What is cancer detection? Cancer detection using Artificial Intelligence (AI) involves leveraging advanced machine learning algorithms and techniques to identify and diagnose cancer from various medical data sources. The goal is to enhance early detection, improve diagnostic accuracy, and potentially reduce the need for invasive procedures.
Papers and Code
Dec 11, 2024
Abstract:Introduction: Global burden of sexually transmitted infections (STIs) is rising out of proportion to specialists. Current chatbots like ChatGPT are not tailored for handling STI-related concerns out of the box. We developed Otiz, an Artificial Intelligence-based (AI-based) chatbot platform designed specifically for STI detection and counseling, and assessed its performance. Methods: Otiz employs a multi-agent system architecture based on GPT4-0613, leveraging large language model (LLM) and Deterministic Finite Automaton principles to provide contextually relevant, medically accurate, and empathetic responses. Its components include modules for general STI information, emotional recognition, Acute Stress Disorder detection, and psychotherapy. A question suggestion agent operates in parallel. Four STIs (anogenital warts, herpes, syphilis, urethritis/cervicitis) and 2 non-STIs (candidiasis, penile cancer) were evaluated using prompts mimicking patient language. Each prompt was independently graded by two venereologists conversing with Otiz as patient actors on 6 criteria using Numerical Rating Scale ranging from 0 (poor) to 5 (excellent). Results: Twenty-three venereologists did 60 evaluations of 30 prompts. Across STIs, Otiz scored highly on diagnostic accuracy (4.1-4.7), overall accuracy (4.3-4.6), correctness of information (5.0), comprehensibility (4.2-4.4), and empathy (4.5-4.8). However, relevance scores were lower (2.9-3.6), suggesting some redundancy. Diagnostic scores for non-STIs were lower (p=0.038). Inter-observer agreement was strong, with differences greater than 1 point occurring in only 12.7% of paired evaluations. Conclusions: AI conversational agents like Otiz can provide accurate, correct, discrete, non-judgmental, readily accessible and easily understandable STI-related information in an empathetic manner, and can alleviate the burden on healthcare systems.
* 18 pages, 1 table
Via
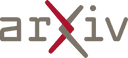
Nov 22, 2024
Abstract:Spread through air spaces (STAS) is a distinct invasion pattern in lung cancer, crucial for prognosis assessment and guiding surgical decisions. Histopathology is the gold standard for STAS detection, yet traditional methods are subjective, time-consuming, and prone to misdiagnosis, limiting large-scale applications. We present VERN, an image analysis model utilizing a feature-interactive Siamese graph encoder to predict STAS from lung cancer histopathological images. VERN captures spatial topological features with feature sharing and skip connections to enhance model training. Using 1,546 histopathology slides, we built a large single-cohort STAS lung cancer dataset. VERN achieved an AUC of 0.9215 in internal validation and AUCs of 0.8275 and 0.8829 in frozen and paraffin-embedded test sections, respectively, demonstrating clinical-grade performance. Validated on a single-cohort and three external datasets, VERN showed robust predictive performance and generalizability, providing an open platform (http://plr.20210706.xyz:5000/) to enhance STAS diagnosis efficiency and accuracy.
* accept for publication in npj Precision Oncology
Via
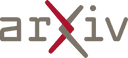
Nov 19, 2024
Abstract:In order to support the creation of reliable machine learning models for anomaly detection, this project focuses on preprocessing, enhancing, and organizing a medical imaging dataset. There are two classifications in the dataset: normal and abnormal, along with extra noise fluctuations. In order to improve the photographs' quality, undesirable artifacts, including visible medical equipment at the edges, were eliminated using central cropping. Adjusting the brightness and contrast was one of the additional preprocessing processes. Normalization was then performed to normalize the data. To make classification jobs easier, the dataset was methodically handled by combining several image subsets into two primary categories: normal and pathological. To provide a strong training set that adapts well to real-world situations, sophisticated picture preprocessing techniques were used, such as contrast enhancement and real-time augmentation (including rotations, zooms, and brightness modifications). To guarantee efficient model evaluation, the data was subsequently divided into training and testing subsets. In order to create precise and effective machine learning models for medical anomaly detection, high-quality input data is ensured via this thorough approach. Because of the project pipeline's flexible and scalable design, it can be easily integrated with bigger clinical decision-support systems.
