Topic:Skin Cancer Segmentation
What is Skin Cancer Segmentation? Skin cancer segmentation is the process of identifying and segmenting skin lesions in medical images for diagnosis and treatment planning.
Papers and Code
May 07, 2025
Abstract:Recent advancements in digital pathology have enabled comprehensive analysis of Whole-Slide Images (WSI) from tissue samples, leveraging high-resolution microscopy and computational capabilities. Despite this progress, there is a lack of labeled datasets and open source pipelines specifically tailored for analysis of skin tissue. Here we propose Histo-Miner, a deep learning-based pipeline for analysis of skin WSIs and generate two datasets with labeled nuclei and tumor regions. We develop our pipeline for the analysis of patient samples of cutaneous squamous cell carcinoma (cSCC), a frequent non-melanoma skin cancer. Utilizing the two datasets, comprising 47,392 annotated cell nuclei and 144 tumor-segmented WSIs respectively, both from cSCC patients, Histo-Miner employs convolutional neural networks and vision transformers for nucleus segmentation and classification as well as tumor region segmentation. Performance of trained models positively compares to state of the art with multi-class Panoptic Quality (mPQ) of 0.569 for nucleus segmentation, macro-averaged F1 of 0.832 for nucleus classification and mean Intersection over Union (mIoU) of 0.884 for tumor region segmentation. From these predictions we generate a compact feature vector summarizing tissue morphology and cellular interactions, which can be used for various downstream tasks. Here, we use Histo-Miner to predict cSCC patient response to immunotherapy based on pre-treatment WSIs from 45 patients. Histo-Miner identifies percentages of lymphocytes, the granulocyte to lymphocyte ratio in tumor vicinity and the distances between granulocytes and plasma cells in tumors as predictive features for therapy response. This highlights the applicability of Histo-Miner to clinically relevant scenarios, providing direct interpretation of the classification and insights into the underlying biology.
* 31 pages including supplement, 5 core figures, 5 supplement figures
Via
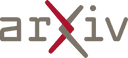
Apr 02, 2025
Abstract:Accurate segmentation of polyps and skin lesions is essential for diagnosing colorectal and skin cancers. While various segmentation methods for polyps and skin lesions using fully supervised deep learning techniques have been developed, the pixel-level annotation of medical images by doctors is both time-consuming and costly. Foundational vision models like the Segment Anything Model (SAM) have demonstrated superior performance; however, directly applying SAM to medical segmentation may not yield satisfactory results due to the lack of domain-specific medical knowledge. In this paper, we propose BiSeg-SAM, a SAM-guided weakly supervised prompting and boundary refinement network for the segmentation of polyps and skin lesions. Specifically, we fine-tune SAM combined with a CNN module to learn local features. We introduce a WeakBox with two functions: automatically generating box prompts for the SAM model and using our proposed Multi-choice Mask-to-Box (MM2B) transformation for rough mask-to-box conversion, addressing the mismatch between coarse labels and precise predictions. Additionally, we apply scale consistency (SC) loss for prediction scale alignment. Our DetailRefine module enhances boundary precision and segmentation accuracy by refining coarse predictions using a limited amount of ground truth labels. This comprehensive approach enables BiSeg-SAM to achieve excellent multi-task segmentation performance. Our method demonstrates significant superiority over state-of-the-art (SOTA) methods when tested on five polyp datasets and one skin cancer dataset.
* 2024 IEEE International Conference on Bioinformatics and Biomedicine
(BIBM)
Via
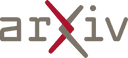
Apr 07, 2025
Abstract:Mohs micrographic surgery (MMS) is the gold standard technique for removing high risk nonmelanoma skin cancer however, intraoperative histopathological examination demands significant time, effort, and professionality. The objective of this study is to develop a deep learning model to detect basal cell carcinoma (BCC) and artifacts on Mohs slides. A total of 731 Mohs slides from 51 patients with BCCs were used in this study, with 91 containing tumor and 640 without tumor which was defined as non-tumor. The dataset was employed to train U-Net based models that segment tumor and non-tumor regions on the slides. The segmented patches were classified as tumor, or non-tumor to produce predictions for whole slide images (WSIs). For the segmentation phase, the deep learning model success was measured using a Dice score with 0.70 and 0.67 value, area under the curve (AUC) score with 0.98 and 0.96 for tumor and non-tumor, respectively. For the tumor classification, an AUC of 0.98 for patch-based detection, and AUC of 0.91 for slide-based detection was obtained on the test dataset. We present an AI system that can detect tumors and non-tumors in Mohs slides with high success. Deep learning can aid Mohs surgeons and dermatopathologists in making more accurate decisions.
