What is cancer detection? Cancer detection using Artificial Intelligence (AI) involves leveraging advanced machine learning algorithms and techniques to identify and diagnose cancer from various medical data sources. The goal is to enhance early detection, improve diagnostic accuracy, and potentially reduce the need for invasive procedures.
Papers and Code
Mar 28, 2025
Abstract:Mammography stands as the main screening method for detecting breast cancer early, enhancing treatment success rates. The segmentation of landmark structures in mammography images can aid the medical assessment in the evaluation of cancer risk and the image acquisition adequacy. We introduce a series of data-centric strategies aimed at enriching the training data for deep learning-based segmentation of landmark structures. Our approach involves augmenting the training samples through annotation-guided image intensity manipulation and style transfer to achieve better generalization than standard training procedures. These augmentations are applied in a balanced manner to ensure the model learns to process a diverse range of images generated by different vendor equipments while retaining its efficacy on the original data. We present extensive numerical and visual results that demonstrate the superior generalization capabilities of our methods when compared to the standard training. For this evaluation, we consider a large dataset that includes mammography images generated by different vendor equipments. Further, we present complementary results that show both the strengths and limitations of our methods across various scenarios. The accuracy and robustness demonstrated in the experiments suggest that our method is well-suited for integration into clinical practice.
Via
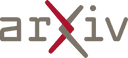
Apr 07, 2025
Abstract:Mohs micrographic surgery (MMS) is the gold standard technique for removing high risk nonmelanoma skin cancer however, intraoperative histopathological examination demands significant time, effort, and professionality. The objective of this study is to develop a deep learning model to detect basal cell carcinoma (BCC) and artifacts on Mohs slides. A total of 731 Mohs slides from 51 patients with BCCs were used in this study, with 91 containing tumor and 640 without tumor which was defined as non-tumor. The dataset was employed to train U-Net based models that segment tumor and non-tumor regions on the slides. The segmented patches were classified as tumor, or non-tumor to produce predictions for whole slide images (WSIs). For the segmentation phase, the deep learning model success was measured using a Dice score with 0.70 and 0.67 value, area under the curve (AUC) score with 0.98 and 0.96 for tumor and non-tumor, respectively. For the tumor classification, an AUC of 0.98 for patch-based detection, and AUC of 0.91 for slide-based detection was obtained on the test dataset. We present an AI system that can detect tumors and non-tumors in Mohs slides with high success. Deep learning can aid Mohs surgeons and dermatopathologists in making more accurate decisions.
* 14 pages, 2 figures
Via
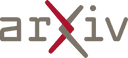
Mar 19, 2025
Abstract:Recent advancements in detecting tumors using deep learning on breast ultrasound images (BUSI) have demonstrated significant success. Deep CNNs and vision-transformers (ViTs) have demonstrated individually promising initial performance. However, challenges related to model complexity and contrast, texture, and tumor morphology variations introduce uncertainties that hinder the effectiveness of current methods. This study introduces a novel hybrid framework, CB-Res-RBCMT, combining customized residual CNNs and new ViT components for detailed BUSI cancer analysis. The proposed RBCMT uses stem convolution blocks with CNN Meet Transformer (CMT) blocks, followed by new Regional and boundary (RB) feature extraction operations for capturing contrast and morphological variations. Moreover, the CMT block incorporates global contextual interactions through multi-head attention, enhancing computational efficiency with a lightweight design. Additionally, the customized inverse residual and stem CNNs within the CMT effectively extract local texture information and handle vanishing gradients. Finally, the new channel-boosted (CB) strategy enriches the feature diversity of the limited dataset by combining the original RBCMT channels with transfer learning-based residual CNN-generated maps. These diverse channels are processed through a spatial attention block for optimal pixel selection, reducing redundancy and improving the discrimination of minor contrast and texture variations. The proposed CB-Res-RBCMT achieves an F1-score of 95.57%, accuracy of 95.63%, sensitivity of 96.42%, and precision of 94.79% on the standard harmonized stringent BUSI dataset, outperforming existing ViT and CNN methods. These results demonstrate the versatility of our integrated CNN-Transformer framework in capturing diverse features and delivering superior performance in BUSI cancer diagnosis.
