What is Sentiment Analysis? Sentiment analysis is the process of determining the sentiment of a piece of text, such as a tweet or a review.
Papers and Code
May 22, 2025
Abstract:The advancements in Multimodal Large Language Models (MLLMs) have enabled various multimodal tasks to be addressed under a zero-shot paradigm. This paradigm sidesteps the cost of model fine-tuning, emerging as a dominant trend in practical application. Nevertheless, Multimodal Sentiment Analysis (MSA), a pivotal challenge in the quest for general artificial intelligence, fails to accommodate this convenience. The zero-shot paradigm exhibits undesirable performance on MSA, casting doubt on whether MLLMs can perceive sentiments as competent as supervised models. By extending the zero-shot paradigm to In-Context Learning (ICL) and conducting an in-depth study on configuring demonstrations, we validate that MLLMs indeed possess such capability. Specifically, three key factors that cover demonstrations' retrieval, presentation, and distribution are comprehensively investigated and optimized. A sentimental predictive bias inherent in MLLMs is also discovered and later effectively counteracted. By complementing each other, the devised strategies for three factors result in average accuracy improvements of 15.9% on six MSA datasets against the zero-shot paradigm and 11.2% against the random ICL baseline.
Via
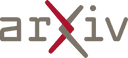
May 21, 2025
Abstract:Sarcasm is a challenge to sentiment analysis because of the incongruity between stated and implied sentiment. The challenge is exacerbated when the implication may be relevant to a specific country or geographical region. Pragmatic metacognitive prompting (PMP) is a cognition-inspired technique that has been used for pragmatic reasoning. In this paper, we harness PMP for explainable sarcasm detection for Australian and Indian English, alongside a benchmark dataset for standard English. We manually add sarcasm explanations to an existing sarcasm-labeled dataset for Australian and Indian English called BESSTIE, and compare the performance for explainable sarcasm detection for them with FLUTE, a standard English dataset containing sarcasm explanations. Our approach utilising PMP when evaluated on two open-weight LLMs (GEMMA and LLAMA) achieves statistically significant performance improvement across all tasks and datasets when compared with four alternative prompting strategies. We also find that alternative techniques such as agentic prompting mitigate context-related failures by enabling external knowledge retrieval. The focused contribution of our work is utilising PMP in generating sarcasm explanations for varieties of English.
* Under review. 4 pages + references
Via
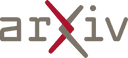
May 15, 2025
Abstract:This paper explores the design of an aspect-based sentiment analysis system using large language models (LLMs) for real-world use. We focus on quadruple opinion extraction -- identifying aspect categories, sentiment polarity, targets, and opinion expressions from text data across different domains and languages. Using internal datasets, we investigate whether a single fine-tuned model can effectively handle multiple domain-specific taxonomies simultaneously. We demonstrate that a combined multi-domain model achieves performance comparable to specialized single-domain models while reducing operational complexity. We also share lessons learned for handling non-extractive predictions and evaluating various failure modes when developing LLM-based systems for structured prediction tasks.
Via
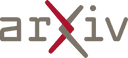
May 26, 2025
Abstract:Political biases encoded by LLMs might have detrimental effects on downstream applications. Existing bias analysis methods rely on small-size intermediate tasks (questionnaire answering or political content generation) and rely on the LLMs themselves for analysis, thus propagating bias. We propose a new approach leveraging the observation that LLM sentiment predictions vary with the target entity in the same sentence. We define an entropy-based inconsistency metric to encode this prediction variability. We insert 1319 demographically and politically diverse politician names in 450 political sentences and predict target-oriented sentiment using seven models in six widely spoken languages. We observe inconsistencies in all tested combinations and aggregate them in a statistically robust analysis at different granularity levels. We observe positive and negative bias toward left and far-right politicians and positive correlations between politicians with similar alignment. Bias intensity is higher for Western languages than for others. Larger models exhibit stronger and more consistent biases and reduce discrepancies between similar languages. We partially mitigate LLM unreliability in target-oriented sentiment classification (TSC) by replacing politician names with fictional but plausible counterparts.
* To be published in the Proceedings of the 63rd Annual Meeting of the
Association for Computational Linguistics (ACL 2025)
Via
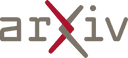
May 17, 2025
Abstract:In today's digitally-driven world, the demand for personalized and context-aware recommendations has never been greater. Traditional recommender systems have made significant strides in this direction, but they often lack the ability to tap into the richness of conversational data. This paper represents a novel approach to recommendation systems by integrating conversational insights into the recommendation process. The Conversational Recommender System integrates cutting-edge technologies such as deep learning, leveraging machine learning algorithms like Apriori for Association Rule Mining, Convolutional Neural Networks (CNN), Recurrent Neural Networks (RNN), and Long Short-Term Memory (LTSM). Furthermore, sophisticated voice recognition technologies, including Hidden Markov Models (HMMs) and Dynamic Time Warping (DTW) algorithms, play a crucial role in accurate speech-to-text conversion, ensuring robust performance in diverse environments. The methodology incorporates a fusion of content-based and collaborative recommendation approaches, enhancing them with NLP techniques. This innovative integration ensures a more personalized and context-aware recommendation experience, particularly in marketing applications.