* 14 pages, 2 figures
Via
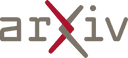
Mar 31, 2025
Abstract:Melanoma is the most lethal form of skin cancer, with an increasing incidence rate worldwide. Analyzing histological images of melanoma by localizing and classifying tissues and cell nuclei is considered the gold standard method for diagnosis and treatment options for patients. While many computerized approaches have been proposed for automatic analysis, most perform tissue-based analysis and nuclei (cell)-based analysis as separate tasks, which might be suboptimal. In this work, using the PUMA challenge dataset, we proposed a novel multi-stage deep learning approach by combining tissue and nuclei information in a unified framework based on the auto-context concept to perform segmentation and classification in histological images of melanoma. Through pre-training and further post-processing, our approach achieved second and first place rankings in the PUMA challenge, with average micro Dice tissue score and summed nuclei F1-score of 73.40% for Track 1 and 63.48% for Track 2, respectively. Our implementation for training and testing is available at: https://github.com/NimaTorbati/PumaSubmit
* 15 pages
Via
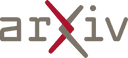
Feb 23, 2025
Abstract:We can achieve fast and consistent early skin cancer detection with recent developments in computer vision and deep learning techniques. However, the existing skin lesion segmentation and classification prediction models run independently, thus missing potential efficiencies from their integrated execution. To unify skin lesion analysis, our paper presents the Gaussian Splatting - Transformer UNet (GS-TransUNet), a novel approach that synergistically combines 2D Gaussian splatting with the Transformer UNet architecture for automated skin cancer diagnosis. Our unified deep learning model efficiently delivers dual-function skin lesion classification and segmentation for clinical diagnosis. Evaluated on ISIC-2017 and PH2 datasets, our network demonstrates superior performance compared to existing state-of-the-art models across multiple metrics through 5-fold cross-validation. Our findings illustrate significant advancements in the precision of segmentation and classification. This integration sets new benchmarks in the field and highlights the potential for further research into multi-task medical image analysis methodologies, promising enhancements in automated diagnostic systems.
* 12 pages, 7 figures, SPIE Medical Imaging 2025
Via
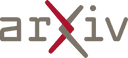
Dec 28, 2024
Abstract:Deep learning models (DLMs) frequently achieve accurate segmentation and classification of tumors from medical images. However, DLMs lacking feedback on their image segmentation mechanisms, such as Dice coefficients and confidence in their performance, face challenges when processing previously unseen images in real-world clinical settings. Uncertainty estimates to identify DLM predictions at the cellular or single-pixel level that require clinician review can enhance trust. However, their deployment requires significant computational resources. This study reports two DLMs, one trained from scratch and another based on transfer learning, with Monte Carlo dropout or Bayes-by-backprop uncertainty estimations to segment lesions from the publicly available The International Skin Imaging Collaboration-19 dermoscopy image database with cancerous lesions. A novel approach to compute pixel-by-pixel uncertainty estimations of DLM segmentation performance in multiple clinical regions from a single dermoscopy image with corresponding Dice scores is reported for the first time. Image-level uncertainty maps demonstrated correspondence between imperfect DLM segmentation and high uncertainty levels in specific skin tissue regions, with or without lesions. Four new linear regression models that can predict the Dice performance of DLM segmentation using constants and uncertainty measures, either individually or in combination from lesions, tissue structures, and non-tissue pixel regions critical for clinical diagnosis and prognostication in skin images (Spearman's correlation, p < 0.05), are reported for the first time for low-compute uncertainty estimation workflows.
* Presented at the 2024 IEEE International Conference on Machine
Learning and Applications (ICMLA), accepted for publication and in press by
IEEE
Via
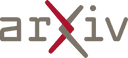
Jan 23, 2025
Abstract:Medical image analysis suffers from a lack of labeled data due to several challenges including patient privacy and lack of experts. Although some AI models only perform well with large amounts of data, we will move to data augmentation where there is a solution to improve the performance of our models and increase the dataset size through traditional or advanced techniques. In this paper, we evaluate the effectiveness of data augmentation techniques on two different medical image datasets. In the first step, we applied some transformation techniques to the skin cancer dataset containing benign and malignant classes. Then, we trained the convolutional neural network (CNN) on the dataset before and after augmentation, which significantly improved test accuracy from 90.74% to 96.88% and decreased test loss from 0.7921 to 0.1468 after augmentation. In the second step, we used the Mixup technique by mixing two random images and their corresponding masks using the retina and blood vessels dataset, then we trained the U-net model and obtained the Dice coefficient which increased from 0 before augmentation to 0.4163 after augmentation. The result shows the effect of using data augmentation to increase the dataset size on the classification and segmentation performance.
