Feb 17, 2025
Abstract:Recently, self-supervised learning methods based on masked latent prediction have proven to encode input data into powerful representations. However, during training, the learned latent space can be further transformed to extract higher-level information that could be more suited for downstream classification tasks. Therefore, we propose a new method: MAsked latenT Prediction And Classification (MATPAC), which is trained with two pretext tasks solved jointly. As in previous work, the first pretext task is a masked latent prediction task, ensuring a robust input representation in the latent space. The second one is unsupervised classification, which utilises the latent representations of the first pretext task to match probability distributions between a teacher and a student. We validate the MATPAC method by comparing it to other state-of-the-art proposals and conducting ablations studies. MATPAC reaches state-of-the-art self-supervised learning results on reference audio classification datasets such as OpenMIC, GTZAN, ESC-50 and US8K and outperforms comparable supervised methods results for musical auto-tagging on Magna-tag-a-tune.
* Copyright 2025 IEEE. Personal use of this material is permitted.
Permission from IEEE must be obtained for all other uses, in any current or
future media, including reprinting/republishing this material for advertising
or promotional purposes, creating new collective works, for resale or
redistribution to servers or lists, or reuse of any copyrighted component of
this work in other works
Via
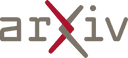