* 12 pages, 10 Figures, 2 Tables. arXiv admin note: substantial text
overlap with arXiv:2405.12986
Via
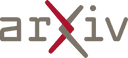
Apr 09, 2025
Abstract:Lung cancer remains one of the leading causes of cancer-related mortality worldwide, with early and accurate diagnosis playing a pivotal role in improving patient outcomes. Automated detection of pulmonary nodules in computed tomography (CT) scans is a challenging task due to variability in nodule size, shape, texture, and location. Traditional Convolutional Neural Networks (CNNs) have shown considerable promise in medical image analysis; however, their limited ability to capture fine-grained spatial-spectral variations restricts their performance in complex diagnostic scenarios. In this study, we propose a novel hybrid deep learning architecture that incorporates Chebyshev polynomial expansions into CNN layers to enhance expressive power and improve the representation of underlying anatomical structures. The proposed Chebyshev-CNN leverages the orthogonality and recursive properties of Chebyshev polynomials to extract high-frequency features and approximate complex nonlinear functions with greater fidelity. The model is trained and evaluated on benchmark lung cancer imaging datasets, including LUNA16 and LIDC-IDRI, achieving superior performance in classifying pulmonary nodules as benign or malignant. Quantitative results demonstrate significant improvements in accuracy, sensitivity, and specificity compared to traditional CNN-based approaches. This integration of polynomial-based spectral approximation within deep learning provides a robust framework for enhancing automated medical diagnostics and holds potential for broader applications in clinical decision support systems.
Via
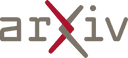
Apr 07, 2025
Abstract:Robust localization of lymph nodes (LNs) in multiparametric MRI (mpMRI) is critical for the assessment of lymphadenopathy. Radiologists routinely measure the size of LN to distinguish benign from malignant nodes, which would require subsequent cancer staging. Sizing is a cumbersome task compounded by the diverse appearances of LNs in mpMRI, which renders their measurement difficult. Furthermore, smaller and potentially metastatic LNs could be missed during a busy clinical day. To alleviate these imaging and workflow problems, we propose a pipeline to universally detect both benign and metastatic nodes in the body for their ensuing measurement. The recently proposed VFNet neural network was employed to identify LN in T2 fat suppressed and diffusion weighted imaging (DWI) sequences acquired by various scanners with a variety of exam protocols. We also use a selective augmentation technique known as Intra-Label LISA (ILL) to diversify the input data samples the model sees during training, such that it improves its robustness during the evaluation phase. We achieved a sensitivity of $\sim$83\% with ILL vs. $\sim$80\% without ILL at 4 FP/vol. Compared with current LN detection approaches evaluated on mpMRI, we show a sensitivity improvement of $\sim$9\% at 4 FP/vol.
* Published at SPIE Medical Imaging 2023
Via
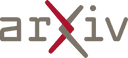
Mar 17, 2025
Abstract:Breast cancer remains one of the leading causes of cancer-related deaths worldwide. Early detection is crucial for improving patient outcomes, yet the diagnostic process is often complex and prone to inconsistencies among pathologists. Computer-aided diagnostic approaches have significantly enhanced breast cancer detection, particularly in binary classification (benign vs. malignant). However, these methods face challenges in multiclass classification, leading to frequent mispredictions. In this work, we propose a novel adaptive learning approach for multiclass breast cancer classification using H&E-stained histopathology images. First, we introduce a misprediction risk analysis framework that quantifies and ranks the likelihood of an image being mislabeled by a classifier. This framework leverages an interpretable risk model that requires only a small number of labeled samples for training. Next, we present an adaptive learning strategy that fine-tunes classifiers based on the specific characteristics of a given dataset. This approach minimizes misprediction risk, allowing the classifier to adapt effectively to the target workload. We evaluate our proposed solutions on real benchmark datasets, demonstrating that our risk analysis framework more accurately identifies mispredictions compared to existing methods. Furthermore, our adaptive learning approach significantly improves the performance of state-of-the-art deep neural network classifiers.
Via
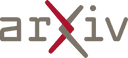
Mar 28, 2025
Abstract:Breast cancer, the most common malignancy among women, requires precise detection and classification for effective treatment. Immunohistochemistry (IHC) biomarkers like HER2, ER, and PR are critical for identifying breast cancer subtypes. However, traditional IHC classification relies on pathologists' expertise, making it labor-intensive and subject to significant inter-observer variability. To address these challenges, this study introduces the India Pathology Breast Cancer Dataset (IPD-Breast), comprising of 1,272 IHC slides (HER2, ER, and PR) aimed at automating receptor status classification. The primary focus is on developing predictive models for HER2 3-way classification (0, Low, High) to enhance prognosis. Evaluation of multiple deep learning models revealed that an end-to-end ConvNeXt network utilizing low-resolution IHC images achieved an AUC, F1, and accuracy of 91.79%, 83.52%, and 83.56%, respectively, for 3-way classification, outperforming patch-based methods by over 5.35% in F1 score. This study highlights the potential of simple yet effective deep learning techniques to significantly improve accuracy and reproducibility in breast cancer classification, supporting their integration into clinical workflows for better patient outcomes.