Via
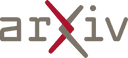
Dec 04, 2024
Abstract:Hepatocellular carcinoma (HCC) is a common type of liver cancer whose early-stage diagnosis is a common challenge, mainly due to the manual assessment of hematoxylin and eosin-stained whole slide images, which is a time-consuming process and may lead to variability in decision-making. For accurate detection of HCC, we propose a hybrid deep learning-based architecture that uses transfer learning to extract the features from pre-trained convolutional neural network (CNN) models and a classifier made up of a sequence of fully connected layers. This study uses a publicly available The Cancer Genome Atlas Hepatocellular Carcinoma (TCGA-LIHC)database (n=491) for model development and database of Kasturba Gandhi Medical College (KMC), India for validation. The pre-processing step involves patch extraction, colour normalization, and augmentation that results in 3920 patches for the TCGA dataset. The developed hybrid deep neural network consisting of a CNN-based pre-trained feature extractor and a customized artificial neural network-based classifier is trained using five-fold cross-validation. For this study, eight different state-of-the-art models are trained and tested as feature extractors for the proposed hybrid model. The proposed hybrid model with ResNet50-based feature extractor provided the sensitivity, specificity, F1-score, accuracy, and AUC of 100.00%, 100.00%, 100.00%, 100.00%, and 1.00, respectively on the TCGA database. On the KMC database, EfficientNetb3 resulted in the optimal choice of the feature extractor giving sensitivity, specificity, F1-score, accuracy, and AUC of 96.97, 98.85, 96.71, 96.71, and 0.99, respectively. The proposed hybrid models showed improvement in accuracy of 2% and 4% over the pre-trained models in TCGA-LIHC and KMC databases.
* 14 figure, 9 tables
Via
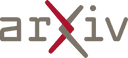
Nov 11, 2024
Abstract:Background. Breast cancer screening programs using mammography have led to significant mortality reduction in high-income countries. However, many low- and middle-income countries lack resources for mammographic screening. Handheld breast ultrasound (BUS) is a low-cost alternative but requires substantial training. Artificial intelligence (AI) enabled BUS may aid in both the detection (perception) and classification (interpretation) of breast cancer. Materials and Methods. This review (CRD42023493053) is reported in accordance with the PRISMA (Preferred Reporting Items for Systematic Reviews and Meta-Analysis) and SWiM (Synthesis Without Meta-analysis) guidelines. PubMed and Google Scholar were searched from January 1, 2016 to December 12, 2023. A meta-analysis was not attempted. Studies are grouped according to their AI task type, application time, and AI task. Study quality is assessed using the QUality Assessment of Diagnostic Accuracy Studies-2 (QUADAS-2) tool. Results. Of 763 candidate studies, 314 total full texts were reviewed. 34 studies are included. The AI tasks of included studies are as follows: 1 frame selection, 6 detection, 11 segmentation, and 16 classification. In total, 5.7 million BUS images from over 185,000 patients were used for AI training or validation. A single study included a prospective testing set. 79% of studies were at high or unclear risk of bias. Conclusion. There has been encouraging development of AI for BUS. Despite studies demonstrating high performance across all identified tasks, the evidence supporting AI-enhanced BUS generally lacks robustness. High-quality model validation will be key to realizing the potential for AI-enhanced BUS in increasing access to screening in resource-limited environments.
Via
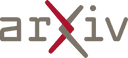
Nov 01, 2024
Abstract:Detecting genetic aberrations is crucial in cancer diagnosis, typically through fluorescence in situ hybridization (FISH). However, existing FISH image classification methods face challenges due to signal variability, the need for costly manual annotations and fail to adequately address the intrinsic uncertainty. We introduce a novel approach that leverages synthetic images to eliminate the requirement for manual annotations and utilizes a joint contrastive and classification objective for training to account for inter-class variation effectively. We demonstrate the superior generalization capabilities and uncertainty calibration of our method, which is trained on synthetic data, by testing it on a manually annotated dataset of real-world FISH images. Our model offers superior calibration in terms of classification accuracy and uncertainty quantification with a classification accuracy of 96.7% among the 50% most certain cases. The presented end-to-end method reduces the demands on personnel and time and improves the diagnostic workflow due to its accuracy and adaptability. All code and data is publicly accessible at: https://github.com/SimonBon/FISHing
* Uncertainty for Safe Utilization of Machine Learning in Medical
Imaging, 6th International Workshop, UNSURE 2024, Held in Conjunction with
MICCAI 2024, Marrakesh, Morocco, October 10, 2024, Pages 23-33
Via
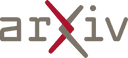
Oct 31, 2024
Abstract:Background: Mammographic breast density, as defined by the American College of Radiology's Breast Imaging Reporting and Data System (BI-RADS), is one of the strongest risk factors for breast cancer, but is derived from mammographic images. Breast ultrasound (BUS) is an alternative breast cancer screening modality, particularly useful for early detection in low-resource, rural contexts. The purpose of this study was to explore an artificial intelligence (AI) model to predict BI-RADS mammographic breast density category from clinical, handheld BUS imaging. Methods: All data are sourced from the Hawaii and Pacific Islands Mammography Registry. We compared deep learning methods from BUS imaging, as well as machine learning models from image statistics alone. The use of AI-derived BUS density as a risk factor for breast cancer was then compared to clinical BI-RADS breast density while adjusting for age. The BUS data were split by individual into 70/20/10% groups for training, validation, and testing. Results: 405,120 clinical BUS images from 14.066 women were selected for inclusion in this study, resulting in 9.846 women for training (302,574 images), 2,813 for validation (11,223 images), and 1,406 for testing (4,042 images). On the held-out testing set, the strongest AI model achieves AUROC 0.854 predicting BI-RADS mammographic breast density from BUS imaging and outperforms all shallow machine learning methods based on image statistics. In cancer risk prediction, age-adjusted AI BUS breast density predicted 5-year breast cancer risk with 0.633 AUROC, as compared to 0.637 AUROC from age-adjusted clinical breast density. Conclusions: BI-RADS mammographic breast density can be estimated from BUS imaging with high accuracy using a deep learning model. Furthermore, we demonstrate that AI-derived BUS breast density is predictive of 5-year breast cancer risk in our population.
