What is autonomous cars? Autonomous cars are self-driving vehicles that use artificial intelligence (AI) and sensors to navigate and operate without human intervention, using high-resolution cameras and lidars that detect what happens in the car's immediate surroundings. They have the potential to revolutionize transportation by improving safety, efficiency, and accessibility.
Papers and Code
Aug 01, 2024
Abstract:This paper introduces a novel nonlinear stochastic model predictive control path integral (MPPI) method, which considers chance constraints on system states. The proposed belief-space stochastic MPPI (BSS-MPPI) applies Monte-Carlo sampling to evaluate state distributions resulting from underlying systematic disturbances, and utilizes a Control Barrier Function (CBF) inspired heuristic in belief space to fulfill the specified chance constraints. Compared to several previous stochastic predictive control methods, our approach applies to general nonlinear dynamics without requiring the computationally expensive system linearization step. Moreover, the BSS-MPPI controller can solve optimization problems without limiting the form of the objective function and chance constraints. By multi-threading the sampling process using a GPU, we can achieve fast real-time planning for time- and safety-critical tasks such as autonomous racing. Our results on a realistic race-car simulation study show significant reductions in constraint violation compared to some of the prior MPPI approaches, while being comparable in computation times.
Via
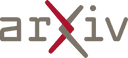
Sep 14, 2024
Abstract:Human-level autonomous driving is an ever-elusive goal, with planning and decision making -- the cognitive functions that determine driving behavior -- posing the greatest challenge. Despite a proliferation of promising approaches, progress is stifled by the difficulty of deploying experimental planners in naturalistic settings. In this work, we propose Lab2Car, an optimization-based wrapper that can take a trajectory sketch from an arbitrary motion planner and convert it to a safe, comfortable, dynamically feasible trajectory that the car can follow. This allows motion planners that do not provide such guarantees to be safely tested and optimized in real-world environments. We demonstrate the versatility of Lab2Car by using it to deploy a machine learning (ML) planner and a search-based planner on self-driving cars in Las Vegas. The resulting systems handle challenging scenarios, such as cut-ins, overtaking, and yielding, in complex urban environments like casino pick-up/drop-off areas. Our work paves the way for quickly deploying and evaluating candidate motion planners in realistic settings, ensuring rapid iteration and accelerating progress towards human-level autonomy.
* This work has been submitted to the IEEE for possible publication.
Copyright may be transferred without notice, after which this version may no
longer be accessible
Via
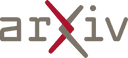
Aug 12, 2024
Abstract:This work presents the design and development of IIT Bombay Racing's Formula Student style autonomous racecar algorithm capable of running at the racing events of Formula Student-AI, held in the UK. The car employs a cutting-edge sensor suite of the compute unit NVIDIA Jetson Orin AGX, 2 ZED2i stereo cameras, 1 Velodyne Puck VLP16 LiDAR and SBG Systems Ellipse N GNSS/INS IMU. It features deep learning algorithms and control systems to navigate complex tracks and execute maneuvers without any human intervention. The design process involved extensive simulations and testing to optimize the vehicle's performance and ensure its safety. The algorithms have been tested on a small scale, in-house manufactured 4-wheeled robot and on simulation software. The results obtained for testing various algorithms in perception, simultaneous localization and mapping, path planning and controls have been detailed.
* 8 pages, 19 figures
Via
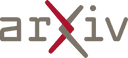
Jul 08, 2024
Abstract:Accurate modeling of car-following behaviors is essential for various applications in traffic management and autonomous driving systems. However, current approaches often suffer from limitations like high sensitivity to data quality and lack of interpretability. In this study, we propose GenFollower, a novel zero-shot prompting approach that leverages large language models (LLMs) to address these challenges. We reframe car-following behavior as a language modeling problem and integrate heterogeneous inputs into structured prompts for LLMs. This approach achieves improved prediction performance and interpretability compared to traditional baseline models. Experiments on the Waymo Open datasets demonstrate GenFollower's superior performance and ability to provide interpretable insights into factors influencing car-following behavior. This work contributes to advancing the understanding and prediction of car-following behaviors, paving the way for enhanced traffic management and autonomous driving systems.
Via
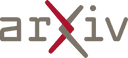
Apr 26, 2024
Abstract:Autonomous Vehicles (AVs) redefine transportation with sophisticated technology, integrating sensors, cameras, and intricate algorithms. Implementing machine learning in AV perception demands robust hardware accelerators to achieve real-time performance at reasonable power consumption and footprint. Lot of research and development efforts using different technologies are still being conducted to achieve the goal of getting a fully AV and some cars manufactures offer commercially available systems. Unfortunately, they still lack reliability because of the repeated accidents they have encountered such as the recent one which happened in California and for which the Cruise company had its license suspended by the state of California for an undetermined period [1]. This paper critically reviews the most recent findings of machine vision systems used in AVs from both hardware and algorithmic points of view. It discusses the technologies used in commercial cars with their pros and cons and suggests possible ways forward. Thus, the paper can be a tangible reference for researchers who have the opportunity to get involved in designing machine vision systems targeting AV
* 14 pages, 15 figures, 4 tables
Via
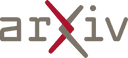
Sep 25, 2024
Abstract:Developing efficient traffic models is essential for optimizing transportation systems, yet current approaches remain time-intensive and susceptible to human errors due to their reliance on manual processes. Traditional workflows involve exhaustive literature reviews, formula optimization, and iterative testing, leading to inefficiencies in research. In response, we introduce the Traffic Research Agent (TR-Agent), an AI-driven system designed to autonomously develop and refine traffic models through an iterative, closed-loop process. Specifically, we divide the research pipeline into four key stages: idea generation, theory formulation, theory evaluation, and iterative optimization; and construct TR-Agent with four corresponding modules: Idea Generator, Code Generator, Evaluator, and Analyzer. Working in synergy, these modules retrieve knowledge from external resources, generate novel ideas, implement and debug models, and finally assess them on the evaluation datasets. Furthermore, the system continuously refines these models based on iterative feedback, enhancing research efficiency and model performance. Experimental results demonstrate that TR-Agent achieves significant performance improvements across multiple traffic models, including the Intelligent Driver Model (IDM) for car following, the MOBIL lane-changing model, and the Lighthill-Whitham-Richards (LWR) traffic flow model. Additionally, TR-Agent provides detailed explanations for its optimizations, allowing researchers to verify and build upon its improvements easily. This flexibility makes the framework a powerful tool for researchers in transportation and beyond. To further support research and collaboration, we have open-sourced both the code and data used in our experiments, facilitating broader access and enabling continued advancements in the field.