Via
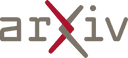
Mar 13, 2025
Abstract:The integration of Internet of Things (IoT) technology in pulmonary nodule detection significantly enhances the intelligence and real-time capabilities of the detection system. Currently, lung nodule detection primarily focuses on the identification of solid nodules, but different types of lung nodules correspond to various forms of lung cancer. Multi-type detection contributes to improving the overall lung cancer detection rate and enhancing the cure rate. To achieve high sensitivity in nodule detection, targeted improvements were made to the YOLOv8 model. Firstly, the C2f\_RepViTCAMF module was introduced to augment the C2f module in the backbone, thereby enhancing detection accuracy for small lung nodules and achieving a lightweight model design. Secondly, the MSCAF module was incorporated to reconstruct the feature fusion section of the model, improving detection accuracy for lung nodules of varying scales. Furthermore, the KAN network was integrated into the model. By leveraging the KAN network's powerful nonlinear feature learning capability, detection accuracy for small lung nodules was further improved, and the model's generalization ability was enhanced. Tests conducted on the LUNA16 dataset demonstrate that the improved model outperforms the original model as well as other mainstream models such as YOLOv9 and RT-DETR across various evaluation metrics.
Via
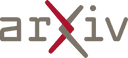
Mar 29, 2025
Abstract:Tissue detection is a crucial first step in most digital pathology applications. Details of the segmentation algorithm are rarely reported, and there is a lack of studies investigating the downstream effects of a poor segmentation algorithm. Disregarding tissue detection quality could create a bottleneck for downstream performance and jeopardize patient safety if diagnostically relevant parts of the specimen are excluded from analysis in clinical applications. This study aims to determine whether performance of downstream tasks is sensitive to the tissue detection method, and to compare performance of classical and AI-based tissue detection. To this end, we trained an AI model for Gleason grading of prostate cancer in whole slide images (WSIs) using two different tissue detection algorithms: thresholding (classical) and UNet++ (AI). A total of 33,823 WSIs scanned on five digital pathology scanners were used to train the tissue detection AI model. The downstream Gleason grading algorithm was trained and tested using 70,524 WSIs from 13 clinical sites scanned on 13 different scanners. There was a decrease from 116 (0.43%) to 22 (0.08%) fully undetected tissue samples when switching from thresholding-based tissue detection to AI-based, suggesting an AI model may be more reliable than a classical model for avoiding total failures on slides with unusual appearance. On the slides where tissue could be detected by both algorithms, no significant difference in overall Gleason grading performance was observed. However, tissue detection dependent clinically significant variations in AI grading were observed in 3.5% of malignant slides, highlighting the importance of robust tissue detection for optimal clinical performance of diagnostic AI.
* 25 pages, 2 tables, 3 figures, 1 supplementary figure
Via
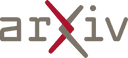
Mar 14, 2025
Abstract:Real-time computer-aided diagnosis using artificial intelligence (AI), with images, can help oncologists diagnose cancer with high accuracy and in an early phase. We reviewed real-time AI-based analyzed images for decision-making in different cancer types. This paper provides insights into the present and future potential of real-time imaging and image fusion. It explores various real-time techniques, encompassing technical solutions, AI-based imaging, and image fusion diagnosis across multiple anatomical areas, and electromagnetic needle tracking. To provide a thorough overview, this paper discusses ultrasound image fusion, real-time in vivo cancer diagnosis with different spectroscopic techniques, different real-time optical imaging-based cancer diagnosis techniques, elastography-based cancer diagnosis, cervical cancer detection using neuromorphic architectures, different fluorescence image-based cancer diagnosis techniques, and hyperspectral imaging-based cancer diagnosis. We close by offering a more futuristic overview to solve existing problems in real-time image-based cancer diagnosis.
* This paper is under review
Via
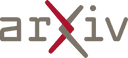