Topic:Skin Cancer Classification
What is Skin Cancer Classification? Skin cancer classification is the process of identifying and categorizing different types of skin cancer using deep learning techniques.
Papers and Code
May 07, 2025
Abstract:Recent advancements in digital pathology have enabled comprehensive analysis of Whole-Slide Images (WSI) from tissue samples, leveraging high-resolution microscopy and computational capabilities. Despite this progress, there is a lack of labeled datasets and open source pipelines specifically tailored for analysis of skin tissue. Here we propose Histo-Miner, a deep learning-based pipeline for analysis of skin WSIs and generate two datasets with labeled nuclei and tumor regions. We develop our pipeline for the analysis of patient samples of cutaneous squamous cell carcinoma (cSCC), a frequent non-melanoma skin cancer. Utilizing the two datasets, comprising 47,392 annotated cell nuclei and 144 tumor-segmented WSIs respectively, both from cSCC patients, Histo-Miner employs convolutional neural networks and vision transformers for nucleus segmentation and classification as well as tumor region segmentation. Performance of trained models positively compares to state of the art with multi-class Panoptic Quality (mPQ) of 0.569 for nucleus segmentation, macro-averaged F1 of 0.832 for nucleus classification and mean Intersection over Union (mIoU) of 0.884 for tumor region segmentation. From these predictions we generate a compact feature vector summarizing tissue morphology and cellular interactions, which can be used for various downstream tasks. Here, we use Histo-Miner to predict cSCC patient response to immunotherapy based on pre-treatment WSIs from 45 patients. Histo-Miner identifies percentages of lymphocytes, the granulocyte to lymphocyte ratio in tumor vicinity and the distances between granulocytes and plasma cells in tumors as predictive features for therapy response. This highlights the applicability of Histo-Miner to clinically relevant scenarios, providing direct interpretation of the classification and insights into the underlying biology.
* 31 pages including supplement, 5 core figures, 5 supplement figures
Via
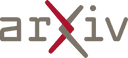
Apr 25, 2025
Abstract:Photon Absorption Remote Sensing (PARS) enables label-free imaging of subcellular morphology by observing biomolecule specific absorption interactions. Coupled with deep-learning, PARS produces label-free virtual Hematoxylin and Eosin (H&E) stained images in unprocessed tissues. This study evaluates the diagnostic performance of these PARS-derived virtual H&E images in benign and malignant excisional skin biopsies, including Squamous (SCC), Basal (BCC) Cell Carcinoma, and normal skin. Sixteen unstained formalin-fixed paraffin-embedded skin excisions were PARS imaged, virtually H&E stained, then chemically stained and imaged at 40x. Seven fellowship trained dermatopathologists assessed all 32 images in a masked randomized fashion. Concordance analysis indicates 95.5% agreement between primary diagnoses rendered on PARS versus H&E images (Cohen's k=0.93). Inter-rater reliability was near-perfect for both image types (Fleiss' k=0.89 for PARS, k=0.80 for H&E). For subtype classification, agreement was near-perfect 91% (k=0.73) for SCC and was perfect for BCC. When assessing malignancy confinement (e.g., cancer margins), agreement was 92% between PARS and H&E (k=0.718). During assessment dermatopathologists could not reliably distinguish image origin (PARS vs. H&E), and diagnostic confidence was equivalent between the modalities. Inter-rater reliability for PARS virtual H&E was consistent with reported benchmarks for histologic evaluation. These results indicate that PARS virtual histology may be diagnostically equivalent to traditional H&E staining in dermatopathology diagnostics, while enabling assessment directly from unlabeled, or unprocessed slides. In turn, the label-free PARS virtual H&E imaging workflow may preserve tissue for downstream analysis while producing data well-suited for AI integration potentially accelerating and enhancing the accuracy of skin cancer diagnostics.