Via
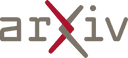
Jan 24, 2025
Abstract:Skin cancer is one of the most prevalent and potentially life-threatening diseases worldwide, necessitating early and accurate diagnosis to improve patient outcomes. Conventional diagnostic methods, reliant on clinical expertise and histopathological analysis, are often time-intensive, subjective, and prone to variability. To address these limitations, we propose a novel hybrid deep learning framework that integrates convolutional neural networks (CNNs) with Radial Basis Function (RBF) Networks to achieve high classification accuracy and enhanced interpretability. The motivation for incorporating RBF Networks lies in their intrinsic interpretability and localized response to input features, which make them well-suited for tasks requiring transparency and fine-grained decision-making. Unlike traditional deep learning models that rely on global feature representations, RBF Networks allow for mapping segments of images to chosen prototypes, exploiting salient features within a single image. This enables clinicians to trace predictions to specific, interpretable patterns. The framework incorporates segmentation-based feature extraction, active learning for prototype selection, and K-Medoids clustering to focus on these salient features. Evaluations on the ISIC 2016 and ISIC 2017 datasets demonstrate the model's effectiveness, achieving classification accuracies of 83.02\% and 72.15\% using ResNet50, respectively, and outperforming VGG16-based configurations. By generating interpretable explanations for predictions, the framework aligns with clinical workflows, bridging the gap between predictive performance and trustworthiness. This study highlights the potential of hybrid models to deliver actionable insights, advancing the development of reliable AI-assisted diagnostic tools for high-stakes medical applications.
* The paper has not been published by any journal/conference. It
contains 14 pages, with six figures and five tables to demonstrate results
Via
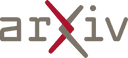
Dec 02, 2024
Abstract:Early detection of skin abnormalities plays a crucial role in diagnosing and treating skin cancer. Segmentation of affected skin regions using AI-powered devices is relatively common and supports the diagnostic process. However, achieving high performance remains a significant challenge due to the need for high-resolution images and the often unclear boundaries of individual lesions. At the same time, medical devices require segmentation models to have a small memory foot-print and low computational cost. Based on these requirements, we introduce a novel lightweight model called MambaU-Lite, which combines the strengths of Mamba and CNN architectures, featuring just over 400K parameters and a computational cost of more than 1G flops. To enhance both global context and local feature extraction, we propose the P-Mamba block, a novel component that incorporates VSS blocks along-side multiple pooling layers, enabling the model to effectively learn multiscale features and enhance segmentation performance. We evaluate the model's performance on two skin datasets, ISIC2018 and PH2, yielding promising results. Our source code will be made publicly available at: https://github.com/nqnguyen812/MambaU-Lite.
* 10 pages, 3 figures, 2 tables
Via
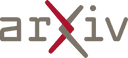
Nov 14, 2024
Abstract:Automatic melanoma segmentation is essential for early skin cancer detection, yet challenges arise from the heterogeneity of melanoma, as well as interfering factors like blurred boundaries, low contrast, and imaging artifacts. While numerous algorithms have been developed to address these issues, previous approaches have often overlooked the need to jointly capture spatial and sequential features within dermatological images. This limitation hampers segmentation accuracy, especially in cases with indistinct borders or structurally similar lesions. Additionally, previous models lacked both a global receptive field and high computational efficiency. In this work, we present the XLSTM-VMUNet Model, which jointly capture spatial and sequential features within derma-tological images successfully. XLSTM-VMUNet can not only specialize in extracting spatial features from images, focusing on the structural characteristics of skin lesions, but also enhance contextual understanding, allowing more effective handling of complex medical image structures. Experiment results on the ISIC2018 dataset demonstrate that XLSTM-VMUNet outperforms VMUNet by 1.25% on DSC and 2.07% on IoU, with faster convergence and consistently high segmentation perfor-mance. Our code of XLSTM-VMUNet is available at https://github.com/MrFang/xLSTM-VMUNet.
Via
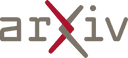