* 19 pages, 10 figures
Via
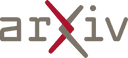
Sep 08, 2024
Abstract:Cooperative perception through vehicle-to-everything (V2X) has garnered significant attention in recent years due to its potential to overcome occlusions and enhance long-distance perception. Great achievements have been made in both datasets and algorithms. However, existing real-world datasets are limited by the presence of few communicable agents, while synthetic datasets typically cover only vehicles. More importantly, the penetration rate of connected and autonomous vehicles (CAVs) , a critical factor for the deployment of cooperative perception technologies, has not been adequately addressed. To tackle these issues, we introduce Multi-V2X, a large-scale, multi-modal, multi-penetration-rate dataset for V2X perception. By co-simulating SUMO and CARLA, we equip a substantial number of cars and roadside units (RSUs) in simulated towns with sensor suites, and collect comprehensive sensing data. Datasets with specified CAV penetration rates can be obtained by masking some equipped cars as normal vehicles. In total, our Multi-V2X dataset comprises 549k RGB frames, 146k LiDAR frames, and 4,219k annotated 3D bounding boxes across six categories. The highest possible CAV penetration rate reaches 86.21%, with up to 31 agents in communication range, posing new challenges in selecting agents to collaborate with. We provide comprehensive benchmarks for cooperative 3D object detection tasks. Our data and code are available at https://github.com/RadetzkyLi/Multi-V2X .
* 9 pages, 4 figures, 5 tables
Via
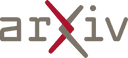
Jul 10, 2024
Abstract:This study introduces the Misclassification Likelihood Matrix (MLM) as a novel tool for quantifying the reliability of neural network predictions under distribution shifts. The MLM is obtained by leveraging softmax outputs and clustering techniques to measure the distances between the predictions of a trained neural network and class centroids. By analyzing these distances, the MLM provides a comprehensive view of the model's misclassification tendencies, enabling decision-makers to identify the most common and critical sources of errors. The MLM allows for the prioritization of model improvements and the establishment of decision thresholds based on acceptable risk levels. The approach is evaluated on the MNIST dataset using a Convolutional Neural Network (CNN) and a perturbed version of the dataset to simulate distribution shifts. The results demonstrate the effectiveness of the MLM in assessing the reliability of predictions and highlight its potential in enhancing the interpretability and risk mitigation capabilities of neural networks. The implications of this work extend beyond image classification, with ongoing applications in autonomous systems, such as self-driving cars, to improve the safety and reliability of decision-making in complex, real-world environments.
* 8 pages, 7 figures, 1 table
Via
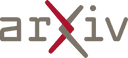
May 17, 2024
Abstract:Safe navigation in unknown environments stands as a significant challenge in the field of robotics. Control Barrier Function (CBF) is a strong mathematical tool to guarantee safety requirements. However, a common assumption in many works is that the CBF is already known and obstacles have predefined shapes. In this letter, we present a novel method called Occupancy Grid Map-based Control Barrier Function (OGM-CBF), which defines Control Barrier Function based on Occupancy Grid Maps. This enables generalization to unknown environments while generating online local or global maps of the environment using onboard perception sensors such as LiDAR or camera. With this method, the system guarantees safety via a single, continuously differentiable CBF per time step, which can be represented as one constraint in the CBF-QP optimization formulation while having an arbitrary number of obstacles with unknown shapes in the environment. This enables practical real-time implementation of CBF in both unknown and known environments. The efficacy of OGM-CBF is demonstrated in the safe control of an autonomous car in the CARLA simulator and a real-world industrial mobile robot.
Via
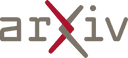
Jul 12, 2024
Abstract:Data for training learning-enabled self-driving cars in the physical world are typically collected in a safe, normal environment. Such data distribution often engenders a strong bias towards safe driving, making self-driving cars unprepared when encountering adversarial scenarios like unexpected accidents. Due to a dearth of such adverse data that is unrealistic for drivers to collect, autonomous vehicles can perform poorly when experiencing such rare events. This work addresses much-needed research by having participants drive a VR vehicle simulator going through simulated traffic with various types of accidental scenarios. It aims to understand human responses and behaviors in simulated accidents, contributing to our understanding of driving dynamics and safety. The simulation framework adopts a robust traffic simulation and is rendered using the Unity Game Engine. Furthermore, the simulation framework is built with portable, light-weight immersive driving simulator hardware, lowering the resource barrier for studies in autonomous driving research. Keywords: Rare Events, Traffic Simulation, Autonomous Driving, Virtual Reality, User Studies
Via
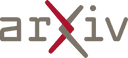