May 05, 2025
Abstract:Identifying and counting blood components such as red blood cells, various types of white blood cells, and platelets is a critical task for healthcare practitioners. Deep learning approaches, particularly convolutional neural networks (CNNs) using supervised learning strategies, have shown considerable success for such tasks. However, CNN based architectures such as U-Net, often struggles to accurately identify platelets due to their sizes and high variability of features. To address these challenges, researchers have commonly employed strategies such as class weighted loss functions, which have demonstrated some success. However, this does not address the more significant challenge of platelet variability in size and tendency to form aggregates and associations with other blood components. In this study, we explored an alternative approach by investigating the role of convolutional kernels in mitigating these issues. We also assigned separate classes to singular platelets and platelet aggregates and performed semantic segmentation using various U-Net architectures for identifying platelets. We then evaluated and compared two common methods (pixel area method and connected component analysis) for counting platelets and proposed an alternative approach specialized for single platelets and platelet aggregates. Our experiments provided results that showed significant improvements in the identification of platelets, highlighting the importance of optimizing convolutional operations and class designations. We show that the common practice of pixel area-based counting often over estimate platelet counts, whereas the proposed method presented in this work offers significant improvements. We discuss in detail about these methods from segmentation masks.
* International Joint Conference on Neural Networks (IJCNN 2025)
Via
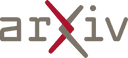