What is cancer detection? Cancer detection using Artificial Intelligence (AI) involves leveraging advanced machine learning algorithms and techniques to identify and diagnose cancer from various medical data sources. The goal is to enhance early detection, improve diagnostic accuracy, and potentially reduce the need for invasive procedures.
Papers and Code
Dec 12, 2024
Abstract:Pathological diagnosis is vital for determining disease characteristics, guiding treatment, and assessing prognosis, relying heavily on detailed, multi-scale analysis of high-resolution whole slide images (WSI). However, traditional pure vision models face challenges of redundant feature extraction, whereas existing large vision-language models (LVLMs) are limited by input resolution constraints, hindering their efficiency and accuracy. To overcome these issues, we propose two innovative strategies: the mixed task-guided feature enhancement, which directs feature extraction toward lesion-related details across scales, and the prompt-guided detail feature completion, which integrates coarse- and fine-grained features from WSI based on specific prompts without compromising inference speed. Leveraging a comprehensive dataset of 490,000 samples from diverse pathology tasks-including cancer detection, grading, vascular and neural invasion identification, and so on-we trained the pathology-specialized LVLM, OmniPath. Extensive experiments demonstrate that this model significantly outperforms existing methods in diagnostic accuracy and efficiency, offering an interactive, clinically aligned approach for auxiliary diagnosis in a wide range of pathology applications.
Via
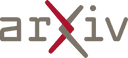
Nov 30, 2024
Abstract:We focus on the problem of Gallbladder Cancer (GBC) detection from Ultrasound (US) images. The problem presents unique challenges to modern Deep Neural Network (DNN) techniques due to low image quality arising from noise, textures, and viewpoint variations. Tackling such challenges would necessitate precise localization performance by the DNN to identify the discerning features for the downstream malignancy prediction. While several techniques have been proposed in the recent years for the problem, all of these methods employ complex custom architectures. Inspired by the success of foundational models for natural image tasks, along with the use of adapters to fine-tune such models for the custom tasks, we investigate the merit of one such design, ViT-Adapter, for the GBC detection problem. We observe that ViT-Adapter relies predominantly on a primitive CNN-based spatial prior module to inject the localization information via cross-attention, which is inefficient for our problem due to the small pathology sizes, and variability in their appearances due to non-regular structure of the malignancy. In response, we propose, LQ-Adapter, a modified Adapter design for ViT, which improves localization information by leveraging learnable content queries over the basic spatial prior module. Our method surpasses existing approaches, enhancing the mean IoU (mIoU) scores by 5.4%, 5.8%, and 2.7% over ViT-Adapters, DINO, and FocalNet-DINO, respectively on the US image-based GBC detection dataset, and establishing a new state-of-the-art (SOTA). Additionally, we validate the applicability and effectiveness of LQ-Adapter on the Kvasir-Seg dataset for polyp detection from colonoscopy images. Superior performance of our design on this problem as well showcases its capability to handle diverse medical imaging tasks across different datasets. Code is released at https://github.com/ChetanMadan/LQ-Adapter
* Accepted at WACV 2025
Via
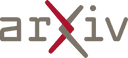
Nov 16, 2024
Abstract:While state-of-the-art models for breast cancer detection leverage multi-view mammograms for enhanced diagnostic accuracy, they often focus solely on visual mammography data. However, radiologists document valuable lesion descriptors that contain additional information that can enhance mammography-based breast cancer screening. A key question is whether deep learning models can benefit from these expert-derived features. To address this question, we introduce a novel multi-modal approach that combines textual BI-RADS lesion descriptors with visual mammogram content. Our method employs iterative attention layers to effectively fuse these different modalities, significantly improving classification performance over image-only models. Experiments on the CBIS-DDSM dataset demonstrate substantial improvements across all metrics, demonstrating the contribution of handcrafted features to end-to-end.