* 19 pages, 3 figures, 6 tables
Via
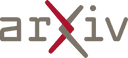
Mar 31, 2025
Abstract:Melanoma is the most lethal form of skin cancer, with an increasing incidence rate worldwide. Analyzing histological images of melanoma by localizing and classifying tissues and cell nuclei is considered the gold standard method for diagnosis and treatment options for patients. While many computerized approaches have been proposed for automatic analysis, most perform tissue-based analysis and nuclei (cell)-based analysis as separate tasks, which might be suboptimal. In this work, using the PUMA challenge dataset, we proposed a novel multi-stage deep learning approach by combining tissue and nuclei information in a unified framework based on the auto-context concept to perform segmentation and classification in histological images of melanoma. Through pre-training and further post-processing, our approach achieved second and first place rankings in the PUMA challenge, with average micro Dice tissue score and summed nuclei F1-score of 73.40% for Track 1 and 63.48% for Track 2, respectively. Our implementation for training and testing is available at: https://github.com/NimaTorbati/PumaSubmit
* 15 pages
Via
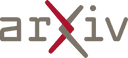
Apr 07, 2025
Abstract:Mohs micrographic surgery (MMS) is the gold standard technique for removing high risk nonmelanoma skin cancer however, intraoperative histopathological examination demands significant time, effort, and professionality. The objective of this study is to develop a deep learning model to detect basal cell carcinoma (BCC) and artifacts on Mohs slides. A total of 731 Mohs slides from 51 patients with BCCs were used in this study, with 91 containing tumor and 640 without tumor which was defined as non-tumor. The dataset was employed to train U-Net based models that segment tumor and non-tumor regions on the slides. The segmented patches were classified as tumor, or non-tumor to produce predictions for whole slide images (WSIs). For the segmentation phase, the deep learning model success was measured using a Dice score with 0.70 and 0.67 value, area under the curve (AUC) score with 0.98 and 0.96 for tumor and non-tumor, respectively. For the tumor classification, an AUC of 0.98 for patch-based detection, and AUC of 0.91 for slide-based detection was obtained on the test dataset. We present an AI system that can detect tumors and non-tumors in Mohs slides with high success. Deep learning can aid Mohs surgeons and dermatopathologists in making more accurate decisions.
* 14 pages, 2 figures
Via
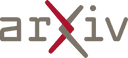
Mar 11, 2025
Abstract:Objective. This paper presents an overview of generalizable and explainable artificial intelligence (XAI) in deep learning (DL) for medical imaging, aimed at addressing the urgent need for transparency and explainability in clinical applications. Methodology. We propose to use four CNNs in three medical datasets (brain tumor, skin cancer, and chest x-ray) for medical image classification tasks. In addition, we perform paired t-tests to show the significance of the differences observed between different methods. Furthermore, we propose to combine ResNet50 with five common XAI techniques to obtain explainable results for model prediction, aiming at improving model transparency. We also involve a quantitative metric (confidence increase) to evaluate the usefulness of XAI techniques. Key findings. The experimental results indicate that ResNet50 can achieve feasible accuracy and F1 score in all datasets (e.g., 86.31\% accuracy in skin cancer). Furthermore, the findings show that while certain XAI methods, such as XgradCAM, effectively highlight relevant abnormal regions in medical images, others, like EigenGradCAM, may perform less effectively in specific scenarios. In addition, XgradCAM indicates higher confidence increase (e.g., 0.12 in glioma tumor) compared to GradCAM++ (0.09) and LayerCAM (0.08). Implications. Based on the experimental results and recent advancements, we outline future research directions to enhance the robustness and generalizability of DL models in the field of biomedical imaging.
* Published in Current Opinion in Biomedical Engineering
Via
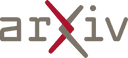
Feb 23, 2025
Abstract:We can achieve fast and consistent early skin cancer detection with recent developments in computer vision and deep learning techniques. However, the existing skin lesion segmentation and classification prediction models run independently, thus missing potential efficiencies from their integrated execution. To unify skin lesion analysis, our paper presents the Gaussian Splatting - Transformer UNet (GS-TransUNet), a novel approach that synergistically combines 2D Gaussian splatting with the Transformer UNet architecture for automated skin cancer diagnosis. Our unified deep learning model efficiently delivers dual-function skin lesion classification and segmentation for clinical diagnosis. Evaluated on ISIC-2017 and PH2 datasets, our network demonstrates superior performance compared to existing state-of-the-art models across multiple metrics through 5-fold cross-validation. Our findings illustrate significant advancements in the precision of segmentation and classification. This integration sets new benchmarks in the field and highlights the potential for further research into multi-task medical image analysis methodologies, promising enhancements in automated diagnostic systems.
* 12 pages, 7 figures, SPIE Medical Imaging 2025
Via
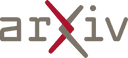
Jan 30, 2025
Abstract:With over 2 million new cases identified annually, skin cancer is the most prevalent type of cancer globally and the second most common in Bangladesh, following breast cancer. Early detection and treatment are crucial for enhancing patient outcomes; however, Bangladesh faces a shortage of dermatologists and qualified medical professionals capable of diagnosing and treating skin cancer. As a result, many cases are diagnosed only at advanced stages. Research indicates that deep learning algorithms can effectively classify skin cancer images. However, these models typically lack interpretability, making it challenging to understand their decision-making processes. This lack of clarity poses barriers to utilizing deep learning in improving skin cancer detection and treatment. In this article, we present a method aimed at enhancing the interpretability of deep learning models for skin cancer classification in Bangladesh. Our technique employs a combination of saliency maps and attention maps to visualize critical features influencing the model's diagnoses.