Via
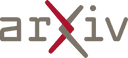
Nov 22, 2024
Abstract:Stereotactic Body Radiation Therapy (SBRT) can be a precise, minimally invasive treatment method for liver cancer and liver metastases. However, the effectiveness of SBRT relies on the accurate delivery of the dose to the tumor while sparing healthy tissue. Challenges persist in ensuring breath-hold reproducibility, with current methods often requiring manual verification of liver dome positions from kV-triggered images. To address this, we propose a proof-of-principle study of a deep learning-based pipeline to automatically delineate the liver dome from kV-planar images. From 24 patients who received SBRT for liver cancer or metastasis inside liver, 711 KV-triggered images acquired for online breath-hold verification were included in the current study. We developed a pipeline comprising a trained U-Net for automatic liver dome region segmentation from the triggered images followed by extraction of the liver dome via thresholding, edge detection, and morphological operations. The performance and generalizability of the pipeline was evaluated using 2-fold cross validation. The training of the U-Net model for liver region segmentation took under 30 minutes and the automatic delineation of a liver dome for any triggered image took less than one second. The RMSE and rate of detection for Fold1 with 366 images was (6.4 +/- 1.6) mm and 91.7%, respectively. For Fold2 with 345 images, the RMSE and rate of detection was (7.7 +/- 2.3) mm and 76.3% respectively.
Via
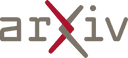
Nov 26, 2024
Abstract:Precise breast cancer classification on histopathological images has the potential to greatly improve the diagnosis and patient outcome in oncology. The data imbalance problem largely stems from the inherent imbalance within medical image datasets, where certain tumor subtypes may appear much less frequently. This constitutes a considerable limitation in biased model predictions that can overlook critical but rare classes. In this work, we adopted EfficientNet, a state-of-the-art convolutional neural network (CNN) model that balances high accuracy with computational cost efficiency. To address data imbalance, we introduce an intensive data augmentation pipeline and cost-sensitive learning, improving representation and ensuring that the model does not overly favor majority classes. This approach provides the ability to learn effectively from rare tumor types, improving its robustness. Additionally, we fine-tuned the model using transfer learning, where weights in the beginning trained on a binary classification task were adopted to multi-class classification, improving the capability to detect complex patterns within the BreakHis dataset. Our results underscore significant improvements in the binary classification performance, achieving an exceptional recall increase for benign cases from 0.92 to 0.95, alongside an accuracy enhancement from 97.35 % to 98.23%. Our approach improved the performance of multi-class tasks from 91.27% with regular augmentation to 94.54% with intensive augmentation, reaching 95.04% with transfer learning. This framework demonstrated substantial gains in precision in the minority classes, such as Mucinous carcinoma and Papillary carcinoma, while maintaining high recall consistently across these critical subtypes, as further confirmed by confusion matrix analysis.
* 19 pages, 7 figures
Via
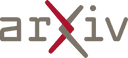
Nov 01, 2024
Abstract:To automatically detect Anterior Mediastinum Lesions (AMLs) in the Anterior Mediastinum (AM), the primary requirement will be an automatic segmentation model specifically designed for the AM. The prevalence of AML is extremely low, making it challenging to conduct screening research similar to lung cancer screening. Retrospectively reviewing chest CT scans over a specific period to investigate the prevalence of AML requires substantial time. Therefore, developing an Artificial Intelligence (AI) model to find location of AM helps radiologist to enhance their ability to manage workloads and improve diagnostic accuracy for AMLs. In this paper, we introduce a U-shaped structure network to segment AM. Two attention mechanisms were used for maintaining long-range dependencies and localization. In order to have the potential of Multi-Head Self-Attention (MHSA) and a lightweight network, we designed a parallel MHSA named Wide-MHSA (W-MHSA). Maintaining long-range dependencies is crucial for segmentation when we upsample feature maps. Therefore, we designed a Dilated Depth-Wise Parallel Path connection (DDWPP) for this purpose. In order to design a lightweight architecture, we introduced an expanding convolution block and combine it with the proposed W-MHSA for feature extraction in the encoder part of the proposed U-shaped network. The proposed network was trained on 2775 AM cases, which obtained an average Dice Similarity Coefficient (DSC) of 87.83%, mean Intersection over Union (IoU) of 79.16%, and Sensitivity of 89.60%. Our proposed architecture exhibited superior segmentation performance compared to the most advanced segmentation networks, such as Trans Unet, Attention Unet, Res Unet, and Res Unet++.
Via
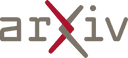