Via
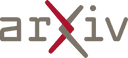
Jan 12, 2025
Abstract:Patients with metastatic breast cancer (mBC) undergo continuous medical imaging during treatment, making accurate lesion detection and monitoring over time critical for clinical decisions. Predicting drug response from post-treatment data is essential for personalized care and pharmacological research. In collaboration with the U.S. Food and Drug Administration and Novartis Pharmaceuticals, we analyzed serial chest CT scans from two large-scale Phase III trials, MONALEESA 3 and MONALEESA 7. This paper has two objectives (a) Data Structuring developing a Registration Aided Automated Correspondence (RAMAC) algorithm for precise lesion tracking in longitudinal CT data, and (b) Survival Analysis creating imaging features and models from RAMAC structured data to predict patient outcomes. The RAMAC algorithm uses a two phase pipeline: three dimensional rigid registration aligns CT images, and a distance metric-based Hungarian algorithm tracks lesion correspondence. Using structured data, we developed interpretable models to assess progression-free survival (PFS) in mBC patients by combining baseline radiomics, post-treatment changes (Weeks 8, 16, 24), and demographic features. Radiomics effects were studied across time points separately and through a non-correlated additive framework. Radiomics features were reduced using (a) a regularized (L1-penalized) additive Cox proportional hazards model, and (b) variable selection via best subset selection. Performance, measured using the concordance index (C-index), improved with additional time points. Joint modeling, considering correlations among radiomics effects over time, provided insights into relationships between longitudinal radiomics and survival outcomes.
* 20 pages, 13 figures, 2 tables
Via
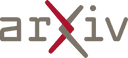
Nov 14, 2024
Abstract:Domain generalisation in computational histopathology is challenging because the images are substantially affected by differences among hospitals due to factors like fixation and staining of tissue and imaging equipment. We hypothesise that focusing on nuclei can improve the out-of-domain (OOD) generalisation in cancer detection. We propose a simple approach to improve OOD generalisation for cancer detection by focusing on nuclear morphology and organisation, as these are domain-invariant features critical in cancer detection. Our approach integrates original images with nuclear segmentation masks during training, encouraging the model to prioritise nuclei and their spatial arrangement. Going beyond mere data augmentation, we introduce a regularisation technique that aligns the representations of masks and original images. We show, using multiple datasets, that our method improves OOD generalisation and also leads to increased robustness to image corruptions and adversarial attacks. The source code is available at https://github.com/undercutspiky/SFL/
* Poster at NeurIPS 2024
Via
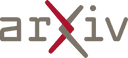
Dec 23, 2024
Abstract:Lung and colon cancers are predominant contributors to cancer mortality. Early and accurate diagnosis is crucial for effective treatment. By utilizing imaging technology in different image detection, learning models have shown promise in automating cancer classification from histopathological images. This includes the histopathological diagnosis, an important factor in cancer type identification. This research focuses on creating a high-efficiency deep-learning model for identifying lung and colon cancer from histopathological images. We proposed a novel approach based on a modified residual attention network architecture. The model was trained on a dataset of 25,000 high-resolution histopathological images across several classes. Our proposed model achieved an exceptional accuracy of 99.30%, 96.63%, and 97.56% for two, three, and five classes, respectively; those are outperforming other state-of-the-art architectures. This study presents a highly accurate deep learning model for lung and colon cancer classification. The superior performance of our proposed model addresses a critical need in medical AI applications.
Via
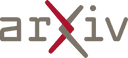
Dec 11, 2024
Abstract:Polyp segmentation in colonoscopy is crucial for detecting colorectal cancer. However, it is challenging due to variations in the structure, color, and size of polyps, as well as the lack of clear boundaries with surrounding tissues. Traditional segmentation models based on Convolutional Neural Networks (CNNs) struggle to capture detailed patterns and global context, limiting their performance. Vision Transformer (ViT)-based models address some of these issues but have difficulties in capturing local context and lack strong zero-shot generalization. To this end, we propose the Mamba-guided Segment Anything Model (SAM-Mamba) for efficient polyp segmentation. Our approach introduces a Mamba-Prior module in the encoder to bridge the gap between the general pre-trained representation of SAM and polyp-relevant trivial clues. It injects salient cues of polyp images into the SAM image encoder as a domain prior while capturing global dependencies at various scales, leading to more accurate segmentation results. Extensive experiments on five benchmark datasets show that SAM-Mamba outperforms traditional CNN, ViT, and Adapter-based models in both quantitative and qualitative measures. Additionally, SAM-Mamba demonstrates excellent adaptability to unseen datasets, making it highly suitable for real-time clinical use.