* 18 pages
Via
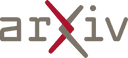
Feb 06, 2025
Abstract:Discriminative classifiers have become a foundational tool in deep learning for medical imaging, excelling at learning separable features of complex data distributions. However, these models often need careful design, augmentation, and training techniques to ensure safe and reliable deployment. Recently, diffusion models have become synonymous with generative modeling in 2D. These models showcase robustness across a range of tasks including natural image classification, where classification is performed by comparing reconstruction errors across images generated for each possible conditioning input. This work presents the first exploration of the potential of class conditional diffusion models for 2D medical image classification. First, we develop a novel majority voting scheme shown to improve the performance of medical diffusion classifiers. Next, extensive experiments on the CheXpert and ISIC Melanoma skin cancer datasets demonstrate that foundation and trained-from-scratch diffusion models achieve competitive performance against SOTA discriminative classifiers without the need for explicit supervision. In addition, we show that diffusion classifiers are intrinsically explainable, and can be used to quantify the uncertainty of their predictions, increasing their trustworthiness and reliability in safety-critical, clinical contexts. Further information is available on our project page: https://faverogian.github.io/med-diffusion-classifier.github.io/
Via
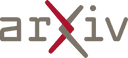
Jan 12, 2025
Abstract:As deep learning models gain attraction in medical data, ensuring transparent and trustworthy decision-making is essential. In skin cancer diagnosis, while advancements in lesion detection and classification have improved accuracy, the black-box nature of these methods poses challenges in understanding their decision processes, leading to trust issues among physicians. This study leverages the CLIP (Contrastive Language-Image Pretraining) model, trained on different skin lesion datasets, to capture meaningful relationships between visual features and diagnostic criteria terms. To further enhance transparency, we propose a method called MedGrad E-CLIP, which builds on gradient-based E-CLIP by incorporating a weighted entropy mechanism designed for complex medical imaging like skin lesions. This approach highlights critical image regions linked to specific diagnostic descriptions. The developed integrated pipeline not only classifies skin lesions by matching corresponding descriptions but also adds an essential layer of explainability developed especially for medical data. By visually explaining how different features in an image relates to diagnostic criteria, this approach demonstrates the potential of advanced vision-language models in medical image analysis, ultimately improving transparency, robustness, and trust in AI-driven diagnostic systems.
* Accepted to 2025 IEEE/CVF Winter Conference on Applications of
Computer Vision Workshops (WACVW)
Via
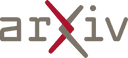
Jan 24, 2025
Abstract:Skin cancer is one of the most prevalent and potentially life-threatening diseases worldwide, necessitating early and accurate diagnosis to improve patient outcomes. Conventional diagnostic methods, reliant on clinical expertise and histopathological analysis, are often time-intensive, subjective, and prone to variability. To address these limitations, we propose a novel hybrid deep learning framework that integrates convolutional neural networks (CNNs) with Radial Basis Function (RBF) Networks to achieve high classification accuracy and enhanced interpretability. The motivation for incorporating RBF Networks lies in their intrinsic interpretability and localized response to input features, which make them well-suited for tasks requiring transparency and fine-grained decision-making. Unlike traditional deep learning models that rely on global feature representations, RBF Networks allow for mapping segments of images to chosen prototypes, exploiting salient features within a single image. This enables clinicians to trace predictions to specific, interpretable patterns. The framework incorporates segmentation-based feature extraction, active learning for prototype selection, and K-Medoids clustering to focus on these salient features. Evaluations on the ISIC 2016 and ISIC 2017 datasets demonstrate the model's effectiveness, achieving classification accuracies of 83.02\% and 72.15\% using ResNet50, respectively, and outperforming VGG16-based configurations. By generating interpretable explanations for predictions, the framework aligns with clinical workflows, bridging the gap between predictive performance and trustworthiness. This study highlights the potential of hybrid models to deliver actionable insights, advancing the development of reliable AI-assisted diagnostic tools for high-stakes medical applications.
* The paper has not been published by any journal/conference. It
contains 14 pages, with six figures and five tables to demonstrate results
Via
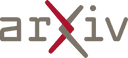