* Presented in ISETE conference (International Conference on Artificial
Intelligence, Machine Learning and Big Data Engineering 2024)
Via
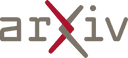
May 17, 2025
Abstract:Social media platforms like X (formerly Twitter) play a crucial role in shaping public discourse and societal norms. This study examines the term Sessiz Istila (Silent Invasion) on Turkish social media, highlighting the rise of anti-refugee sentiment amidst the Syrian refugee influx. Using BERTurk and the TREMO dataset, we developed an advanced Emotion Recognition Model (ERM) tailored for Turkish, achieving 92.62% accuracy in categorizing emotions such as happiness, fear, anger, sadness, disgust, and surprise. By applying this model to large-scale X data, the study uncovers emotional nuances in Turkish discourse, contributing to computational social science by advancing sentiment analysis in underrepresented languages and enhancing our understanding of global digital discourse and the unique linguistic challenges of Turkish. The findings underscore the transformative potential of localized NLP tools, with our ERM model offering practical applications for real-time sentiment analysis in Turkish-language contexts. By addressing critical areas, including marketing, public relations, and crisis management, these models facilitate improved decision-making through timely and accurate sentiment tracking. This highlights the significance of advancing research that accounts for regional and linguistic nuances.
Via
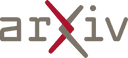
May 12, 2025
Abstract:The emergence of global health crises, such as COVID-19 and Monkeypox (mpox), has underscored the importance of understanding public sentiment to inform effective public health strategies. This study conducts a comparative sentiment analysis of public perceptions surrounding COVID-19 and mpox by leveraging extensive datasets of 147,475 and 106,638 tweets, respectively. Advanced machine learning models, including Logistic Regression, Naive Bayes, RoBERTa, DistilRoBERTa and XLNet, were applied to perform sentiment classification, with results indicating key trends in public emotion and discourse. The analysis highlights significant differences in public sentiment driven by disease characteristics, media representation, and pandemic fatigue. Through the lens of sentiment polarity and thematic trends, this study offers valuable insights into tailoring public health messaging, mitigating misinformation, and fostering trust during concurrent health crises. The findings contribute to advancing sentiment analysis applications in public health informatics, setting the groundwork for enhanced real-time monitoring and multilingual analysis in future research.
Via
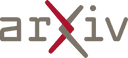
May 16, 2025
Abstract:This paper presents an end-to-end suite for multilingual information extraction and processing from image-based documents. The system uses Optical Character Recognition (Tesseract) to extract text in languages such as English, Hindi, and Tamil, and then a pipeline involving large language model APIs (Gemini) for cross-lingual translation, abstractive summarization, and re-translation into a target language. Additional modules add sentiment analysis (TensorFlow), topic classification (Transformers), and date extraction (Regex) for better document comprehension. Made available in an accessible Gradio interface, the current research shows a real-world application of libraries, models, and APIs to close the language gap and enhance access to information in image media across different linguistic environments
* 8 pages, 7 figures, direct arXiv submission
Via
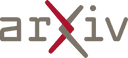
May 29, 2025
Abstract:Opinion mining plays a vital role in analysing user feedback and extracting insights from textual data. While most research focuses on sentiment polarity (e.g., positive, negative, neutral), fine-grained emotion classification in app reviews remains underexplored. This paper addresses this gap by identifying and addressing the challenges and limitations in fine-grained emotion analysis in the context of app reviews. Our study adapts Plutchik's emotion taxonomy to app reviews by developing a structured annotation framework and dataset. Through an iterative human annotation process, we define clear annotation guidelines and document key challenges in emotion classification. Additionally, we evaluate the feasibility of automating emotion annotation using large language models, assessing their cost-effectiveness and agreement with human-labelled data. Our findings reveal that while large language models significantly reduce manual effort and maintain substantial agreement with human annotators, full automation remains challenging due to the complexity of emotional interpretation. This work contributes to opinion mining by providing structured guidelines, an annotated dataset, and insights for developing automated pipelines to capture the complexity of emotions in app reviews.
* Accepted at the 33rd IEEE International Requirements Engineering 2025
conference
Via
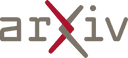
May 16, 2025
Abstract:While transformer-based models achieve strong performance on text classification, we explore whether masking input tokens can further enhance their effectiveness. We propose token masking regularization, a simple yet theoretically motivated method that randomly replaces input tokens with a special [MASK] token at probability p. This introduces stochastic perturbations during training, leading to implicit gradient averaging that encourages the model to capture deeper inter-token dependencies. Experiments on language identification and sentiment analysis -- across diverse models (mBERT, Qwen2.5-0.5B, TinyLlama-1.1B) -- show consistent improvements over standard regularization techniques. We identify task-specific optimal masking rates, with p = 0.1 as a strong general default. We attribute the gains to two key effects: (1) input perturbation reduces overfitting, and (2) gradient-level smoothing acts as implicit ensembling.
Via
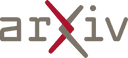