Via
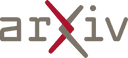
Nov 11, 2024
Abstract:Breast cancer detection is still an open research field, despite a tremendous effort devoted to work in this area. Effect size is a statistical concept that measures the strength of the relationship between two variables on a numeric scale. Feature selection is widely used to reduce the dimensionality of data by selecting only a subset of predictor variables to improve a learning model. In this work, an algorithm and experimental results demonstrate the feasibility of developing a statistical feature-selector-based learning tool capable of reducing the data dimensionality using parametric effect size measures from features extracted from cell nuclei images. The SVM classifier with a linear kernel as a learning tool achieved an accuracy of over 90%. These excellent results suggest that the effect size is within the standards of the feature-selector methods
* 16 pages, 10 figures, 5 tables,2024 IEEE Biennial Congress of
Argentina (ARGENCON)
Via
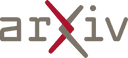
Nov 30, 2024
Abstract:Skin cancer (SC) stands out as one of the most life-threatening forms of cancer, with its danger amplified if not diagnosed and treated promptly. Early intervention is critical, as it allows for more effective treatment approaches. In recent years, Deep Learning (DL) has emerged as a powerful tool in the early detection and skin cancer diagnosis (SCD). Although the DL seems promising for the diagnosis of skin cancer, still ample scope exists for improving model efficiency and accuracy. This paper proposes a novel approach to skin cancer detection, utilizing optimization techniques in conjunction with pre-trained networks and wavelet transformations. First, normalized images will undergo pre-trained networks such as Densenet-121, Inception, Xception, and MobileNet to extract hierarchical features from input images. After feature extraction, the feature maps are passed through a Discrete Wavelet Transform (DWT) layer to capture low and high-frequency components. Then the self-attention module is integrated to learn global dependencies between features and focus on the most relevant parts of the feature maps. The number of neurons and optimization of the weight vectors are performed using three new swarm-based optimization techniques, such as Modified Gorilla Troops Optimizer (MGTO), Improved Gray Wolf Optimization (IGWO), and Fox optimization algorithm. Evaluation results demonstrate that optimizing weight vectors using optimization algorithms can enhance diagnostic accuracy and make it a highly effective approach for SCD. The proposed method demonstrates substantial improvements in accuracy, achieving top rates of 98.11% with the MobileNet + Wavelet + FOX and DenseNet + Wavelet + Fox combination on the ISIC-2016 dataset and 97.95% with the Inception + Wavelet + MGTO combination on the ISIC-2017 dataset, which improves accuracy by at least 1% compared to other methods.
Via
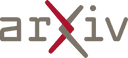
Jan 10, 2025
Abstract:The skin, as the largest organ of the human body, is vulnerable to a diverse array of conditions collectively known as skin lesions, which encompass various dermatoses. Diagnosing these lesions presents significant challenges for medical practitioners due to the subtle visual differences that are often imperceptible to the naked eye. While not all skin lesions are life-threatening, certain types can act as early indicators of severe diseases, including skin cancers, underscoring the critical need for timely and accurate diagnostic methods. Deep learning algorithms have demonstrated remarkable potential in facilitating the early detection and prognosis of skin lesions. This study advances the field by curating a comprehensive and diverse dataset comprising 39 categories of skin lesions, synthesized from five publicly available datasets. Using this dataset, the performance of five state-of-the-art deep learning models -- MobileNetV2, Xception, InceptionV3, EfficientNetB1, and Vision Transformer - is rigorously evaluated. To enhance the accuracy and robustness of these models, attention mechanisms such as the Efficient Channel Attention (ECA) and the Convolutional Block Attention Module (CBAM) are incorporated into their architectures. Comprehensive evaluation across multiple performance metrics reveals that the Vision Transformer model integrated with CBAM outperforms others, achieving an accuracy of 93.46%, precision of 94%, recall of 93%, F1-score of 93%, and specificity of 93.67%. These results underscore the significant potential of the proposed system in supporting medical professionals with accurate and efficient prognostic tools for diagnosing a broad spectrum of skin lesions. The dataset and code used in this study can be found at https://github.com/akabircs/Skin-Lesions-Classification.
* 26 pages
Via
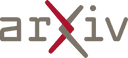