeddings have demonstrated superior performance over contextual text embeddings in various tasks. However, due to the bias in popular evaluation benchmarks, the negation awareness capacity of these models remains unclear. To bridge the gap in existing literature, an in-depth analysis is initiated in this work to study the negation awareness of cutting-edge universal text embedding models. Our findings reveal a significant lack of negation awareness in these models, often interpreting negated text pairs as semantically similar. To efficiently deal with the conflict that different tasks need different trade-offs between topic and negation information among other semantic information, a data-efficient and computational-efficient embedding re-weighting method is proposed without modifying the parameters of text embedding models. The proposed solution is able to improve text embedding models' negation awareness significantly on both simple negation understanding task and complex negation understanding task. Furthermore, the proposed solution can also significantly improve the negation awareness of Large Language Model based task-specific high dimensional universal text embeddings.
Via
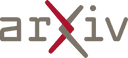
Mar 27, 2025
Abstract:This research presents a hybrid emotion recognition system integrating advanced Deep Learning, Natural Language Processing (NLP), and Large Language Models (LLMs) to analyze audio and textual data for enhancing customer interactions in contact centers. By combining acoustic features with textual sentiment analysis, the system achieves nuanced emotion detection, addressing the limitations of traditional approaches in understanding complex emotional states. Leveraging LSTM and CNN models for audio analysis and DistilBERT for textual evaluation, the methodology accommodates linguistic and cultural variations while ensuring real-time processing. Rigorous testing on diverse datasets demonstrates the system's robustness and accuracy, highlighting its potential to transform customer service by enabling personalized, empathetic interactions and improving operational efficiency. This research establishes a foundation for more intelligent and human-centric digital communication, redefining customer service standards.
* 5 pages, 1 figure, 2 tables
Via
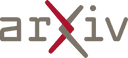
Mar 15, 2025
Abstract:This research presents an advanced sentiment analysis framework studied on Iranian restaurant reviews, combining fuzzy logic with conventional sentiment analysis techniques to assess both sentiment polarity and intensity. A dataset of 1266 reviews, alongside corresponding star ratings, was compiled and preprocessed for analysis. Initial sentiment analysis was conducted using the Sentiment Intensity Analyzer (VADER), a rule-based tool that assigns sentiment scores across positive, negative, and neutral categories. However, a noticeable bias toward neutrality often led to an inaccurate representation of sentiment intensity. To mitigate this issue, based on a fuzzy perspective, two refinement techniques were introduced, applying square-root and fourth-root transformations to amplify positive and negative sentiment scores while maintaining neutrality. This led to three distinct methodologies: Approach 1, utilizing unaltered VADER scores; Approach 2, modifying sentiment values using the square root; and Approach 3, applying the fourth root for further refinement. A Fuzzy Inference System incorporating comprehensive fuzzy rules was then developed to process these refined scores and generate a single, continuous sentiment value for each review based on each approach. Comparative analysis, including human supervision and alignment with customer star ratings, revealed that the refined approaches significantly improved sentiment analysis by reducing neutrality bias and better capturing sentiment intensity. Despite these advancements, minor over-amplification and persistent neutrality in domain-specific cases were identified, leading us to propose several future studies to tackle these occasional barriers. The study's methodology and outcomes offer valuable insights for businesses seeking a more precise understanding of consumer sentiment, enhancing sentiment analysis across various industries.
Via
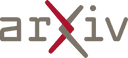
Mar 15, 2025
Abstract:Interpretability remains a key difficulty in sentiment analysis with Large Language Models (LLMs), particularly in high-stakes applications where it is crucial to comprehend the rationale behind forecasts. This research addressed this by introducing a technique that applies SHAP (Shapley Additive Explanations) by breaking down LLMs into components such as embedding layer,encoder,decoder and attention layer to provide a layer-by-layer knowledge of sentiment prediction. The approach offers a clearer overview of how model interpret and categorise sentiment by breaking down LLMs into these parts. The method is evaluated using the Stanford Sentiment Treebank (SST-2) dataset, which shows how different sentences affect different layers. The effectiveness of layer-wise SHAP analysis in clarifying sentiment-specific token attributions is demonstrated by experimental evaluations, which provide a notable enhancement over current whole-model explainability techniques. These results highlight how the suggested approach could improve the reliability and transparency of LLM-based sentiment analysis in crucial applications.
Via
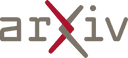